Plant Disease Detection Methods using Image Processing
Pankaj Gumber1 , Lal Chand2
Section:Review Paper, Product Type: Journal Paper
Volume-7 ,
Issue-7 , Page no. 391-395, Jul-2019
CrossRef-DOI: https://doi.org/10.26438/ijcse/v7i7.391395
Online published on Jul 31, 2019
Copyright © Pankaj Gumber, Lal Chand . This is an open access article distributed under the Creative Commons Attribution License, which permits unrestricted use, distribution, and reproduction in any medium, provided the original work is properly cited.
View this paper at Google Scholar | DPI Digital Library
How to Cite this Paper
- IEEE Citation
- MLA Citation
- APA Citation
- BibTex Citation
- RIS Citation
IEEE Style Citation: Pankaj Gumber, Lal Chand, “Plant Disease Detection Methods using Image Processing,” International Journal of Computer Sciences and Engineering, Vol.7, Issue.7, pp.391-395, 2019.
MLA Style Citation: Pankaj Gumber, Lal Chand "Plant Disease Detection Methods using Image Processing." International Journal of Computer Sciences and Engineering 7.7 (2019): 391-395.
APA Style Citation: Pankaj Gumber, Lal Chand, (2019). Plant Disease Detection Methods using Image Processing. International Journal of Computer Sciences and Engineering, 7(7), 391-395.
BibTex Style Citation:
@article{Gumber_2019,
author = {Pankaj Gumber, Lal Chand},
title = {Plant Disease Detection Methods using Image Processing},
journal = {International Journal of Computer Sciences and Engineering},
issue_date = {7 2019},
volume = {7},
Issue = {7},
month = {7},
year = {2019},
issn = {2347-2693},
pages = {391-395},
url = {https://www.ijcseonline.org/full_paper_view.php?paper_id=4776},
doi = {https://doi.org/10.26438/ijcse/v7i7.391395}
publisher = {IJCSE, Indore, INDIA},
}
RIS Style Citation:
TY - JOUR
DO = {https://doi.org/10.26438/ijcse/v7i7.391395}
UR - https://www.ijcseonline.org/full_paper_view.php?paper_id=4776
TI - Plant Disease Detection Methods using Image Processing
T2 - International Journal of Computer Sciences and Engineering
AU - Pankaj Gumber, Lal Chand
PY - 2019
DA - 2019/07/31
PB - IJCSE, Indore, INDIA
SP - 391-395
IS - 7
VL - 7
SN - 2347-2693
ER -
![]() |
![]() |
![]() |
497 | 273 downloads | 193 downloads |
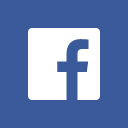
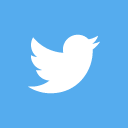
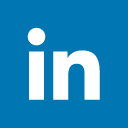
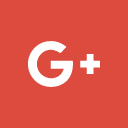
Abstract
The image processing is the technique which can process the information stored in the form of pixels. The disease of the plants can be detected using the methods of image processing. The plant image has various types of noises which can affect accuracy of plant disease detection. In this work, various image de noising methods are reviewed and analyzed in terms of certain parameters
Key-Words / Index Term
Plant disease detection, De noising, feature extraction
References
[1] Jayamala K. Patil and Raj Kumar, “ Advance in image Processing for detection of plant disease”, Journal of Advanced Bioinformatics Applications and Research ISSN 0976-2604 Vol 2, Issue 2, June-2011, pp 135-141
[2] Laxman Poudal , Bhola Thapa, Bim “Application of Digital Image Processing for Shape Characterization of Sand Particles”, 2011
[3] Jie Zhao, Xu Zhao and Yuncai Liu, “A Method for Detection and Classification of Glass Defects in Low Resolution Images,” Sixth International Conference on Image and Graphics, 2011, pp.642-647.
[4] Luo Juan & Oubong Gwun,” A Comparison of SIFT, PCA-SIFT and SURF, International Journal of Image Processing (IJIP) Volume(3), Issue(4),2009,pp. 143-152
[5] F. Adamo, F. Attivissimo, A. Di Nisio, M. Savino, An Automated visual inspection system for the glass industry,In: Proc. of 16th IMEKO TC4 Symposium, Florence, Italy, Sept. 22–24, 2008.
[6] Krystian Mikolajczyk and Cordelia Schmid,” A performance evaluation of local descriptors”, Pattern Analysis and Machine Intelligence, IEEE Transactions on Pattern Analysis and Machine Intelligence ,Volume 27 , Issue 10 ,2005, pp 1615 – 1630.
[7] Yan K, Rahul Sukthankar, “pca-sift: a more distinctive representation for local image descriptors” Computer Vision and Pattern Recognition, 2004, CVPR 2004.,Proceedings of the 2004, IEEE Computer Society Conference on ,2004 pp.506-513.
[8] K. Seeliger, M. Fritsche, U. Guclu, S. Schoenmakers, J.-M. Schoffelen, S. E. Bosch, M. A. J. van Gerven, “Convolutional Neural Network-based Encoding and Decoding of Visual Object Recognition in Space and Time”, 2017, ScienceDirect
[9] Edna Chebet Too, Li Yujian, Sam Njuki, Liu Yingchun, “A comparative study of fine-tuning deep learning models for plant disease identification”, 2018, Computers and Electronics in Agriculture
[10] Ye Xu, Yun Chi, Ye Tian, “Deep Convolutional Neural Networks for Feature Extraction of Images Generated from Complex Networks Topologies”, Springer Science+Business Media, LLC, part of Springer Nature 2018
[11] Konstantinos P. Ferentinos, “Deep learning models for plant disease detection and diagnosis”, Computers and Electronics in Agriculture 145 (2018) 311–318
[12] Xiaolong Zhu, Meng Zhu, Honge Ren, “Method of plant leaf recognition based on improved deep convolutional neural network”, Cognitive Systems Research 52 (2018) 223–233
[13] Soniya, Sandeep Paul, Lotika Singh, “A Review on Advances in Deep Learning”, 2015, IEEE
[14] Jayme Garcia, Arnal Barbedo, “A review on the main challenges in automatic plant disease identification based on visible range images”, 2016, Biosystem Engineering 144, 52-60
[15] Weibo Liu, Zidong Wang, Xiaohui Liu, Nianyin Zeng, Yurong Liu, and Fuad E. Alsaadi, “A Survey of Deep Neural Network Architectures and Their Applications”, 2016, Neurocomputing; ScienceDirect Publications
[16] Federico Martinelli, Riccardo Scalenghe, Salvatore Davino, Stefano Panno, Giuseppe Scuderi, Paolo Ruisi, Paolo Villa, Daniela Stroppiana, Mirco Boschetti, Luiz R. Goulart, “Advanced methods of plant disease detection. A review”, Springer, 2014
[17] Jayme Garcia, Arnal Barbedo, “An Automatic Method to Detect and Measure Leaf Disease Symptoms Using Digital Image Processing”, 2014 The American Phytopathological Society