Particle Swarm Optimization based Feature Selection with Evolutionary Outlay-Aware Deep Belief Network Classifier (PSO-EOA-DBNC) for High Dimensional Datasets
M. Praveena1 , V. Jaiganesh2
Section:Research Paper, Product Type: Journal Paper
Volume-7 ,
Issue-8 , Page no. 61-69, Aug-2019
CrossRef-DOI: https://doi.org/10.26438/ijcse/v7i8.6169
Online published on Aug 31, 2019
Copyright © M. Praveena, V. Jaiganesh . This is an open access article distributed under the Creative Commons Attribution License, which permits unrestricted use, distribution, and reproduction in any medium, provided the original work is properly cited.
View this paper at Google Scholar | DPI Digital Library
How to Cite this Paper
- IEEE Citation
- MLA Citation
- APA Citation
- BibTex Citation
- RIS Citation
IEEE Citation
IEEE Style Citation: M. Praveena, V. Jaiganesh, “Particle Swarm Optimization based Feature Selection with Evolutionary Outlay-Aware Deep Belief Network Classifier (PSO-EOA-DBNC) for High Dimensional Datasets,” International Journal of Computer Sciences and Engineering, Vol.7, Issue.8, pp.61-69, 2019.
MLA Citation
MLA Style Citation: M. Praveena, V. Jaiganesh "Particle Swarm Optimization based Feature Selection with Evolutionary Outlay-Aware Deep Belief Network Classifier (PSO-EOA-DBNC) for High Dimensional Datasets." International Journal of Computer Sciences and Engineering 7.8 (2019): 61-69.
APA Citation
APA Style Citation: M. Praveena, V. Jaiganesh, (2019). Particle Swarm Optimization based Feature Selection with Evolutionary Outlay-Aware Deep Belief Network Classifier (PSO-EOA-DBNC) for High Dimensional Datasets. International Journal of Computer Sciences and Engineering, 7(8), 61-69.
BibTex Citation
BibTex Style Citation:
@article{Praveena_2019,
author = {M. Praveena, V. Jaiganesh},
title = {Particle Swarm Optimization based Feature Selection with Evolutionary Outlay-Aware Deep Belief Network Classifier (PSO-EOA-DBNC) for High Dimensional Datasets},
journal = {International Journal of Computer Sciences and Engineering},
issue_date = {8 2019},
volume = {7},
Issue = {8},
month = {8},
year = {2019},
issn = {2347-2693},
pages = {61-69},
url = {https://www.ijcseonline.org/full_paper_view.php?paper_id=4790},
doi = {https://doi.org/10.26438/ijcse/v7i8.6169}
publisher = {IJCSE, Indore, INDIA},
}
RIS Citation
RIS Style Citation:
TY - JOUR
DO = {https://doi.org/10.26438/ijcse/v7i8.6169}
UR - https://www.ijcseonline.org/full_paper_view.php?paper_id=4790
TI - Particle Swarm Optimization based Feature Selection with Evolutionary Outlay-Aware Deep Belief Network Classifier (PSO-EOA-DBNC) for High Dimensional Datasets
T2 - International Journal of Computer Sciences and Engineering
AU - M. Praveena, V. Jaiganesh
PY - 2019
DA - 2019/08/31
PB - IJCSE, Indore, INDIA
SP - 61-69
IS - 8
VL - 7
SN - 2347-2693
ER -
![]() |
![]() |
![]() |
507 | 384 downloads | 250 downloads |
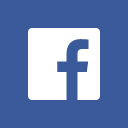
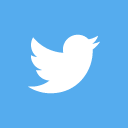
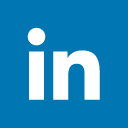
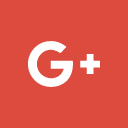
Abstract
Data mining research extends its wings to several domains and classification is one of the thrust areas for researchers. The curse of dimensionality is reduced by many optimization techniques and machine learning algorithms. In this research work, a particle swarm optimization based feature selection method is employed to deal with the curse of dimensionality. The PSO algorithm makes use of the fitness function that is obtained from the evolutionary outlay aware deep belief network which conducts classification. 20 datasets are taken for evaluating the conductance of the PSO – EOA – DBNC in terms of classification accuracy and elapsed time. From the results it is significant to notice that PSO-EOA-DBNC out conducts than that of other classifiers.
