Process Improvement in the Criteria of Investment on Stock Market Using Data Mining Techniques
S.K. Sharma1
Section:Research Paper, Product Type: Journal Paper
Volume-9 ,
Issue-9 , Page no. 31-38, Sep-2021
CrossRef-DOI: https://doi.org/10.26438/ijcse/v9i9.3138
Online published on Sep 30, 2021
Copyright © S.K. Sharma . This is an open access article distributed under the Creative Commons Attribution License, which permits unrestricted use, distribution, and reproduction in any medium, provided the original work is properly cited.
View this paper at Google Scholar | DPI Digital Library
How to Cite this Paper
- IEEE Citation
- MLA Citation
- APA Citation
- BibTex Citation
- RIS Citation
IEEE Citation
IEEE Style Citation: S.K. Sharma, “Process Improvement in the Criteria of Investment on Stock Market Using Data Mining Techniques,” International Journal of Computer Sciences and Engineering, Vol.9, Issue.9, pp.31-38, 2021.
MLA Citation
MLA Style Citation: S.K. Sharma "Process Improvement in the Criteria of Investment on Stock Market Using Data Mining Techniques." International Journal of Computer Sciences and Engineering 9.9 (2021): 31-38.
APA Citation
APA Style Citation: S.K. Sharma, (2021). Process Improvement in the Criteria of Investment on Stock Market Using Data Mining Techniques. International Journal of Computer Sciences and Engineering, 9(9), 31-38.
BibTex Citation
BibTex Style Citation:
@article{Sharma_2021,
author = {S.K. Sharma},
title = {Process Improvement in the Criteria of Investment on Stock Market Using Data Mining Techniques},
journal = {International Journal of Computer Sciences and Engineering},
issue_date = {9 2021},
volume = {9},
Issue = {9},
month = {9},
year = {2021},
issn = {2347-2693},
pages = {31-38},
url = {https://www.ijcseonline.org/full_paper_view.php?paper_id=5391},
doi = {https://doi.org/10.26438/ijcse/v9i9.3138}
publisher = {IJCSE, Indore, INDIA},
}
RIS Citation
RIS Style Citation:
TY - JOUR
DO = {https://doi.org/10.26438/ijcse/v9i9.3138}
UR - https://www.ijcseonline.org/full_paper_view.php?paper_id=5391
TI - Process Improvement in the Criteria of Investment on Stock Market Using Data Mining Techniques
T2 - International Journal of Computer Sciences and Engineering
AU - S.K. Sharma
PY - 2021
DA - 2021/09/30
PB - IJCSE, Indore, INDIA
SP - 31-38
IS - 9
VL - 9
SN - 2347-2693
ER -
![]() |
![]() |
![]() |
428 | 423 downloads | 215 downloads |
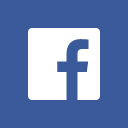
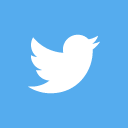
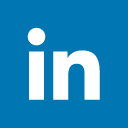
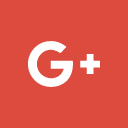
Abstract
Exact expectation of stock trade returns could be a difficult undertaking in view of unpredictable and non-direct nature of the monetary securities exchanges. The financial exchange information, as S&P500 Index is gigantic, perplexing, non-straight and noised. Foreseeing stock costs is a difficult undertaking as it relies upon different elements including however not restricted to worldwide economy, political conditions, organization`s monetary reports and execution and so on The speculation models utilizing this data have been a test. Along these lines, to augment the benefit and limit the misfortunes, procedures to anticipate estimations of the stock in advance by examining the pattern over the past couple of years, could end up being exceptionally valuable for making securities exchange developments [42,43]. This investigation proposes the accompanying momentary bit by bit technique: to consolidate two data sources that the financial backers can break down to settle on a choice. In the first place, the file information comprises the contribution for Profound Learning Neural Organization preparing, for addressing and estimating following day stock worth. Second, this exploration distinguishes the principal delegate endeavors, remembered for File, which address the List social inclination, utilizing Highlight Determination Investigation. At long last, the yields are supplemented and verified; the technique shows promising outcomes to upgrade the financial backer`s choice. Especially, for stock trade investigation, the data size is enormous and furthermore non-direct. To influence such an information proficient model is required which will recognize the secret examples and muddled relations during this huge informational index. AI strategies during this region have demonstrated to improve efficiencies by 60-86 percent when contrasted with the past techniques.
