Multilingual-Word-Script Classification in Text Video Frames
Sunil C.1 , Chethan H.K.2 , Raghunandan K.S.3 , G. Hemantha Kumar4
Section:Research Paper, Product Type: Journal Paper
Volume-7 ,
Issue-12 , Page no. 87-92, Dec-2019
CrossRef-DOI: https://doi.org/10.26438/ijcse/v7i12.8792
Online published on Dec 31, 2019
Copyright © Sunil C., Chethan H.K., Raghunandan K.S., G. Hemantha Kumar . This is an open access article distributed under the Creative Commons Attribution License, which permits unrestricted use, distribution, and reproduction in any medium, provided the original work is properly cited.
View this paper at Google Scholar | DPI Digital Library
How to Cite this Paper
- IEEE Citation
- MLA Citation
- APA Citation
- BibTex Citation
- RIS Citation
IEEE Style Citation: Sunil C., Chethan H.K., Raghunandan K.S., G. Hemantha Kumar, “Multilingual-Word-Script Classification in Text Video Frames,” International Journal of Computer Sciences and Engineering, Vol.7, Issue.12, pp.87-92, 2019.
MLA Style Citation: Sunil C., Chethan H.K., Raghunandan K.S., G. Hemantha Kumar "Multilingual-Word-Script Classification in Text Video Frames." International Journal of Computer Sciences and Engineering 7.12 (2019): 87-92.
APA Style Citation: Sunil C., Chethan H.K., Raghunandan K.S., G. Hemantha Kumar, (2019). Multilingual-Word-Script Classification in Text Video Frames. International Journal of Computer Sciences and Engineering, 7(12), 87-92.
BibTex Style Citation:
@article{C._2019,
author = {Sunil C., Chethan H.K., Raghunandan K.S., G. Hemantha Kumar},
title = {Multilingual-Word-Script Classification in Text Video Frames},
journal = {International Journal of Computer Sciences and Engineering},
issue_date = {12 2019},
volume = {7},
Issue = {12},
month = {12},
year = {2019},
issn = {2347-2693},
pages = {87-92},
url = {https://www.ijcseonline.org/full_paper_view.php?paper_id=4979},
doi = {https://doi.org/10.26438/ijcse/v7i12.8792}
publisher = {IJCSE, Indore, INDIA},
}
RIS Style Citation:
TY - JOUR
DO = {https://doi.org/10.26438/ijcse/v7i12.8792}
UR - https://www.ijcseonline.org/full_paper_view.php?paper_id=4979
TI - Multilingual-Word-Script Classification in Text Video Frames
T2 - International Journal of Computer Sciences and Engineering
AU - Sunil C., Chethan H.K., Raghunandan K.S., G. Hemantha Kumar
PY - 2019
DA - 2019/12/31
PB - IJCSE, Indore, INDIA
SP - 87-92
IS - 12
VL - 7
SN - 2347-2693
ER -
![]() |
![]() |
![]() |
345 | 314 downloads | 164 downloads |
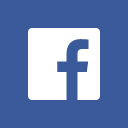
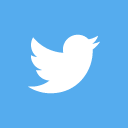
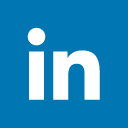
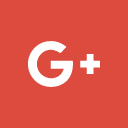
Abstract
Nowadays, achieving good results for the text classification of the multilingual scripts in arbitrary images in the videos is the most challenging task for the researchers. Most of the people depends on the internet and the digital world that makes difficult task to understand the multilingual script in various domain. Motivated from this, we proposed a text classification model for multilingual-word-scripts in video frames extracted from the videos which contains South Indian Multilingual Scripts namely, English, Tamil, Kannada, Malayalam and Telugu. Six-layer convolution neural network model has been used to classify the text to their respective classes. In this work we have castoff 600 word images from each script and total of 3000 word images that is extracted as the word images from the video frames for our experimentation. Our proposed model is proficient in accomplishing decent classification results when compared to existing conventional methods such as KNN and SVM classifier.
Key-Words / Index Term
Deep Neural Networks, Text Classification, Multilingual Scripts
References
[1] L Pang, S Zhu and C W Ngo., “Deep Multimodal Learning for Affective Analysis and Retrieval”, IEEE Transactions, Multimedia, Vol. 17, pp. 2008-2020, 2015.
[2] M M Rathore, A Paul, A Ahmad and S Rho., “Urban planning and Building Smart Cities based on Internet Things using Big Data Analytics”, Computer Networks, pp. 63-80, 2016.
[3] P B Pati and A G Ramakrishana., “OCR in Indian Scripts: A Survey”, Journal of IETE Technical Review, pp. 217-227, 2015.
[4] D Ghosh, T Dube and A P Shivaprasad., “Script Recognition –Review”, IEEE Transactions, pp. 2142-2161, PAMI 2010.
[5] T Young, D Hazarika and S Poria., “Recent Trends in Deep Learning based onNatural language processing”, IEEE Computational Intelligence Magazine, Vol 13, Issue 3, pp 55-75, 2018.
