Comparative Study of Time Series Algorithms on Stock Price Forecasting
Mehul Kundu1 , Arpan Bose2 , Debasmita Guha3 , Romit Das4 , Aftab Alam5
Section:Research Paper, Product Type: Journal Paper
Volume-11 ,
Issue-01 , Page no. 12-28, Nov-2023
Online published on Nov 30, 2023
Copyright © Mehul Kundu, Arpan Bose, Debasmita Guha, Romit Das, Aftab Alam . This is an open access article distributed under the Creative Commons Attribution License, which permits unrestricted use, distribution, and reproduction in any medium, provided the original work is properly cited.
View this paper at Google Scholar | DPI Digital Library
How to Cite this Paper
- IEEE Citation
- MLA Citation
- APA Citation
- BibTex Citation
- RIS Citation
IEEE Citation
IEEE Style Citation: Mehul Kundu, Arpan Bose, Debasmita Guha, Romit Das, Aftab Alam, “Comparative Study of Time Series Algorithms on Stock Price Forecasting,” International Journal of Computer Sciences and Engineering, Vol.11, Issue.01, pp.12-28, 2023.
MLA Citation
MLA Style Citation: Mehul Kundu, Arpan Bose, Debasmita Guha, Romit Das, Aftab Alam "Comparative Study of Time Series Algorithms on Stock Price Forecasting." International Journal of Computer Sciences and Engineering 11.01 (2023): 12-28.
APA Citation
APA Style Citation: Mehul Kundu, Arpan Bose, Debasmita Guha, Romit Das, Aftab Alam, (2023). Comparative Study of Time Series Algorithms on Stock Price Forecasting. International Journal of Computer Sciences and Engineering, 11(01), 12-28.
BibTex Citation
BibTex Style Citation:
@article{Kundu_2023,
author = {Mehul Kundu, Arpan Bose, Debasmita Guha, Romit Das, Aftab Alam},
title = {Comparative Study of Time Series Algorithms on Stock Price Forecasting},
journal = {International Journal of Computer Sciences and Engineering},
issue_date = {11 2023},
volume = {11},
Issue = {01},
month = {11},
year = {2023},
issn = {2347-2693},
pages = {12-28},
url = {https://www.ijcseonline.org/full_spl_paper_view.php?paper_id=1408},
publisher = {IJCSE, Indore, INDIA},
}
RIS Citation
RIS Style Citation:
TY - JOUR
UR - https://www.ijcseonline.org/full_spl_paper_view.php?paper_id=1408
TI - Comparative Study of Time Series Algorithms on Stock Price Forecasting
T2 - International Journal of Computer Sciences and Engineering
AU - Mehul Kundu, Arpan Bose, Debasmita Guha, Romit Das, Aftab Alam
PY - 2023
DA - 2023/11/30
PB - IJCSE, Indore, INDIA
SP - 12-28
IS - 01
VL - 11
SN - 2347-2693
ER -
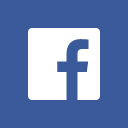
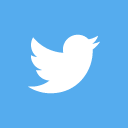
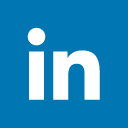
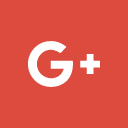
Abstract
Gauging the pulse of political climes and global tides alike, the stock exchange carries considerable consequence. Given the capricious manner by which the apparatus maneuvers, present technological means and trade tenets forbid accurately foretelling how planetary perturbations may sway share values over extended time. Of late, nonetheless, informatic techniques and artificially adroit algorithms have enabled scrutinizing episodes and occurrences that surface symbiotically, prognosticating the trajectory of equity prices for most, if not all, corporations in qualifying bourses. Past the fluctuating cultural zeitgeists and mercantile vicissitudes defining our epoch`s global political topography, prognosticating distant futures proves an elusive enterprise. Our objective henceforth shall be to prognosticate the quotidian inclination of equity premiums in the stock bazaar with the utmost precision achievable. The Stacking Ensemble model is a prevalent and trustworthy fashion to anticipate chronological successions. We shall explain why we opt for it over and above the other techniques adduced, akin to Meta Prophet, and the LSTM modus operandi, posterior to assaying. We manipulate a combination exemplar to ascertain the weighted norm of all in-sample data. In this exploration, we endeavored to disparate things with the stock valuations of multiple shareholding fully remunerated ordinary portions and thereafter effectuated predictions about stock valuations.
Key-Words / Index Term
Stock price forecast, ARIMA, SARIMA, LSTM, Meta Prophet, GRU, Kalman Filter, Ensemble
References
[1]. Zhang, G.P. "Time series forecasting using a hybrid ARIMA and neural network model" Neurocomputing, Vol.50, pp.159-175, 2003. http://doi.org/10.1016/S0925-2312(01)00702-0
[2]. Nikolas Topaloglou, Hercules Vladimirou, Stavros A. Zenios, "Integrated dynamic models for hedging international portfolio risks", European Journal of Operational Research, Vol.285, Issue.1, pp.48-65, 2019. http://doi.org/10.1016/j.ejor.2019.01.027
[3]. Taylor, Sean J. and Letham, Benjamin, “Forecasting at Scale“, The American Statistician, Vol.72, pp.37-45, 2018. http://doi.org/10.1080/00031305.2017.1380080
[4]. Chatfield, Chris, “The Analysis of Time Series: An Introduction”, Sixth Edition, Taylor & Francis, Chapman and Hall/CRC, United States of America, 2003, ISBN – 9781584883173
[5]. Xiao, Daiyou; Su, Jinxia, “Research on Stock Price Time Series Prediction Based on Deep Learning and Autoregressive Integrated Moving Average”, Scientific Programming, Vol.2022, 2022, Article ID 4758698, 12 pages, https://doi.org/10.1155/2022/4758698
[6]. Cai J, Zhang K, Jiang H, “Power Quality Disturbance Classification Based on Parallel Fusion of CNN and GRU”, Energies, Vol.16, Issue.10, pp.4029, 2023, https://doi.org/10.3390/en16104029
[7]. Wu, Hao; Zhou, Bing; Zhou, Haoru; Zhang, Pengyu; Wang, Meili, "Be-1DCNN: a neural network model for chromatin loop prediction based on bagging ensemble learning", Briefings in Functional Genomics, pp.2041-2657, 2023, https://doi.org/10.1093/bfgp/elad015
[8]. Padhi, D.K., Padhy, N., “Prognosticate of the financial market utilizing ensemble-based conglomerate model with technical indicators”, Evol. Intel, Vol.14, pp.1035–1051, 2021, https://doi.org/10.1007/s12065-020-00528-z
[9]. Nti, I.K., Adekoya, A.F. & Weyori, B.A, “A comprehensive evaluation of ensemble learning for stock-market prediction”, Journal of Big Data, Vol.7, Issue.20, 2020, https://doi.org/10.1186/s40537-020-00299-5
[10]. [10] F. A. Gers, J. Schmidhuber and F. Cummins, "Learning to Forget: Continual Prediction with LSTM," Neural Computation, Vol.12, Issue.10, pp.2451-2471, 2000. http:/doi.org/10.1162/089976600300015015