Machine Learning Architecture to Financial Service Organizations
K. Palanivel1
Section:Research Paper, Product Type: Journal Paper
Volume-7 ,
Issue-11 , Page no. 85-104, Nov-2019
CrossRef-DOI: https://doi.org/10.26438/ijcse/v7i11.85104
Online published on Nov 30, 2019
Copyright © K. Palanivel . This is an open access article distributed under the Creative Commons Attribution License, which permits unrestricted use, distribution, and reproduction in any medium, provided the original work is properly cited.
View this paper at Google Scholar | DPI Digital Library
How to Cite this Paper
- IEEE Citation
- MLA Citation
- APA Citation
- BibTex Citation
- RIS Citation
IEEE Style Citation: K. Palanivel, “Machine Learning Architecture to Financial Service Organizations,” International Journal of Computer Sciences and Engineering, Vol.7, Issue.11, pp.85-104, 2019.
MLA Style Citation: K. Palanivel "Machine Learning Architecture to Financial Service Organizations." International Journal of Computer Sciences and Engineering 7.11 (2019): 85-104.
APA Style Citation: K. Palanivel, (2019). Machine Learning Architecture to Financial Service Organizations. International Journal of Computer Sciences and Engineering, 7(11), 85-104.
BibTex Style Citation:
@article{Palanivel_2019,
author = {K. Palanivel},
title = {Machine Learning Architecture to Financial Service Organizations},
journal = {International Journal of Computer Sciences and Engineering},
issue_date = {11 2019},
volume = {7},
Issue = {11},
month = {11},
year = {2019},
issn = {2347-2693},
pages = {85-104},
url = {https://www.ijcseonline.org/full_paper_view.php?paper_id=4950},
doi = {https://doi.org/10.26438/ijcse/v7i11.85104}
publisher = {IJCSE, Indore, INDIA},
}
RIS Style Citation:
TY - JOUR
DO = {https://doi.org/10.26438/ijcse/v7i11.85104}
UR - https://www.ijcseonline.org/full_paper_view.php?paper_id=4950
TI - Machine Learning Architecture to Financial Service Organizations
T2 - International Journal of Computer Sciences and Engineering
AU - K. Palanivel
PY - 2019
DA - 2019/11/30
PB - IJCSE, Indore, INDIA
SP - 85-104
IS - 11
VL - 7
SN - 2347-2693
ER -
![]() |
![]() |
![]() |
659 | 322 downloads | 240 downloads |
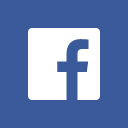
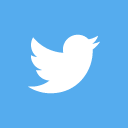
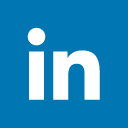
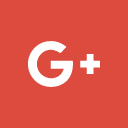
Abstract
Financial Services is a heavily regulated industry and organizational complexity that is driven by business segments, product lines, customer segments, a multitude of channels and transaction volumes. The role of data onto the financial services institutes has grown exponentially in recent years and is advancing rapidly. Traditional data solutions were built based on the demands of earlier days using technologies available at that point in time. However, the ever-growing amount of data and the insights that can now be extracted from it have rendered these solutions obsolete. A modern technology and advanced analytical solutions can only handle current demands and achieve business goals. Todays, Machine Learning (ML) gains traction in digital businesses and embraces it as a tool for creating operational efficiencies. The ML algorithm can analyze thousands of data sources simultaneously, something that human traders cannot possibly achieve. They help human traders squeeze a slim advantage over the market average. In addition, it has given the vast volumes of trading operations that small advantage often translate into significant profits. Robust architecture designs is one of the common traits of a successful enterprise financial ecosystem. This article discusses the use cases, benefits and pitfalls and the requirements of ML architecture to financial services institutes. This proposed ML architecture provides a fully functional technical picture for developing a cohesive business solution.
Key-Words / Index Term
Advanced Analytics, Machine Learning, Machine Learning Model, Machine Learning Architecture, Financial Service Institutes, Digital Business
References
[1] Addepto, Data Science in Finance – Why it is Beneficial to Use it, 2019.
[2] Akli Adjaoute. The AI Disconnect in the Financial Services Industry, Few industries are leveraging AI to the full extent of the technology’s power, 2019.
