Performance Evaluation of Machine Learning Classifiers for Epileptic Seizure Detection
Mirwais Farahi1 , Doreswamy 2
Section:Research Paper, Product Type: Journal Paper
Volume-7 ,
Issue-8 , Page no. 122-129, Aug-2019
CrossRef-DOI: https://doi.org/10.26438/ijcse/v7i8.122129
Online published on Aug 31, 2019
Copyright © Mirwais Farahi, Doreswamy . This is an open access article distributed under the Creative Commons Attribution License, which permits unrestricted use, distribution, and reproduction in any medium, provided the original work is properly cited.
View this paper at Google Scholar | DPI Digital Library
How to Cite this Paper
- IEEE Citation
- MLA Citation
- APA Citation
- BibTex Citation
- RIS Citation
IEEE Citation
IEEE Style Citation: Mirwais Farahi, Doreswamy, “Performance Evaluation of Machine Learning Classifiers for Epileptic Seizure Detection,” International Journal of Computer Sciences and Engineering, Vol.7, Issue.8, pp.122-129, 2019.
MLA Citation
MLA Style Citation: Mirwais Farahi, Doreswamy "Performance Evaluation of Machine Learning Classifiers for Epileptic Seizure Detection." International Journal of Computer Sciences and Engineering 7.8 (2019): 122-129.
APA Citation
APA Style Citation: Mirwais Farahi, Doreswamy, (2019). Performance Evaluation of Machine Learning Classifiers for Epileptic Seizure Detection. International Journal of Computer Sciences and Engineering, 7(8), 122-129.
BibTex Citation
BibTex Style Citation:
@article{Farahi_2019,
author = {Mirwais Farahi, Doreswamy},
title = {Performance Evaluation of Machine Learning Classifiers for Epileptic Seizure Detection},
journal = {International Journal of Computer Sciences and Engineering},
issue_date = {8 2019},
volume = {7},
Issue = {8},
month = {8},
year = {2019},
issn = {2347-2693},
pages = {122-129},
url = {https://www.ijcseonline.org/full_paper_view.php?paper_id=4799},
doi = {https://doi.org/10.26438/ijcse/v7i8.122129}
publisher = {IJCSE, Indore, INDIA},
}
RIS Citation
RIS Style Citation:
TY - JOUR
DO = {https://doi.org/10.26438/ijcse/v7i8.122129}
UR - https://www.ijcseonline.org/full_paper_view.php?paper_id=4799
TI - Performance Evaluation of Machine Learning Classifiers for Epileptic Seizure Detection
T2 - International Journal of Computer Sciences and Engineering
AU - Mirwais Farahi, Doreswamy
PY - 2019
DA - 2019/08/31
PB - IJCSE, Indore, INDIA
SP - 122-129
IS - 8
VL - 7
SN - 2347-2693
ER -
![]() |
![]() |
![]() |
1038 | 598 downloads | 233 downloads |
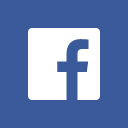
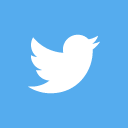
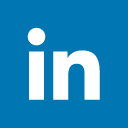
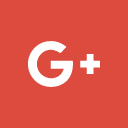
Abstract
Epilepsy is a neurological disorder in the human brain, which is characterized by chronic disorders and occurs at random to interrupt the normal function of the brain. The diagnosis and analysis of epileptic seizure is made with the help of Electroencephalography (EEG). In order to detect seizure, this study aims to construct an automatic seizure detection system to analyze epileptic EEG signals. The CHB-MIT Scalp EEG dataset is used for the experiment purpose. The Welch Fast Fourier Transform is applied to convert time-domain signals to frequency-domain. The statistical features are extracted from both time and frequency domains. The ANOVA based feature selection is used to select the most significant features. Data under-sampling and over-sampling techniques are used to balance the data. Eight machine learning algorithms, including Decision Tree, Extremely Randomized Decision Tree, Linear Discriminant Analysis, Quadratic Discriminant Analysis, Random Forest, Gradient Boosting, Multilayer Perceptron, and Stochastic Gradient Descent are used to classify the data. The highest result is recorded as 99.48% of accuracy, 99.79% of sensitivity, and 99.17% of specificity for the Extremely Randomized Decision Tree. The system might be a helpful tool for physicians to make a more reliable and objective analysis of a patient`s EEG records.
Key-Words / Index Term
Epilepsy, Electroencephalogram, Welch Fast Fourier Transform, Data Sampling techniques, Machine Learning Algorithms
References
[1]. Fisher, Robert S., Walter Van Emde Boas, Warren Blume, Christian Elger, Pierre Genton, Phillip Lee, and Jerome Engel Jr. "Epileptic seizures and epilepsy: definitions proposed by the International League Against Epilepsy (ILAE) and the International Bureau for Epilepsy (IBE)." Epilepsia 46, no. 4 (2005): 470-472.
[2]. Supriya, Siuly, Siuly Siuly, and Yanchun Zhang. "Automatic epilepsy detection from EEG introducing a new edge weight method in the complex network." Electronics Letters 52, no. 17 (2016): 1430-1432.