Key-Words / Index Term
Stock Exchange, Machine Learning, Predict, Feature Selection and Forecasting
References
[1] F. Mostafa, T. Dillon, and E. Chang, "Computational intelligence applications to option pricing, volatility forecasting and value at risk, Studies in Computational Intelligence," Springer International Publishing, vol. 697, 2017.
[2] Y. Fang, "Feature selection, deep neural network, and trend prediction," Journal of Shanghai Jiaotong University (Science), vol. 23, no. 2, pp. 297–307, 2018.
[3] X. Zheng and B. Chen, "Stock market modeling and forecasting," LNCIS, Vol. 442, pp. 1–11, London: Springer-Verlag, 2013.
[4] M. Olden, "Predicting stocks with machine learning," Master’s Thesis, 2016.
[5] X.-Y. Qian and S. Gao, "Financial series prediction: Comparison between precision of time series models and machine learning methods," Mathematics, Computer Science, Economics, 2017.
[6] S. Banik and A. Khan, "Forecasting US NASDAQ stock index values using hybrid forecasting systems," in Porc. 18th International Conference on Computer and Information Technology (ICCIT), 2015 [6].
[7] Z. Cao, L. Wang, and G. Melo, "Multiple-weight recurrent neural networks," in Proc. the Twenty-Sixth International Joint Conference on Artificial Intelligence (IJCAI-17), 2017.
[8] R. Singh and S. Srivastava, "Stock prediction using deep learning," Multimedia Tools Application, vol. 76, pp. 18569–18584, 2017.
[9] B. Yong, M. Rahim, and A. Abdullah, "A stock market trading system using deep neural network," AsiaSim 2017, Part I, Singapore, 2017.
[10] B. Yang, Z. Gong, and W. Yang, "Stock market index prediction using deep neural network ensemble," in Proc. the 36th Chinese Control Conference, Dalian, 2017.
[11] J. Ma, M. Yu, S. Fong, K. Ono, E. Sage, B. Demchak, R. Sharan, and T. Ideker, "Using deep learning to model the hierarchical structure and function of a cell," Nature Methods, pp. 1-12, 2018.
[12] P. Addo, D. Guegan and B. Hassani, "Credit risk analysis using machine and deep learning models," Documents de Travail du Centre d’Economie de la Sorbonne, 2018.
[13] T. Fischer and C. Krauss, "Deep learning with long short-term memory networks,” FAU Discussion Papers in Economics, 2017.
[14] H. Abdou, "Prediction of financial strength ratings using machine learning and conventional techniques," Investment Management and Financial Innovation, vol. 14, no. 4, pp. 194-211, 2017.
[15] S. Edet., Recurrent Neural Networks in Forecasting S&P 500 Index, 2017.
[16] V. Gavrishchaka, Z. Yang, R. Miao, and O. Senyukova, "Advantages of hybrid deep learning frameworks in applications with limited Data," International Journal of Machine Learning and Computing, vol. 8, no. 6, pp. 549-558, 2018.
[17] V. Sze, Y. Chen, T. Yang, and J. Emer, "Efficient Processing of Deep Neural Networks: A Tutorial and Survey," Proceedings of the IEEE, vol. 105, no. 12, pp. 2296-2329, 2017.
[18] T. Cook and A. Hall, Macroeconomic Indicator Forecasting with Deep Neural Networks, 2017.
[19] M. Abe and H. Nakayama, Deep Learning for Forecasting Stock Returns in the Cross-Section, 2017.
[20] A. Moghaddama, M. Moghaddamb, and M. Esfandyaric, "Stock market index prediction using artificial neural network," Journal of Economics, Finance and Administrative Science, vol. 21, pp. 89–93, 2016.
[21] I. Guyon and A. Elisseeff, "An introduction to variable and feature selection," Journal of Machine Learning Research, vol. 3, pp. 1157-1182, 2003.
