An Efficient Approach for Converting 2D Medical Images Into Non Immersive VR Model
M. Mohamed Sathik1 , A. Farzana2 , S. Shajun Nisha3
Section:Research Paper, Product Type: Journal Paper
Volume-9 ,
Issue-8 , Page no. 46-51, Aug-2021
CrossRef-DOI: https://doi.org/10.26438/ijcse/v9i8.4651
Online published on Aug 31, 2021
Copyright © M. Mohamed Sathik, A. Farzana, S. Shajun Nisha . This is an open access article distributed under the Creative Commons Attribution License, which permits unrestricted use, distribution, and reproduction in any medium, provided the original work is properly cited.
View this paper at Google Scholar | DPI Digital Library
How to Cite this Paper
- IEEE Citation
- MLA Citation
- APA Citation
- BibTex Citation
- RIS Citation
IEEE Style Citation: M. Mohamed Sathik, A. Farzana, S. Shajun Nisha, “An Efficient Approach for Converting 2D Medical Images Into Non Immersive VR Model,” International Journal of Computer Sciences and Engineering, Vol.9, Issue.8, pp.46-51, 2021.
MLA Style Citation: M. Mohamed Sathik, A. Farzana, S. Shajun Nisha "An Efficient Approach for Converting 2D Medical Images Into Non Immersive VR Model." International Journal of Computer Sciences and Engineering 9.8 (2021): 46-51.
APA Style Citation: M. Mohamed Sathik, A. Farzana, S. Shajun Nisha, (2021). An Efficient Approach for Converting 2D Medical Images Into Non Immersive VR Model. International Journal of Computer Sciences and Engineering, 9(8), 46-51.
BibTex Style Citation:
@article{Sathik_2021,
author = {M. Mohamed Sathik, A. Farzana, S. Shajun Nisha},
title = {An Efficient Approach for Converting 2D Medical Images Into Non Immersive VR Model},
journal = {International Journal of Computer Sciences and Engineering},
issue_date = {8 2021},
volume = {9},
Issue = {8},
month = {8},
year = {2021},
issn = {2347-2693},
pages = {46-51},
url = {https://www.ijcseonline.org/full_paper_view.php?paper_id=5377},
doi = {https://doi.org/10.26438/ijcse/v9i8.4651}
publisher = {IJCSE, Indore, INDIA},
}
RIS Style Citation:
TY - JOUR
DO = {https://doi.org/10.26438/ijcse/v9i8.4651}
UR - https://www.ijcseonline.org/full_paper_view.php?paper_id=5377
TI - An Efficient Approach for Converting 2D Medical Images Into Non Immersive VR Model
T2 - International Journal of Computer Sciences and Engineering
AU - M. Mohamed Sathik, A. Farzana, S. Shajun Nisha
PY - 2021
DA - 2021/08/31
PB - IJCSE, Indore, INDIA
SP - 46-51
IS - 8
VL - 9
SN - 2347-2693
ER -
![]() |
![]() |
![]() |
463 | 351 downloads | 209 downloads |
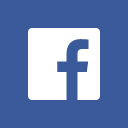
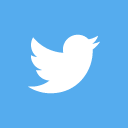
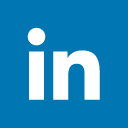
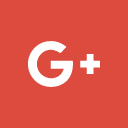
Abstract
The primary objective of this paper is to find out an efficient approach for converting 2D medical images into a desktop level VR model. The target is achieved in three stages: segmentation, 2D to 3D reconstruction, and 3D to VR modeling. Segmentation is the process of partitioning the digital image into sub parts or meaningful segments which help in segregating the cognitive information in the region of interest. Several segmentation algorithms are used to segment the input image. Best segmentation techniques are preferred for 3D reconstruction. Two types of 3d reconstruction techniques are used in formulating a 3D model. The AMILab 3.2.0 is used to provide non immersive visualization. Quantitative metrics such as Accuracy, Sensitivity, Specificity, Precision, F Score, Border Error, Jaccard Distance, Volumetric Overlap Error, Relative Volume Difference, Average Symmetric surface Distance and Maximum Symmetric surface Distance are used to evaluate the performance. Constructed VR model helps students in learning human anatomy efficiently.