[3]. Fazel, Seena, Achim Wolf, Niklas Långström, Charles R. Newton, and Paul Lichtenstein. "Premature mortality in epilepsy and the role of psychiatric comorbidity: a total population study." The Lancet 382, no. 9905 (2013): 1646-1654.
[4]. Keusch, Gerald T., Joan Wilentz, and Arthur Kleinman. "Stigma and global health: developing a research agenda." The Lancet 367, no. 9509 (2006): 525-527.
[5]. Hirtz, D., D. J. Thurman, K. Gwinn-Hardy, M. Mohamed, A. R. Chaudhuri, and R. Zalutsky. "How common are the “common” neurologic disorders?." Neurology 68, no. 5 (2007): 326-337.
[6]. Sirven, Joseph I. "Epilepsy: a spectrum disorder." Cold Spring Harbor perspectives in medicine 5, no. 9 (2015): a022848.
[7]. Wu, Jimmy Ming-Tai, Meng-Hsiun Tsai, Chia-Te Hsu, Hsien-Chung Huang, and Hsiang-Chun Chen. "Intelligent Signal Classifier for Brain Epileptic EEG Based on Decision Tree, Multilayer Perceptron and Over-Sampling Approach." In Future of Information and Communication Conference, pp. 11-24. Springer, Cham, 2019.
[8]. Siuly, Siuly, Yan Li, and Yanchun Zhang. "EEG signal analysis and classification." IEEE Transactions on Neural Systems and Rehabilitaiton Engineering 11 (2016): 141-144.
[9]. Yoo, Jerald, and Muhammad Awais Bin Altaf. "Machine-based patient-specific seizure classification system." U.S. Patent 9,848,793, issued December 26, 2017.
[10]. Ayoubian, Lacoma, H. Lacoma, and J. Gotman. "Automatic seizure detection in SEEG using high frequency activities in wavelet domain." Medical engineering & physics 35, no. 3 (2013): 319-328.
[11]. Hopfengärtner, Rüdiger, Burkhard S. Kasper, Wolfgang Graf, Stephanie Gollwitzer, Gernot Kreiselmeyer, Hermann Stefan, and Hajo Hamer. "Automatic seizure detection in long-term scalp EEG using an adaptive thresholding technique: a validation study for clinical routine." Clinical Neurophysiology 125, no. 7 (2014): 1346-1352.
[12]. Hunyadi, Borbála, Marco Signoretto, Wim Van Paesschen, Johan AK Suykens, Sabine Van Huffel, and Maarten De Vos. "Incorporating structural information from the multichannel EEG improves patient-specific seizure detection." Clinical Neurophysiology 123, no. 12 (2012): 2352-2361.
[13]. Yoo, Jerald, Long Yan, Dina El-Damak, Muhammad Bin Altaf, Ali Shoeb, Hoi-Jun Yoo, and Anantha Chandrakasan. "An 8-channel scalable EEG acquisition SoC with fully integrated patient-specific seizure classification and recording processor." In 2012 IEEE International Solid-State Circuits Conference, pp. 292-294. IEEE, 2012.
[14]. Harpale, Varsha, and Vinayak Bairagi. "An adaptive method for feature selection and extraction for classification of epileptic EEG signal in significant states." Journal of King Saud University-Computer and Information Sciences (2018).
[15]. Fergus, Paul, A. Hussain, David Hignett, Dhiya Al-Jumeily, Khaled Abdel-Aziz, and Hani Hamdan. "A machine learning system for automated whole-brain seizure detection." Applied Computing and Informatics 12, no. 1 (2016): 70-89.
[16]. Shanir, PP Muhammed, Kashif Ahmad Khan, Yusuf Uzzaman Khan, Omar Farooq, and Hojjat Adeli. "Automatic seizure detection based on morphological features using one-dimensional local binary pattern on long-term EEG." Clinical EEG and neuroscience 49, no. 5 (2018): 351-362.
[17]. Orosco, Lorena, Agustina Garcés Correa, Pablo Diez, and Eric Laciar. "Patient non-specific algorithm for seizures detection in scalp EEG." Computers in biology and medicine 71 (2016): 128-134.
[18]. Chakrabarti, Satarupa, Aleena Swetapadma, Prasant Kumar Pattnaik, and Tina Samajdar. "Pediatric Seizure prediction from EEG signals based on unsupervised learning techniques using various distance measures." In 2017 1st International Conference on Electronics, Materials Engineering and Nano-Technology (IEMENTech), pp. 1-5. IEEE, 2017.
[19]. Hosseini, Mohammad-Parsa, Dario Pompili, Kost Elisevich, and Hamid Soltanian-Zadeh. "Random ensemble learning for EEG classification." Artificial intelligence in medicine 84 (2018): 146-158.
[20]. Shoeb, Ali Hossam. "Application of machine learning to epileptic seizure onset detection and treatment." PhD diss., Massachusetts Institute of Technology, 2009.
