A Critical Analysis of Techniques Used For Learning Analytics Corresponding to Buiness
Kajal Devi1 , Harjinder Kaur2
Section:Review Paper, Product Type: Journal Paper
Volume-9 ,
Issue-9 , Page no. 39-44, Sep-2021
CrossRef-DOI: https://doi.org/10.26438/ijcse/v9i9.3944
Online published on Sep 30, 2021
Copyright © Kajal Devi, Harjinder Kaur . This is an open access article distributed under the Creative Commons Attribution License, which permits unrestricted use, distribution, and reproduction in any medium, provided the original work is properly cited.
View this paper at Google Scholar | DPI Digital Library
How to Cite this Paper
- IEEE Citation
- MLA Citation
- APA Citation
- BibTex Citation
- RIS Citation
IEEE Citation
IEEE Style Citation: Kajal Devi, Harjinder Kaur, “A Critical Analysis of Techniques Used For Learning Analytics Corresponding to Buiness,” International Journal of Computer Sciences and Engineering, Vol.9, Issue.9, pp.39-44, 2021.
MLA Citation
MLA Style Citation: Kajal Devi, Harjinder Kaur "A Critical Analysis of Techniques Used For Learning Analytics Corresponding to Buiness." International Journal of Computer Sciences and Engineering 9.9 (2021): 39-44.
APA Citation
APA Style Citation: Kajal Devi, Harjinder Kaur, (2021). A Critical Analysis of Techniques Used For Learning Analytics Corresponding to Buiness. International Journal of Computer Sciences and Engineering, 9(9), 39-44.
BibTex Citation
BibTex Style Citation:
@article{Devi_2021,
author = {Kajal Devi, Harjinder Kaur},
title = {A Critical Analysis of Techniques Used For Learning Analytics Corresponding to Buiness},
journal = {International Journal of Computer Sciences and Engineering},
issue_date = {9 2021},
volume = {9},
Issue = {9},
month = {9},
year = {2021},
issn = {2347-2693},
pages = {39-44},
url = {https://www.ijcseonline.org/full_paper_view.php?paper_id=5392},
doi = {https://doi.org/10.26438/ijcse/v9i9.3944}
publisher = {IJCSE, Indore, INDIA},
}
RIS Citation
RIS Style Citation:
TY - JOUR
DO = {https://doi.org/10.26438/ijcse/v9i9.3944}
UR - https://www.ijcseonline.org/full_paper_view.php?paper_id=5392
TI - A Critical Analysis of Techniques Used For Learning Analytics Corresponding to Buiness
T2 - International Journal of Computer Sciences and Engineering
AU - Kajal Devi, Harjinder Kaur
PY - 2021
DA - 2021/09/30
PB - IJCSE, Indore, INDIA
SP - 39-44
IS - 9
VL - 9
SN - 2347-2693
ER -
![]() |
![]() |
![]() |
386 | 494 downloads | 211 downloads |
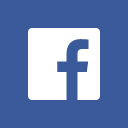
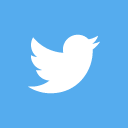
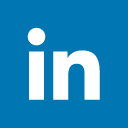
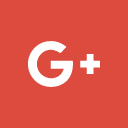
Abstract
Data Mining plays an important role in the Business world and it helps to the marketing institution to predict and make decisions related to the business’ academic status. Predicting business’ performance becomes more challenging due to the large volume of data in marketing databases. Currently in Malaysia, the lack of existing system to analyse and monitor the performance of the business is not being addressed. There are two main reasons of why this is happening. First, the study on existing prediction methods is still insufficient to identify the most suitable methods for predicting the performance of the business in Malaysian’s institutions. Second, Due to the lack of investigations on the factors affecting student’s achievements in particular courses within Malaysian context. Therefore, a systematically literature review on predicting student performance by the proposed system is a web based which makes use of the mining techniques for the extraction of useful information. This work is dig insight into state and event-based approaches for predicting student performance. Comparative analysis is conducted to suggest regression-based algorithms of state-based framework lack accuracy and correlation-based algorithms under event driven approach outperforms classical regression algorithms. It is also concluded from pedagogical point of view, higher engagement with social media leads to higher final grades
