Analyzing and Predicting Students Flow Visualization
N.S. Hima Bindu1 , R. Swathi2 , K. Sreedivya3
Section:Survey Paper, Product Type: Journal Paper
Volume-7 ,
Issue-11 , Page no. 145-147, Nov-2019
CrossRef-DOI: https://doi.org/10.26438/ijcse/v7i11.145147
Online published on Nov 30, 2019
Copyright © N.S. Hima Bindu, R. Swathi, K. Sreedivya . This is an open access article distributed under the Creative Commons Attribution License, which permits unrestricted use, distribution, and reproduction in any medium, provided the original work is properly cited.
View this paper at Google Scholar | DPI Digital Library
How to Cite this Paper
- IEEE Citation
- MLA Citation
- APA Citation
- BibTex Citation
- RIS Citation
IEEE Style Citation: N.S. Hima Bindu, R. Swathi, K. Sreedivya, “Analyzing and Predicting Students Flow Visualization,” International Journal of Computer Sciences and Engineering, Vol.7, Issue.11, pp.145-147, 2019.
MLA Style Citation: N.S. Hima Bindu, R. Swathi, K. Sreedivya "Analyzing and Predicting Students Flow Visualization." International Journal of Computer Sciences and Engineering 7.11 (2019): 145-147.
APA Style Citation: N.S. Hima Bindu, R. Swathi, K. Sreedivya, (2019). Analyzing and Predicting Students Flow Visualization. International Journal of Computer Sciences and Engineering, 7(11), 145-147.
BibTex Style Citation:
@article{Bindu_2019,
author = {N.S. Hima Bindu, R. Swathi, K. Sreedivya},
title = {Analyzing and Predicting Students Flow Visualization},
journal = {International Journal of Computer Sciences and Engineering},
issue_date = {11 2019},
volume = {7},
Issue = {11},
month = {11},
year = {2019},
issn = {2347-2693},
pages = {145-147},
url = {https://www.ijcseonline.org/full_paper_view.php?paper_id=4958},
doi = {https://doi.org/10.26438/ijcse/v7i11.145147}
publisher = {IJCSE, Indore, INDIA},
}
RIS Style Citation:
TY - JOUR
DO = {https://doi.org/10.26438/ijcse/v7i11.145147}
UR - https://www.ijcseonline.org/full_paper_view.php?paper_id=4958
TI - Analyzing and Predicting Students Flow Visualization
T2 - International Journal of Computer Sciences and Engineering
AU - N.S. Hima Bindu, R. Swathi, K. Sreedivya
PY - 2019
DA - 2019/11/30
PB - IJCSE, Indore, INDIA
SP - 145-147
IS - 11
VL - 7
SN - 2347-2693
ER -
![]() |
![]() |
![]() |
434 | 336 downloads | 224 downloads |
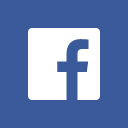
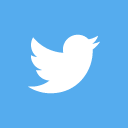
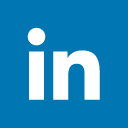
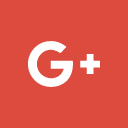
Abstract
In this work, I have a tendency to gift information science system to model and visualize student flow patterns supported electronic student data of a university. The datasets utilized by eCamp were antecedently disconnected and solely maintained and accessed in a much siloed manner by freelance field offices. At a campus-level, our models and image show however students create selections among many potential majors, as students step by step progress towards their sophomore, junior, and senior year. At a department-level, the scholar flow patterns unconcealed by eCamp show however every course plays a special role inside a syllabus. ECamp more dives all the way down to the roughness of the precise categories offered in every semester. At that level, eCamp shows however students navigate from one set of categories in one semester to a different set in a very enchant semester. I’d wish to build a deeper set of analytics mistreatment a lot of discourse info with further information sources like pedagogue info of every category, student help info, and student admission info. Previously, comprehensive info regarding student progression patterns in the slightest degree of those levels was merely unavailable. to it finish, we have a tendency to additionally demonstrate however insights into such student flow patterns will support analytical tasks involving student outcomes, student retention, and syllabus style.