[22] G. Chandrashekar and F. Sahin, "A survey on feature selection methods," Computers and Electrical Engineering, vol. 40, pp. 16–28, 2014.
[23] Y. Saeys, I. Inza, and P. Larrañaga, "A review of feature selection techniques in bioinformatics," Bioinformatics Review, vol. 23, no. 19, pp. 2507–2517, 2007.
[24] J. Miao and L. Niub, "A survey on feature selection," Information Technology and Quantitative Managemen, 2016.
[25] J. Dy and C. Brodley, "Feature selection for unsupervised learning," Journal of Machine Learning Research, vol. 5, pp. 845–889, 2004.
[26] M. Law, M. Figueiredo, and A. Jain, "Simultaneous feature selection and clustering using mixture models.," IEEE Transactions on Pattern Analysis and Machine Intelligence, vol. 26, no. 9, pp. 1154-1166, 2004.
[27] R. Kohavi and G. John, "Wrappers for feature subset selection," Artificial Intelligence, vol. 97, pp. 273-324, 1997.
[28] V. Bolón-Canedo, N. Sánchez-Maroño, and A. Alonso-Betanzos, Feature Selection for High-Dimensional Data, Switzerland: Springer International Publishing, 2015.
[29] H. Abusamra, "A comparative study of feature selection and classification methods for gene expression data of glioma," Procedia Computer Science, vol. 23, pp. 5-14, 2013.
[30] R. Kaur, M. Sachdeva, and G. Kumar, "Study and comparison of feature selection approaches for intrusion detection," International Journal of Computer Applications, 2016.
[31] E. Mahsereci, S. Ay?e, and T. ?brikçib, "A comparative study on the effect of feature selection on classification accuracy," Procedia Technology, vol. 1, pp. 323-327, 2012.
[32] W. Puch, E. Goodman, M. Pei, L. Chia-Shun, P. Hovland and R. Enbody, "Further research on feature selection and classification using genetic algorithm," in Porc. International Conference On Genetic Algorithm, 1993.
[33] B. Arguello, "A survey of feature selection methods: Algorithms and software," Austin, 2015.
[34] The University of Waikato, WEKA Manual for Version 3-7-8, Hamilton: New Zealand, 2013.
[35] I. Witten, F. Eibe and M. Hall, "Data Mining: Practical machine learning tools and techniques," The Morgan Kaufmann Series in Data Management Systems, 2011.
[36] E. Barnard and L.Wessels, "Extrapolation and interpolation in neural network classifiers," IEEE Control Systems Magazine, vol. 12, no. 5, pp. 50-53, 1992.
[37] P. Haley and D. Soloway, "Extrapolation limitations of multilayer feedforward neural networks," in Proc. International Joint Conference on Neural Networks, Baltimore, 1992.
[38] A. Pektas and H. Cigizoglu, "Investigating the extrapolation performance of neural network models in suspended sediment data," Hydrological Sciences Journal, vol. 62, no. 10, pp.1694-1703, 2017.
[39] P. Hettiarachchi, M. Hall and A. Minns, "The extrapolation of artificial neural networks for the modeling of rainfall-runoff relationships," Journal of Hydroinformatics, vol. 07, no. 4, pp. 291-296, 2005.
[40] MathWorks, Neural Network Toolbox™. User’s Guide. R2014a, The MathWorks, Inc., 2014.
[41] A. Prastyo, D. Junaedi, and M. Sulistiyo, "Stock price forecasting using artificial neural network," in Proc. Fifth International Conference on Information and Communication Technology (ICoICT), 2017.
[42] MH. Najeb, Masoud, “The impact of stock market performance upon economic growth.” International Journal of Economics and Financial Issues 3 (4) : 788–798, 2017.
[43] Murkute, Amod, and Tanuja Sarode, “Forecasting market price of stock using artificial neural network.” International Journal of Computer Applications 124 (12) : 11-15, 2015.
[44] C. Montenegro and M. Malina, “Improving the Criteria of the Investment on Stock Market Using Data Mining Techniques: The Case of S&P500 Index,” International Journal of Machine Learning and Computing, Vol. 10, No. 2, 2020.