Identification of Brain Tumor Using Projection Pursuit Bivariate Multilayer Perceptred Classification
Renjeni P.S.1 , B. Mukunthan2
Section:Research Paper, Product Type: Journal Paper
Volume-9 ,
Issue-5 , Page no. 7-14, May-2021
CrossRef-DOI: https://doi.org/10.26438/ijcse/v9i5.714
Online published on May 31, 2021
Copyright © Renjeni P.S., B. Mukunthan . This is an open access article distributed under the Creative Commons Attribution License, which permits unrestricted use, distribution, and reproduction in any medium, provided the original work is properly cited.
View this paper at Google Scholar | DPI Digital Library
How to Cite this Paper
- IEEE Citation
- MLA Citation
- APA Citation
- BibTex Citation
- RIS Citation
IEEE Citation
IEEE Style Citation: Renjeni P.S., B. Mukunthan, “Identification of Brain Tumor Using Projection Pursuit Bivariate Multilayer Perceptred Classification,” International Journal of Computer Sciences and Engineering, Vol.9, Issue.5, pp.7-14, 2021.
MLA Citation
MLA Style Citation: Renjeni P.S., B. Mukunthan "Identification of Brain Tumor Using Projection Pursuit Bivariate Multilayer Perceptred Classification." International Journal of Computer Sciences and Engineering 9.5 (2021): 7-14.
APA Citation
APA Style Citation: Renjeni P.S., B. Mukunthan, (2021). Identification of Brain Tumor Using Projection Pursuit Bivariate Multilayer Perceptred Classification. International Journal of Computer Sciences and Engineering, 9(5), 7-14.
BibTex Citation
BibTex Style Citation:
@article{P.S._2021,
author = {Renjeni P.S., B. Mukunthan},
title = {Identification of Brain Tumor Using Projection Pursuit Bivariate Multilayer Perceptred Classification},
journal = {International Journal of Computer Sciences and Engineering},
issue_date = {5 2021},
volume = {9},
Issue = {5},
month = {5},
year = {2021},
issn = {2347-2693},
pages = {7-14},
url = {https://www.ijcseonline.org/full_paper_view.php?paper_id=5330},
doi = {https://doi.org/10.26438/ijcse/v9i5.714}
publisher = {IJCSE, Indore, INDIA},
}
RIS Citation
RIS Style Citation:
TY - JOUR
DO = {https://doi.org/10.26438/ijcse/v9i5.714}
UR - https://www.ijcseonline.org/full_paper_view.php?paper_id=5330
TI - Identification of Brain Tumor Using Projection Pursuit Bivariate Multilayer Perceptred Classification
T2 - International Journal of Computer Sciences and Engineering
AU - Renjeni P.S., B. Mukunthan
PY - 2021
DA - 2021/05/31
PB - IJCSE, Indore, INDIA
SP - 7-14
IS - 5
VL - 9
SN - 2347-2693
ER -
![]() |
![]() |
![]() |
401 | 487 downloads | 182 downloads |
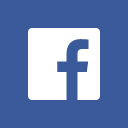
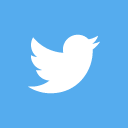
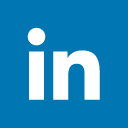
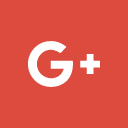
Abstract
The method of identifying the disease with person’s symptoms and signs is medical diagnosis. Brain tumour is the stimulating disorder that has to be identified at early stage for treatment. Many classification techniques have been introduced for performing brain tumour identification. However, the brain tumour identification accuracy level was not enhanced and time consumption was not lessened. In order to address these problems, Projection Pursuit Feature Selective Bivariate Multilayer Perceptred Classification (PPFSBMPC) Method is introduced. PPFSBMPC Method comprises two processes, namely feature selection and classification for brain tumour identification. To select the relevant features from the input database, Projection Pursuit Feature Selection process is carried out in PPFSBMPC Method. After performing the feature selection, Bivariate Multilayer Perceptred Classification process is accomplished for brain tumor identification. In addition, the classification process comprised multiple layers to categorize the input data as normal data or tumour diseased data. By this way, PPFSBMPC Method increases the brain tumor identification performance with higher accuracy and lesser time consumption. Experimental evaluation of PPFSBMPC Method is carried out with Epileptic Seizure Recognition Dataset on factors such as brain tumour identification accuracy, execution time, and error rate with respect to number of patient data. The experimental result demonstrates that the PPFSBMPC Method enhances the brain tumour identification accuracy and reduces the execution time when compared to state-of-the-art-works.
Key-Words / Index Term
Medical diagnosis, brain tumour, classification, feature selection, classification process, identification, seizure
References
[1] Varsha Harpale and Vinayak Bairagi, “An adaptive method for feature selection and extraction for classification of epileptic EEG signal in significant states”, Journal of King Saud University - Computer and Information Sciences, Elsevier, Pages 1-9 2018.
[2] Musa Peker, Baha Sen and Dursun Delen, “A Novel Method for Automated Diagnosis of Epilepsy Using Complex-Valued Classifiers”, IEEE Journal of Biomedical and Health Informatics, Volume 20, Issue 1, Pages 108-118,2016.
[3] Danda Shashank Reddy, Chinta Naga Harshitha and Carmel Mary Belinda, “Brain tumor prediction using naïve Bayes’ classifier and decision tree algorithms”, International Journal of Engineering &Technology, Volume 7, Pages 137-141,2018.