Key-Words / Index Term
data mining, feature selection, particle swarm optimization, deep belief network, evolutionary algorithm.
References
[1] D. Polat, Z. Çataltepe, “Feature selection and classification on brain computer interface (BCI) data”, in Proceedings of the 2012 20th Signal Processing and Communications Applications Conference (SIU), IEEE, 2012, pp. 1–4.
[2] G.P. Zhang, “Neural networks for classification: a survey”, IEEE Trans. Syst. Man Cybern. Part C: Appl. Rev. 30 (4) (2000) 451–462.
[3] D.D. Lewis, “Naive (Bayes) at forty: the independence assumption in information retrieval, in Machine Learning”: ECML-98, Springer, Berlin, Heidelberg, 1998, pp. 4–15.
[4] Kuan-Cheng Lin, Kai-Yuan Zhang, Yi-Hung Huang, Jason C Hung, Neil Yen, “Feature selection based on an improved cat swarm optimization algorithm for big data classification”, J. Super computer. 72 (8) (2016) 3210–3221.
[5] Kuan-Cheng Lin, Yi-Hung Huang, Jason C. Hung, Yung-Tso Lin, “Feature selection and parameter optimization of support vector machines based on modified cat swarm optimization”, Int. J. Distributed Sensational Network 2015 (2015).
[6] Kuan-Cheng Lin, Sih-Yang Chen, Jason C. Hung, “Feature selection and parameter optimization of support vector machines based on modified artificial fish swarm algorithms”, Mathematical Probability Eng.( 2015 ).
[7] C.A. Pena-Reyes, M. Sipper, “Evolutionary computation in medicine: an overview, Artificial Intelligence”, Med. 19 (1) (2000) 1–23.
[8] S.H. Cha, C. Tappert, “A genetic algorithm for constructing compact binary decision trees”, J. Pattern Recognition. Res. 4 (1) (2009) 1–13.
[9] J. Kennedy, “Particle swarms optimization, in Encyclopaedia of Machine Learning”, Springer, US, 2010, pp. 760–766.
[10] P.P. Brahma, D. Wu, Y. She, “Why Deep Learning Works: A Manifold Disentanglement Perspective”, IEEE Transactions on Neural Networks & Learning Systems, 2016, 27(10):1997-2008.
[11] D. Li, S. Y. Dong, “Deep learning: methods and applications, Foundations & Trends in Information Retrieval”, 2014, 7(3):197-387.
[12] R. Salakhutdinov, G. Hinton,” An efficient learning procedure for deep Boltzmann machines, Neural Computation”, 2012, 24(8):1967.
[13] M.Praveena, Dr.V.Jaiganesh, “Improved Genetic Algorithm Based Feature Selection Strategy Based Five Layered Artificial Neural Network Classifier (IGA – FLANN)”, International Journal of Engineering and Techniques - Volume 3 Issue 5, Sep - Oct 2017, 199-213.
[14] M.Praveena, Dr.V.Jaiganesh, “Routine Correspondence Method with Grey Wolf Optimization based Imperforate Support Vector Machine Classifier (ISVMC) for High Dimensional Datasets”, Journal of Advanced Research in Dynamical & Control Systems, Vol. 11, 01-Special Issue, 2019, 652-660.
[15] M.Praveena, Dr.V.Jaiganesh, “Adaptive Particle Swarm Optimization based Credentialed Extreme Learning Machine Classifier (APSO-CELMC) for High Dimensional Datasets”, International Journal of Innovative Technology and Exploring Engineering (IJITEE) ISSN: 2278-3075, Volume-8, Issue-10S, August 2019.
[16] M.Praveena, Dr.V.Jaiganesh, “A Literature Review on Supervised Machine Learning Algorithms and Boosting Process”, International Journal of Computer Applications (0975 – 8887) Volume 169 – No.8, July 2017.