[3] Anastasia D, Seven Exciting Uses of ML in FinTech, 2018.
[4] Why you need a Digital Data Architecture to build a Sustainable Digital Business, 2017.
[5] C. Belém, L. Santos, and A.Leitão, “On the Impact of Machine Learning. Architecture without Architects?” in CAAD Futures, Seoul, South Korea., 2019.
[6] Carlton E. Sapp, Preparing and Architecting for ML, 2017.
[7] Daniel Faggella, Machine Learning in Finance – Present and Future Applications, 2019.
[8] Daniela Ventura, Diego Casado-Mansilla, Juan López-de-Armentia, Pablo Garaizar, Diego López-de-Ipiña, Vincenzo Catania, ARIIMA: A Real IoT Implementation of a ML Architecture for Reducing Energy Consumption, Springer International Publishing, 2014.
[9] DataStax Enterprise Reference Architecture, www.datastax.com.
[10] Elena Moldavskaya. Top Five Machine Learning Use Cases for the Financial Industry, Intetics Inc., 2018
[11] Elma, Machine learning in European financial institutions Study, 2018.
[12] Håkon Hapnes Strand. A Lightweight Machine Learning Architecture for IoT Streams, 2019.
[13] Introduction to Machine Learning Architecture, 2019.
[14] Joseph E. Beck, Beverly Park Woolf, Carole R. BealADVISOR: A machine learning architecture for intelligent tutor construction, American Association for Artificial Intelligence, 2000.
[15] Justin Boyan, Dayne Freitag, Thorsten Joachims, A Machine Learning Architecture for Optimizing Web Search Engines, In AAAI Workshop on Internet-based Information Systems, 1996.
[16] Karsten Egetoft, Data-Driven Analytics: Practical Use Cases for Financial Services, 2019.
[17] LB Shyamasundar, P Jhansi Rani. A Multiple-Layer Machine Learning Architecture for Improved Accuracy in Sentiment Analysis, Computational Intelligence, Machine Learning and Data Analytics, the Computer Journal, 2019.
[18] Machine Learning: How to Build Scalable Machine Learning Models, 2019.
[19] Mark Labbe, AI in Financial Services Helps Speed Consumer Interaction, 2019.
[20] Nikhil Gokhale, Ankur Gajjaria, Rob Kaye, Dave Kuder, AI Leaders in Financial Services Common Traits of Frontrunners in the Artificial Intelligence Race, 2018.
[21] Sidney D`Mello, Stan Franklin, Uma Ramamurthy, Bernard Baars. A Cognitive Science-Based Machine Learning Architecture, American Association for Artificial Intelligence, 2006.
[22] Tanmoy Ray, Scopes of ML and AI in Banking & Financial Services, ML & AI, The Future of Fintechs, 2017.
[23] Techwave, Machine Learning Use Cases in Finance, 2018.
[24] Thatchanamoorthy Lakshmanan, Anti-Money Laundering Powered by RegTech, 2017.
[25] William Markito. An Open Source Reference Architecture for Real-Time Stock Prediction. 2015.
[26] Palanivel K, Chithralekha T. “Big Data Reference Architecture for e-Learning Analytical Systems”, International Journal on Recent and Innovation Trends in Computing and Communication, Vol. 6, Issue. 1, pp.55-67, 2018.
[27] Palanivel, K. “Modern Network Analytics Architecture Stack to Enterprise Networks”, International Journal for Research in Applied Science & Engineering Technology (IJRASET), Vol.7, Issue.4, pp.263-280, Apr 2019.
[28] Kurt Stockinger, Nils Bundi, Jonas Heitz and Wolfgang Breymann. “Scalable architecture for Big Data financialanalytics: user‑defined functions vs. SQL”, Journal of Big Data, Vol.6, Issue.46, 2019.
[29] Andersen, T.G., Bollerslev, T., Frederiksen, P.H., and Nielsen, M.Ø., Continuous-time models, realized volatilities, and testable distributional implications for daily stock returns. Working paper, Northwestern University, 2006
[30] Steinfeld, K. Dreams May Come. In Acadia 2017, 590–599, 2017