Diabetes Risk Detection Review Using Machine Learning Techniques
Sanat Kumar Sahu1
Section:Review Paper, Product Type: Journal Paper
Volume-9 ,
Issue-8 , Page no. 84-86, Aug-2021
CrossRef-DOI: https://doi.org/10.26438/ijcse/v9i8.8486
Online published on Aug 31, 2021
Copyright © Sanat Kumar Sahu . This is an open access article distributed under the Creative Commons Attribution License, which permits unrestricted use, distribution, and reproduction in any medium, provided the original work is properly cited.
View this paper at Google Scholar | DPI Digital Library
How to Cite this Paper
- IEEE Citation
- MLA Citation
- APA Citation
- BibTex Citation
- RIS Citation
IEEE Citation
IEEE Style Citation: Sanat Kumar Sahu, “Diabetes Risk Detection Review Using Machine Learning Techniques,” International Journal of Computer Sciences and Engineering, Vol.9, Issue.8, pp.84-86, 2021.
MLA Citation
MLA Style Citation: Sanat Kumar Sahu "Diabetes Risk Detection Review Using Machine Learning Techniques." International Journal of Computer Sciences and Engineering 9.8 (2021): 84-86.
APA Citation
APA Style Citation: Sanat Kumar Sahu, (2021). Diabetes Risk Detection Review Using Machine Learning Techniques. International Journal of Computer Sciences and Engineering, 9(8), 84-86.
BibTex Citation
BibTex Style Citation:
@article{Sahu_2021,
author = {Sanat Kumar Sahu},
title = {Diabetes Risk Detection Review Using Machine Learning Techniques},
journal = {International Journal of Computer Sciences and Engineering},
issue_date = {8 2021},
volume = {9},
Issue = {8},
month = {8},
year = {2021},
issn = {2347-2693},
pages = {84-86},
url = {https://www.ijcseonline.org/full_paper_view.php?paper_id=5386},
doi = {https://doi.org/10.26438/ijcse/v9i8.8486}
publisher = {IJCSE, Indore, INDIA},
}
RIS Citation
RIS Style Citation:
TY - JOUR
DO = {https://doi.org/10.26438/ijcse/v9i8.8486}
UR - https://www.ijcseonline.org/full_paper_view.php?paper_id=5386
TI - Diabetes Risk Detection Review Using Machine Learning Techniques
T2 - International Journal of Computer Sciences and Engineering
AU - Sanat Kumar Sahu
PY - 2021
DA - 2021/08/31
PB - IJCSE, Indore, INDIA
SP - 84-86
IS - 8
VL - 9
SN - 2347-2693
ER -
![]() |
![]() |
![]() |
248 | 333 downloads | 182 downloads |
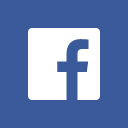
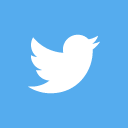
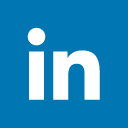
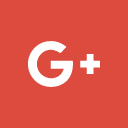
Abstract
Data Mining (DM) and Machine Learning (ML) are used as training algorithm for learning classification and feature selection technique (FST) from data. The DM and ML are contemporary concepts that are used to classify data with remarkable accuracy and efficiency. This paper contains a collection of research publications that utilized DM and ML techniques to diagnose diabetes. The survey`s objective was to determine the study objective, diabetic type, data sets and technologies employed, as well as the results.
Key-Words / Index Term
Classification, data mining, diabetic disease, feature selection technique, machine learning
References
[1] J. Han, M. Kamber, and J. Pei, Data mining: concepts and techniques, Third. Elsevier, 2012.
[2] A. M. Altamimi, “Performance Analysis of Supervised Classifying Algorithms to Predict Diabetes in Children,” J. Xi’an Univ. Archit. Technol., vol. XII, no. III, pp. 2010–2017, 2020.
[3] A. Kareem, L. Shi, L. Wei, and Y. Tao, “A Comparative Analysis and Risk Prediction of Diabetes at Early Stage using Machine Learning Approach A Comparative Analysis and Risk Prediction of Diabetes at Early Stage using Machine Learning Approach,” Int. J. Futur. Gener. Commun. Netw., vol. 13, no. 3, pp. 4151–4163, 2020.
[4] G. A. Pethunachiyar, “Classification of diabetes patients using kernel based support vector machines,” in 2020 International Conference on Computer Communication and Informatics, ICCCI 2020, 2020, pp. 22–25.
[5] H. Kaur and G. Kaur, “Prediction of Diabetes Using Support Vector Machine,” Int. J. Res. Eng. Appl. Manag., vol. 05, no. 02, pp. 470–473, 2019.
[6] J. Zhang et al., “Diagnostic Method of Diabetes Based on Support Vector Machine and Tongue Images,” Hindawi BioMed Res. Int. Res. Int., vol. 2017, 2017.
[7] S. Perveen, M. Shahbaz, A. Guergachi, and K. Keshavjee, “Performance Analysis of Data Mining Classification Techniques to Predict Diabetes,” in Procedia Computer Science, 2016, vol. 82, no. March, pp. 115–121.
[8] L. Han, S. Luo, J. Yu, L. Pan, and S. Chen, “Rule extraction from support vector machines using ensemble learning approach: An application for diagnosis of diabetes,” IEEE J. Biomed. Heal. Informatics, vol. 19, no. 2, pp. 728–734, 2015.
[9] O. S.Soliman and E. AboElhamd, “Classification of Diabetes Mellitus using Modified Particle Swarm Optimization and Least Squares Support Vector Machine,” Int. J. Comput. Trends Technol., vol. 8, no. 1, pp. 38–44, 2014.
[10] A. Kumari and R. Chitra, “Classification Of Diabetes Disease Using Support Vector Machine,” Int. J. Eng. Res. Appl., vol. 3, no. 2, pp. 1797–1801, 2013.
[11] M. S. Uzer, N. Yilmaz, and O. Inan, “Feature selection method based on artificial bee colony algorithm and support vector machines for medical datasets classification,” Sci. World J., vol. 2013, 2013.
[12] D. Giveki, H. Salimi, G. Bahmanyar, and Y. Khademian, “Automatic Detection of Diabetes Diagnosis using Feature Weighted Support Vector Machines based on Mutual Information and Modified Cuckoo Search,” cornell, 2012.
[13] M. Rambhajani, W. Deepanker, and N. Pathak, “A Survey on Implementation of Machine Learning Techniques for Dermatology Diseases Classification,” Int. J. Adv. Eng. Technol., vol. 8, no. 2, pp. 194–202, 2015.
[14] M. He, D. Jianan, and Z. Sinian, “Kaggle Competition?: Product Classification,” Kaggle Compet. Prod. Classif., 2015.
[15] M. Leshno, “Chapter 25 statistical methods for data mining,” no. Dm, 2005.