[4] ?ostas ?. Tsiouris , Vasileios C. Pezoulas , Michalis Zervakis, Spiros Konitsiotis, Dimitrios D. Koutsouris, Dimitrios I. Fotiadis, “A Long Short-Term Memory deep learning network for the prediction of epileptic seizures using EEG signals”, Computers in Biology and Medicine, Elsevier ,Volume 99, Pages 24-37, 2018.
[5] S Raghu, Natarajan Sriraam, Alangar Sathyaranjan Hegde, Pieter L Kubben, “A novel approach for classification of epileptic seizures using matrix determinant”, Expert Systems with Applications, Elsevier, Volume 127, Pages 323-341, 2019.
[6] Yuanfa Wang, Zunchao Li, Lichen Feng, Chuang Zheng, and Wenhao Zhang, “Automatic Detection of Epilepsy and Seizure using Multiclass Sparse Extreme Learning Machine Classification”, Computational and Mathematical Methods in Medicine, Hindawi Publishing Corporation, Volume 2017,Pages1-10, June 2017.
[7] Md. Kamrul Hasan, Md. Asif Ahamed, Mohiuddin Ahmad, and M. A. Rashid, “Prediction of Epileptic Seizure by Analysing Time Series EEG Signal Using k-NN Classifier”, Applied Bionics and Biomechanics, Hindawi, Volume 2017, Pages 1-12, August 2017.
[8] Md. Faizul Bari and Shaikh Anowarul Fattah, “Epileptic seizure detection in EEG signals using normalized IMFs in CEEMDAN domain and quadratic discriminant classifier”, Biomedical Signal Processing and Control, Elsevier, Volume 58, Pages 1-8, April 2020.
[9] Sandeep Kumar Satapathy, Satchidananda Dehuri, Alok Kumar Jagadev, “ABC optimized RBF network for classification of EEG signal for epileptic seizure identification”, Egyptian Informatics Journal, Elsevier, Volume 18, Issue 1, Pages 55-66,2017.
[10] Shamsul Huda, John Yearwood, Herbert F. Jelinek, Mohammad Mehedi Hassan, “A Hybrid Feature Selection with Ensemble Classification for Imbalanced Healthcare Data: A Case Study for Brain Tumor Diagnosis”, IEEE Access, Volume 4, Pages 9145- 9154, 2016.
[11] Lingraj Dora, Sanjay Agrawal, Rutuparna Pand and Ajith Abraham, “Optimal breast cancer classification using Gauss-Newton representation based algorithm”, Expert Systems with Applications, Elsevier, Volume 85, Pages 134-145, November 2017.
[12] Chih-Jen Tseng, Chi-Jie Lu, Chi-Chang Chang and Gin-Den Chen and Chalong Cheewakriangkrai, “Integration of data mining classification techniques and ensemble learning to identify risk factors and diagnose ovarian cancer recurrence”, Artificial Intelligence in Medicine, Elsevier, Volume 78, Pages 47-54, May 2017.
[13] Chu-Yu Chin, Sun-Yuan Hsieh and Vincent S. Tseng, “eDRAM: Effective early disease risk assessment with matrix factorization on a large-scale medical database: A case study on rheumatoid arthritis”, PLoS ONE, Volume 13, Issue 11, Pages 1-19. 2018.
[14] Varun Jain and Sunila Godara, “Comparative Study of Data Mining Classification Methods in Brain Tumour Disease Detection”, International Journal of Computer Science & Communication, Volume 8, Issue 2, Pages 12-17, March 2017.
[15] Abeg Kumar Jaiswal and Haider Banka, “Local pattern transformation based feature extraction techniques for classification of epileptic EEG signals”, Journal of Medical and Biological Engineering, Springer, Volume 38, Issue 2, Pages 222-235, April 2018.
[16] Mengni Zhou, Cheng Tian, Rui Cao, Bin Wang, Yan Niu, Ting Hu, Hao Guo and Jie Xiang, “Epileptic Seizure Detection Based on EEG Signals and CNN”, Frontiers in Neuroinformatics, Pages 1-15, December 2018.
[17] Diah P. Wulandari, Nomala G. P. Putriz, Yoyon K. Suprapto and Santi W. Purnami, Anda I. Juniani and Wardah R. Islamiyah, “Epileptic Seizure Detection Based on Bandwidth Features of EEG Signals”, Procedia Computer Science, Elsevier, Volume 161, Pages 568-576, 2019.
[18] Zeynab Mohammadpoory, Mahda Nasrolahzadeh and Javad Haddadnia, “Epileptic seizure detection in EEGs signals based on the weighted visibility graph entropy”, Seizure, Elsevier, Volume 50, Pages 202-208 August 2017.
[19] Anurag Nishad and Ram Bilas Pachori, “Classification of epileptic electroencephalogram signals using tunable-Q wavelet transform based filter-bank”, Journal of Ambient Intelligence and Humanized Computing, Springer, Pages 1-15, 2020.
[20] G. Ravi Shankar Reddy and Rameshwar Rao “Automated identification system for seizure EEG signals using tunable-Q wavelet transform”, Engineering Science and Technology, an International Journal, Elsevier .Volume 20, Issue 5, Pages 1486-1493, October 2017.