High Confidence Association Rule for Product Selling Strategy
Mamata S. Kalas1 , Amruta G. Unne2
Section:Review Paper, Product Type: Journal Paper
Volume-7 ,
Issue-6 , Page no. 1184-1188, Jun-2019
CrossRef-DOI: https://doi.org/10.26438/ijcse/v7i6.11841188
Online published on Jun 30, 2019
Copyright © Mamata S. Kalas, Amruta G. Unne . This is an open access article distributed under the Creative Commons Attribution License, which permits unrestricted use, distribution, and reproduction in any medium, provided the original work is properly cited.
View this paper at Google Scholar | DPI Digital Library
How to Cite this Paper
- IEEE Citation
- MLA Citation
- APA Citation
- BibTex Citation
- RIS Citation
IEEE Style Citation: Mamata S. Kalas, Amruta G. Unne, “High Confidence Association Rule for Product Selling Strategy,” International Journal of Computer Sciences and Engineering, Vol.7, Issue.6, pp.1184-1188, 2019.
MLA Style Citation: Mamata S. Kalas, Amruta G. Unne "High Confidence Association Rule for Product Selling Strategy." International Journal of Computer Sciences and Engineering 7.6 (2019): 1184-1188.
APA Style Citation: Mamata S. Kalas, Amruta G. Unne, (2019). High Confidence Association Rule for Product Selling Strategy. International Journal of Computer Sciences and Engineering, 7(6), 1184-1188.
BibTex Style Citation:
@article{Kalas_2019,
author = {Mamata S. Kalas, Amruta G. Unne},
title = {High Confidence Association Rule for Product Selling Strategy},
journal = {International Journal of Computer Sciences and Engineering},
issue_date = {6 2019},
volume = {7},
Issue = {6},
month = {6},
year = {2019},
issn = {2347-2693},
pages = {1184-1188},
url = {https://www.ijcseonline.org/full_paper_view.php?paper_id=4704},
doi = {https://doi.org/10.26438/ijcse/v7i6.11841188}
publisher = {IJCSE, Indore, INDIA},
}
RIS Style Citation:
TY - JOUR
DO = {https://doi.org/10.26438/ijcse/v7i6.11841188}
UR - https://www.ijcseonline.org/full_paper_view.php?paper_id=4704
TI - High Confidence Association Rule for Product Selling Strategy
T2 - International Journal of Computer Sciences and Engineering
AU - Mamata S. Kalas, Amruta G. Unne
PY - 2019
DA - 2019/06/30
PB - IJCSE, Indore, INDIA
SP - 1184-1188
IS - 6
VL - 7
SN - 2347-2693
ER -
![]() |
![]() |
![]() |
336 | 310 downloads | 179 downloads |
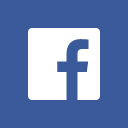
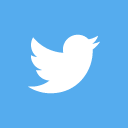
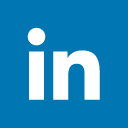
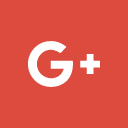
Abstract
Mining association rules help data owners to unveil hidden patterns from their data to analyze & predict the operation on application domain. However, mining rules in a distributed environment is not a minor task due to privacy concerns. Data owners are interested in collaborating to mine rules on different levels; however, they are concerned that sensitive information related to somebody involved in their database might get compromised during the mining process. Here formulate the problem to solving association rules queries in a environment such that the mining process is confidential and the outcomes are differentially private. Work proposes a privacy-preserving association rules mining where strong association rules are determined privately, and the results returned satisfy differential privacy. Finally done experiments on real-life data it shows that designed approach can efficiently answer association rules queries and is scalable with increasing data records.
Key-Words / Index Term
Association rules mining, Data Privacy, Data Mining, High confidence
References
[1] R. Agrawal and R. Srikant. “Privacy preserving data mining”, InProceedings of International Conference on Management of Data (ACMSIGMOD), 2000.
[2] R. Bhaskar, S. Laxman, A. Smith, and A. Thakurta. “Discoveringfrequent patterns in sensitive data”. In Proc. of Intl. Conf. on Knowledge Discovery and Data Mining (KDD), pages 503–512, 2010.
[3] R. Chen, B. C. Fung, B. C. Desai, and N. M. Sossou. “Differentiallyprivate transit data publication: a case study on the Montrealtransportation system”. In Proc. of Intl. Conf. on Knowledge Discoveryand Data Mining (KDD), pages 213–221, 2012.
[4] G. Cormode, C. Procopiuc, E. Shen, D. Srivastava, and T. Yu.”Differentially private spatial decompositions.” In ICDE, pages 20–31, 2012.
[5] C. Dwork, F. McSherry, K. Nissim, and A. Smith. “Calibrating noiseto sensitivity in private data analysis”. In TCC, pages 265–284, 2006.
[6] C. Dwork, M. Naor, O. Reingold, G. N. Rothblum, and S. Vadhan.”On the complexity of differentially private data release: Efficient algorithms and hardness results”. In ACM Symposium on Theory ofComputing, pages 381–390, 2009.
[7] C. Dwork and A. Roth. “The algorithmic foundations of differentialprivacy”. Foundations and Trends in Theoretical Computer Science,9(34):211–407, 2014.
[8] A. Friedman and A. Schuster. “Data mining with differentialprivacy”. In Proc. of Intl. Conf. on Knowledge Discovery and DataMining (KDD), pages 493–502, 2010.
[9] A. Ghosh, T. Roughgarden, and M. Sundararajan. “Universally utility-maximizing privacy mechanisms”. In ACM Symposium onTheory of Computing, pages 351–360, 2009.
[10] Omar Abdel Wahab, Moulay Omar Hachami etal “DARM: A Privacy-preserving Approach for Distributed Association Rules Mining on Horizontally-partitioned Data”. Conference Paper • July 2014 DOI: 10.1145/2628194.2628206
[11] Pradeep Chouksey, "Mining Frequent model Using mass-produced Approach", International Journal of Scientific Research in Computer Science and Engineering, Vol.5, Issue.4, pp.89-94, 2017
[12] P.V. Nikam, D.S. Deshpande, "Different Approaches for Frequent Itemset Mining", International Journal of Scientific Research in Computer Science and Engineering, Vol.6, Issue.2, pp.10-14, 2018