[21]. Chen, Lan-Lan, Jian Zhang, Jun-Zhong Zou, Chen-Jie Zhao, and Gui-Song Wang. "A framework on wavelet-based nonlinear features and extreme learning machine for epileptic seizure detection." Biomedical Signal Processing and Control 10 (2014): 1-10.
[22]. Welch, Peter. "The use of fast Fourier transform for the estimation of power spectra: a method based on time averaging over short, modified periodograms." IEEE Transactions on audio and electroacoustics 15, no. 2 (1967): 70-73.
[23]. Akhilesh Krishna, P. R., and Jency Andrews. "PSD computation using modified Welch algorithm." Int J Sci Res Eng Technol (IJSRET) 4, no. 9 (2015): 951-954.
[24]. Bennasar, Mohamed, Yulia Hicks, and Rossitza Setchi. "Feature selection using joint mutual information maximisation." Expert Systems with Applications 42, no. 22 (2015): 8520-8532.
[25]. Lee, Sang-Hong, and Joon S. Lim. "Minimum feature selection for epileptic seizure classification using wavelet-based feature extraction and a fuzzy neural network." Applied Mathematics & Information Sciences 8, no. 3 (2014): 1295.
[26]. Penny, W., and R. Henson. "Analysis of variance." Statistical parametric mapping: The analysis of functional brain images (2006): 166-177.
[27]. Chowdhury, Tanima Tasmin, Shaikh Anowarul Fattah, and Celia Shahnaz. "Classification of seizure and non-seizure activity in seizure patients using time-frequency domain processing of gamma band EEG signals." In 2017 4th International Conference on Advances in Electrical Engineering (ICAEE), pp. 537-540. IEEE, 2017.
[28]. Nuzzo, Regina. "Scientific method: statistical errors." Nature News 506, no. 7487 (2014): 150.
[29]. Al Shalabi, Luai, Zyad Shaaban, and Basel Kasasbeh. "Data mining: A preprocessing engine." Journal of Computer Science 2, no. 9 (2006): 735-739.
[30]. Patro, S., and Kishore Kumar Sahu. "Normalization: A preprocessing stage." arXiv preprint arXiv:1503.06462 (2015).
[31]. Liu, Alexander, Joydeep Ghosh, and Cheryl E. Martin. "Generative Oversampling for Mining Imbalanced Datasets." In DMIN, pp. 66-72. 2007.
[32]. Chawla, Nitesh V., Kevin W. Bowyer, Lawrence O. Hall, and W. Philip Kegelmeyer. "SMOTE: synthetic minority over-sampling technique." Journal of artificial intelligence research 16 (2002): 321-357.
[33]. Breiman, Leo. Classification and regression trees. Routledge, 2017.
[34]. Geurts, Pierre, Damien Ernst, and Louis Wehenkel. "Extremely randomized trees." Machine learning 63, no. 1 (2006): 3-42.
[35]. Lotte, Fabien, Marco Congedo, Anatole Lécuyer, Fabrice Lamarche, and Bruno Arnaldi. "A review of classification algorithms for EEG-based brain–computer interfaces." Journal of neural engineering 4, no. 2 (2007): R1.
[36]. Deepika Mallampati, "An Efficient Spam Filtering using Supervised Machine Learning Techniques", International Journal of Scientific Research in Computer Science and Engineering, Vol.6, Issue.2, pp.33-37, 2018.
[37]. Kim, Kang Soo, Heung Ho Choi, Chang Soo Moon, and Chi Woong Mun. "Comparison of k-nearest neighbor, quadratic discriminant and linear discriminant analysis in classification of electromyogram signals based on the wrist-motion directions." Current applied physics 11, no. 3 (2011): 740-745.
[38]. Breiman, L. "Random forests machine learning. 45: 5–32." View Article PubMed/NCBI Google Scholar (2001).
[39]. Friedman, Jerome H. "Greedy function approximation: a gradient boosting machine." Annals of statistics (2001): 1189-1232.
[40]. Hauck, Trent. scikit-learn Cookbook. Packt Publishing Ltd, 2014.
[41]. Rohini M., Arsha P., "Detection of Microaneurysm using Machine Learning Techniques", International Journal of Scientific Research in Network Security and Communication, Vol.7, Issue.3, pp.1-6, 2019
[42]. Chu, Hyunho, Chun Kee Chung, Woorim Jeong, and Kwang-Hyun Cho. "Predicting epileptic seizures from scalp EEG based on attractor state analysis." Computer methods and programs in biomedicine 143 (2017): 75-87.
[43]. Liang, Weixia, Haijun Pei, Qingling Cai, and Yonghua Wang. "Scalp EEG epileptogenic zone recognition and localization based on long-term recurrent convolutional network." Neurocomputing (2019).
[44]. Yuvaraj, Rajamanickam, John Thomas, Tilmann Kluge, and Justin Dauwels. "A deep Learning Scheme for Automatic Seizure Detection from Long-Term Scalp EEG." In 2018 52nd Asilomar Conference on Signals, Systems, and Computers, pp. 368-372. IEEE, 2018.