Key-Words / Index Term
Medical Image, Segmentation, 3D Reconstruction, Non Immersive VR
References
[1]. A.HaseenaThasneem, R.Mehaboobathunnisa, M. Mohammed Sathik and Arumugam, ”Comparison Of Different Segmentation Algorithms For Dermoscopic Images” Ictact Journal On Image And Video Processing, Volume: 05, Issue: 04, May 2015.
[2]. Nida M. Zaitouna, Musbah J. Aqelb, “Survey on Image Segmentation Techniques” International Conference on Communication, Management and Information Technology (ICCMIT 2015), Procedia Computer Science volume 65, 797 – 806, 2015.
[3]. A. Farzana , Dr.M. Mohamed Sathik, “Review of Brain MRI Segmentation Techniques”, International Research Journal of Engineering and Technology (IRJET), Volume: 04 Issue: 04, Apr -2017.
[4]. C. Li, C. Xu, C. Gui and M. D. Fox, "Distance Regularized Level Set Evolution and Its Application to Image Segmentation," in IEEE Transactions on Image Processing, vol. 19, no. 12, pp. 3243-3254, Dec. 2010 .
[5]. T. F. Chan and L. A. Vese, "Active contours without edges," in IEEE Transactions on Image Processing, vol. 10, no. 2, pp. 266-277, Feb. 2001, doi: 10.1109/83.902291.
[6]. Y. Zhang, M. Brady, and S. Smith, “Segmentation of Brain MR Images Through a Hidden Markov Random Field Model and the Expectation-Maximization Algorithm”, IEEE Transactions on Medical Imaging, 20(1), 45-57, (2001).
[7]. Himani S. Bhatt and Sima K. Gonsai,” 3D Modelling and Volume Rotation of 2D Medical Images”, International Journal of Advanced Research in Electrical,Electronics and Instrumentation Engineering, Vol. 3, Issue 3, March 2014.
[8]. Chethan kumar, Anitha kumari R D.,”3D Reconstruction of Brain Tumor from 2D MRI’s using FCM and Marching cubes”, International Journal of Advanced Research in Electronics and Communication Engineering (IJARECE) Volume 3, Issue 9, September 2014
[9]. Sudharani. K., Rashmi. K., Sarma. T.C., K. Satya Prasad. T.C.,” 3D Multimodal MRI Brain Tumour Segmentation: A Volume Rendering Approach”, International Journal of Current Trends in Engineering & Research (IJCTER), Volume 2 Issue 6, pp. 290 – 294,June 2016.
[10]. Sindhushree. K. S., Manjula. T. R., Ramesha K.,” Detection And 3d Reconstruction Of Brain Tumor From Brain MRI Images”, International Journal of Engineering Research & Technology (IJERT), Vol. 2 Issue 8, ISSN: 2278-0181, August – 2013
[11]. Ananda Resmi1. S., Tessamma Thomas, “A semi-automatic method for segmentation and 3D modeling of glioma tumors from brain MRI”, J. Biomedical Science and Engineering, 378-383, July 2012.
[12].C.A. Cocosco, V. Kollokian, R.K.-S. Kwan, A.C. Evans : "BrainWeb: Online Interface to a 3D MRI Simulated Brain Database" NeuroImage, vol.5, no.4, part 2/4, S425, 1997 -- Proceedings of 3-rd International Conference on Functional Mapping of the Human Brain, Copenhagen, May 1997. http://www.bic.mni.mcgill.ca/brainweb/
[13]. A. Trujillo and K. Krissian, "AMILab: A 2D/3D Image Processing Software," in Proceedings Eighth IEEE International Conference on Computer Vision, Vancouver, BC, Canada, pp. 744, 2001. doi: 10.1109/ICCV.2001.937706
[14]. Krissian, Karl & Santana-Jorge, FJ & Santana-Cedrés, D. & Falcón-Torres, Carlos & Arencibia, Sara & Illera, Sara & Trujillo-Pino, Agustín & Chalopin, Claire & Alvarez, Luis, “AMILab software: medical image analysis, processing and visualization”, Studies in health technology and informatics. 173. 233-7, 2012.
[15]. A. Farzana, Dr. M. Mohamed Sathik, “Analysis of 2D to 3D Reconstruction Techniques over Brain MRI”, International Journal of Innovative Research in Computer and Communication Engineering, Vol. 5, Issue 5, May 2017