Key-Words / Index Term
Performance Prediction, Learning Analytics, Regression algorithm, correlation algorithms, social media
References
[1] Amra IAA (2017) Business performance prediction using KNN and Naïve Bayesian. Res Gate. doi: 10.1109/ICITECH.2017.8079967
[2] Bekele R, Menzel W (2017) A BAYESIAN APPROCH TO PREDICT PERFORMANCE OF STUDENT(BAPPS): A CASE WITH ETHIOPIAN BUSINESS. IJAER
[3] Carter BJ (2017) An ANOVA Analysis of Education Inequities Using Participation and Representation in Education Systems This is to certify that the doctoral dissertation by. IEEE Access
[4] Chen J, Feng J, Sun X, Wu N, Yang Z, Chen S (2019) MOOC Dropout Prediction Using a Hybrid Algorithm Based on Decision Tree and Extreme Learning Machine. IEEE Access 2019:
[5] Eashwar KB, Venkatesan R (2017) STUDENT PERFORMANCE PREDICTION USING SVM. IJMET 8:649–662
[6] Feng W, Tang J, Liu TX (2015) Understanding Dropouts in MOOCs. IEEE Access
[7] Gitinabard N, Khoshnevisan F, Lynch CF, Wang EY (2014) Your Actions or Your Associates?? Predicting Certification and Dropout in MOOCs with Behavioral and Social Features. IEEE Access
[8] Hoic-Bozic N, Holenko Dlab M, Mornar V (2015) Recommender System and Web 2.0 Tools to Enhance a Blended Learning Model. IEEE Trans Educ 1–1 . doi: 10.1109/TE.2015.2427116
[9] Ix DQG, Dojrulwkp J, Dpvd D, Kdnnhwkrwwdp QL (2016) student academic performance prediction model using decision tree and fuzzy genetic algorithm. Elsevier 25:326–332 . doi: 10.1016/j.protcy.2016.08.114
[10] Jasmer AM (2015) DISPOSITIONAL EMPLOYABILITY AND THE RELATIONSHIP TO CAREER SUCCESS?: A META-ANALYSIS. CSUSB Sch Work
[11] Kabra RR, Bichkar RS (2016) BUSINESS ’ PERFORMANCE PREDICTION USING GENETIC ABSTRACT?: IJCEA VI:19–29
[12] Kakkar H (2016) The Dispositional Antecedents of Promotive and Prohibitive Voice. J Appl Psychol 101:1342–1351
[13] Kaur A, Umesh N, Singh B (2018) Machine Learning Approach to Predict Student Academic Performance. IJRASET 6:734–742
[14] Khder M (2018) A Classification and Prediction Model for Student ’ s Performance in University A Classification and Prediction Model for Student ’ s Performance in University Level. Res Gate. doi: 10.3844/jcssp.2017.228.233
[15] Kloft M, Stiehler F, Zheng Z, Pinkwart N (2014) Predicting MOOC Dropout over Weeks Using Machine Learning Methods. IEEE Access 60–65
[16] Loh W-Y (2006) Logistic Regression Tree Analysis. Handb Eng Stat 537–549
[17] Lopes AP (2017) LEARNING MANAGEMENT SYSTEMS IN HIGHER EDUCATION. Res Gate
[18] Osmanbegovic, E., Suljic M (2012) Data mining approach for predicting student performance. J Econ Bus X:3–12
[19] Pinjuh A (2015) Learning Management system logs. Res Gate. doi: 10.1109/SOFTCOM.2015.7314114
[20] Popescu E, Leon F (2018) Predicting Academic Performance Based on Learner Traces in a Social Learning Environment. IEEE Access 6:72774–72785 . doi: 10.1109/ACCESS.2018.2882297
[21] Rosenfeld A, Sina S, Sarne D, Avidov O, Kraus S (2018) A Study of WhatsApp Usage Patterns and Prediction Models without Message Content. IEEE Access 1–24
[22] Thai-Nghe N, Drumond L, Krohn-Grimberghe A, Schmidt-Thieme L (2010) Recommender system for predicting student performance. Procedia Comput Sci 1:2811–2819 . doi: 10.1016/j.procs.2010.08.006
[23] Wang W, Graduate I (2015) Deep Model for Dropout Prediction in MOOCs. IEEE Access
[24] Zhang S, Yao L, Sun A, Tay YI (2018) Deep Learning based Recommender System?: A Survey and New Perspectives. CSIR 1:1–35