Designing Interpretable and Transparent Machine Learning Models for Early Detection of Breast Cancer
S Santra1 , A Sarkar2 , P Sahoo3 , T Ghosh4 , S Gautam5 , A Bhowmik6 , S Majumdar7 , A Bhowmik8 , S Bhowmik9 , P Sarkar10
Section:Research Paper, Product Type: Journal Paper
Volume-11 ,
Issue-01 , Page no. 316-322, Nov-2023
Online published on Nov 30, 2023
Copyright © S Santra, A Sarkar, P Sahoo, T Ghosh, S Gautam, A Bhowmik,S Majumdar,A Bhowmik, S Bhowmik, P Sarkar . This is an open access article distributed under the Creative Commons Attribution License, which permits unrestricted use, distribution, and reproduction in any medium, provided the original work is properly cited.
View this paper at Google Scholar | DPI Digital Library
How to Cite this Paper
- IEEE Citation
- MLA Citation
- APA Citation
- BibTex Citation
- RIS Citation
IEEE Style Citation: S Santra, A Sarkar, P Sahoo, T Ghosh, S Gautam, A Bhowmik,S Majumdar,A Bhowmik, S Bhowmik, P Sarkar, “Designing Interpretable and Transparent Machine Learning Models for Early Detection of Breast Cancer,” International Journal of Computer Sciences and Engineering, Vol.11, Issue.01, pp.316-322, 2023.
MLA Style Citation: S Santra, A Sarkar, P Sahoo, T Ghosh, S Gautam, A Bhowmik,S Majumdar,A Bhowmik, S Bhowmik, P Sarkar "Designing Interpretable and Transparent Machine Learning Models for Early Detection of Breast Cancer." International Journal of Computer Sciences and Engineering 11.01 (2023): 316-322.
APA Style Citation: S Santra, A Sarkar, P Sahoo, T Ghosh, S Gautam, A Bhowmik,S Majumdar,A Bhowmik, S Bhowmik, P Sarkar, (2023). Designing Interpretable and Transparent Machine Learning Models for Early Detection of Breast Cancer. International Journal of Computer Sciences and Engineering, 11(01), 316-322.
BibTex Style Citation:
@article{Santra_2023,
author = {S Santra, A Sarkar, P Sahoo, T Ghosh, S Gautam, A Bhowmik,S Majumdar,A Bhowmik, S Bhowmik, P Sarkar},
title = {Designing Interpretable and Transparent Machine Learning Models for Early Detection of Breast Cancer},
journal = {International Journal of Computer Sciences and Engineering},
issue_date = {11 2023},
volume = {11},
Issue = {01},
month = {11},
year = {2023},
issn = {2347-2693},
pages = {316-322},
url = {https://www.ijcseonline.org/full_spl_paper_view.php?paper_id=1451},
publisher = {IJCSE, Indore, INDIA},
}
RIS Style Citation:
TY - JOUR
UR - https://www.ijcseonline.org/full_spl_paper_view.php?paper_id=1451
TI - Designing Interpretable and Transparent Machine Learning Models for Early Detection of Breast Cancer
T2 - International Journal of Computer Sciences and Engineering
AU - S Santra, A Sarkar, P Sahoo, T Ghosh, S Gautam, A Bhowmik,S Majumdar,A Bhowmik, S Bhowmik, P Sarkar
PY - 2023
DA - 2023/11/30
PB - IJCSE, Indore, INDIA
SP - 316-322
IS - 01
VL - 11
SN - 2347-2693
ER -
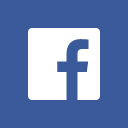
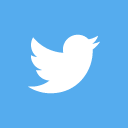
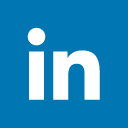
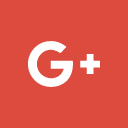
Abstract
Annually, over 1.15 million individuals are diagnosed with breast cancer across the globe. Today, only a select few accurate prognostic and predictive indicators are utilized in the clinical management of these patients. Early recognition of this deadly disease is pivotal, as it can reduce mortality rates while enhancing the life expectancy of those affected by breast cancer. Women are severely impacted by this condition, which has a high incidence and lethality rate. The absence of sturdy prognosis models complicates the medical professional`s ability to develop a treatment strategy that could extend the patient`s lifespan. Consequently, the need of the hour is to devise techniques that minimize error to boost accuracy. This study contrasts four algorithms, that predict the outcome of breast cancer, utilizing diverse datasets. All trials are performed within a simulated environment and facilitated on the JUPYTER platform. The research objective is divided into three sectors. The first sector entails predicting cancer prior to diagnosis, the second involves forecasting diagnosis and therapy, and the third centres on outcome during treatment. The proposed initiative can be employed to predict the outcomes of various methods, with the appropriate techniques chosen based on necessity. This investigation is undertaken to predict accuracy. Future studies can focus on predicting other distinct parameters, and breast cancer research can be classified based on these other parameters.
Key-Words / Index Term
Machine Learning, Identification of Breast Cancer, Attribute Isolation, PCA, LDA, Decision Tree
References
[1] The Massivebio, accessed 06 June 2022
[2] J. R. Quinlan, “Improved Use of Continuous Attributes in C4.5,” J. Artif. Intell. Res., vol. 4, pp. 77–
90, Mar. 1996.
[3] H. J. Hamilton, N. Shan, and N. Cercone, “RIAC: A Rule Induction Algorithm Based on Approximate Classiication,” 1996.
[4] S. Nayak and D. Gope, "Comparison of supervised learning algorithms for RF-based breast cancer detection," 2017 Computing and Electromagnetics International Workshop (CEM), Barcelona, 2017, pp.
[5] L Breiman, Machine Learning 45, 5-32 (2001).
[6] M. T. Ribeiro, S. Singh, and C. Guestrin, “Why should I trust you?: Explaining the predictions of any classifier,” in Proceedings of the 22nd ACM SIGKDD Int. Conf. on Knowledge Discovery and Data Mining, ACM, 2016, pp. 1135–1144.
[7] A. A. Freitas, “Comprehensible classification models: A position paper,” ACM SIGKDD explorations newsletter, vol. 15, no. 1, pp. 1–10, 2014.
[8] UCI Repository, Wisconsin Breast Cancer Database (WBCD), 2018