[6] A K Bhunia, A Konwer, A K Bhunia, A Bhowmick, P P Roy and U Pal., “Script Identification in natural scene image and video frames using attention based Convolutional-LSTM network”, Pattern Recognition, Elsevier, Vol 85, pp. 172-184, 2019.
[7] W Li, P Liu, Q Zhang and W Liu., “An Improved Approach for Text Sentiment Classification Based on Deep Neural Network via a Sentiment Attention Mechanism”, Journal of Future Internet, 11940, 2019.
[8] M Z Amin and N Nadeem., “Convolution Neural Network: Text Classification Model for Open Domain Question Answering System”, Computer Science, Information Retrieval, 2019.
[9] M Hughes, I Li, S Kotoulas and T Suzumura., “Medical Text Classification Using Convolution Neural Networks”, Studies in Health Technology and Informatics, Vol 235, pp. 246-250, 2017.
[10] A Hassan and A Mahmood., “Efficient Deep Learning Model for Text Classification based on Recurrent and Convolutional Layers”, 2017 16th IEEE International conference on Machine Learning and Applications (ICMLA), pp. 1108-1113, 2017.
[11] K S Raghunandan, P Shivakumara, G H Kumar, U Pal, and T Lu., “Sharpness and Contrast Features for Word-Wise Video Type Classification”, 2017 4trh IAPR Asian Conference on Pattern Recognition (ACPR) pp. 103-108, 2017.
[12] J Mei, L Dai, B Shi and X Bai., “Scene Text Script Identification with Convolutional Recurrent Neural Networks”, 2016 23rd International Conference on Pattern Recognition (ICPR), pp. 4053-4058, 2016.
[13] S Roy, P Shivakumara, U Pal, T Lu and C L Tan., “New tampered features for Scene and Caption text Classification in Video Frames”,2016 15th International Conference of Frontiers in Handwriting Recognition (ICFHR), pp. 36-41, 2016.
[14] N Sharma, P Shivakumara, U Pal, M Blumenstein and C L tan., “Piece-wise Linearity based Method for Text Frame Classification in Video”, Pattern Recognition, Elsevier, Vol 48, pp. 862-881, 2015.
[15] P P Yeotikar and P R Deshmukh., “Script Identification of Text Words from Multilingual Document”, International Journal of Computer Applications, pp. 22-29, X-PLORE 2013.
[16] D Duong, T Ba Dinh, T Dinh and D Duc., “Sports Video Classification using Bag of Words Model”, Intelligent Information and database Systems, ACIIDS, Springer, Vol. 7198, pp. 316-325,2012.
[17] S Haboubi, S Maddouri and H Amiri., “Word Classification in Bilingual Printed Documents”, 2012 6th International Conference on Sciences of Electronics, Technologies of Information and Telecommunications (SETIT), pp. 502-506, 2012.
[18] P Shivakumara, A Dutta, T Q Phan, C L Tan and U Pal., “A Novel Mutual Nearest Neighbor based Symmetry for Text Frame Classification”, Pattern Recognition, Elsevier, Vol 44, Issue 8, pp. 1671-1683, 2011.
[19] S Chanda, S Pal, K Frankle and U Pal., “Two-stage Approach for word-wise script Identification”, 2009 10th International Conference on Document Analysis and Recognition, pp. 926-930, 2009.
[20] W Zhang, T Yoshida and X Tang., “Text Classification based on multi-word with Support Vector Machine ”, Knowledge Based Systems, Elsevier, Vol 21, Issue 8, pp. 879-886, 2008.
[21] P B Pati and A G Ramakrishana., “Word Level Muli-Script Identification”, Pttern Recognition Letters, Vol 29, Issue 9, pp. 1218-1229, 2008.
[22] S Jaeger, H Ma and D Doermann., “ Identifying Script on Word-Level with Informational Confidence”, Eighth International Conference on Document Analysis and Recognition (ICDAR’05), Vol 1, pp. 416-420, 2005.
[23] A S Banu, P Vasuki, S M M Roomi and A Y Khan., “Sar Image Classification by Wavelet Transform and Euclidean Distance with Shanon Index Measurment”, International Journal of Scientific Research in Network Security and Communictions (IJSRNSC), Vol 6, Issue 3, pp 13-17, 2018.
[24] N S Lele., “Image Classification using Convolution Neural Network”, International Journal of Scientific Research inComputer Science and Engineering (IJSRCSE), Vol 6, Issue 3, pp 22-26, 2018.
[25] M S Hossain, M Al-Hammadi and G Muhammad., “Automatic fruit Classification using Deep Learning for Industrial applications” In IEEE Transactions on Industrial Informatics, 2015, pp.1027-1034.
[26] G E Dahl, T N Sainath and G E Hinton., ”Improving Deep Neural Networks for LVSCR using Rectified Linear Units and Dropout”, 2013 IEEE International Conference on Acoustics, Speech and Signal Processing, 2013, pp. 8609-8613.