Key-Words / Index Term
Big Data Applications, Data Analysis, Data Visualization
References
[1] G. Gee et al., “An open letter to college and university leaders: College completion must be our priority.” A report by National Commission on Higher Education Attainment. American Council on Education, 2011.
[2] L. Soares, “Post-traditional learners and the transformation of postsecondary education: A manifesto for college leaders,” American Council on Education, vol. 118, 2013.
[3] W. S. Swail, “A different viewpoint on student retention,” Higher Learning Research Communications, vol. 4, no. 2, 2014.
[4] V. Tinto, “Promoting student completion one class at a time,” in Symp. at the Retention 360 Conference, 2010.
[5] M. Raji, J. Duggan, B. DeCotes, J. Huang, and B. V. Zanden, “Visual progression analysis of student records data,” in Proc. of Workshop on Visualization in Data Science (VDS), Oct 2017.
[6] P. Attewell, S. Heil, and L. Reisel, “Competing explanations of undergraduate noncompletion,” American Educational Research Journal, vol. 48, no. 3, pp. 536–559, 2011.
[7] M. R. Clark, “Negotiating the freshman year: Challenges and strategies among first-year college students,” Journal of College Student Development, vol. 46, no. 3, pp. 296–316, 2005.
[8] J. Grann and D. Bushway, “Competency map: visualizing student learning to promote student success,” in Proc. of the 4th Intl Conference on Learning Analytics and Knowledge. ACM, 2014, pp. 168–172.
[9] G. D. Kuh, J. Kinzie, J. H. Schuh, and E. J. Whitt, Student success in college: Creating conditions that matter. John Wiley & Sons, 2011.
[10] M. J. Lutz, J. R. Vallino, K. Martinez, and D. E. Krutz, “Instilling a software engineering mindset through freshman seminar,” in Frontiers in Education Conf. (FIE), 2012, 2012.
[11] R. Mazza and V. Dimitrova, “Generation of graphical representations of student tracking data in course management systems,” in Proc. of the 9th Information Visualisation Conf., 2005.
[12] “Visualising student tracking data to support instructors in web-based distance education,” in Proc. of the 13th Intl World Wide Web Conf. on Alternate Track Papers & Posters, 2004.
[13] U. Rueda, M. Larranaga, M. Kerejeta, J. A. Elorriaga, and A. Arru- ˜ arte, “Visualizing student data in a real teaching context by means of concept maps,” in International Conf. on Knowledge Management I-Know, vol. 5, 2005.
[14] H. Siirtola, K.-J. Raiha, and V. Surakka, “Interactive curriculum visualization,” in 17th International Conference Information Visualisation (IV). IEEE, 2013, pp. 108–117.
[15] S. Gama and D. Goncalves, “Visualizing large quantities of educational datamining information,” in 18th International Conference on Information Visualisation (IV). IEEE, 2014, pp. 102–107.
[16] D. Wortman and P. Rheingans, “Visualizing trends in student performance across computer science courses,” ACM SIGCSE Bulletin, vol. 39, no. 1, pp. 430–434, 2007.
[17] B. Johnson and B. Shneiderman, “Tree-maps: a space-filling approach to the visualization of hierarchical information structures,” in Proc. of IEEE Visualization, Oct 1991, pp. 284–291.
[18] D. H. Huson, D. C. Richter, C. Rausch, T. Dezulian, M. Franz, and R. Rupp, “Dendroscope: An interactive viewer for large phylogenetic trees,” BMC bioinformatics, vol. 8, no. 1, p. 460, 2007.
[19] H. Zhang, S. Gao, M. J. Lercher, S. Hu, and W.-H. Chen, “Evolview, an online tool for visualizing, annotating and managing phylogenetic trees,” Nucleic acids research, vol. 40, no. W1, pp. W569–W572, 2012.
[20] Y. Zhu, L. Sun, A. Garbarino, C. Schmidt, J. Fang, and J. Chen, “Pathrings: a web-based tool for exploration of ortholog and expression data in biological pathways,” BMC bioinformatics, vol. 16, no. 1, p. 165, 2015.