Indirect Occupancy Detection using Environmental SensorData for Smart Office Buildings
C. Durai1
Section:Research Paper, Product Type: Journal Paper
Volume-7 ,
Issue-6 , Page no. 1092-1095, Jun-2019
CrossRef-DOI: https://doi.org/10.26438/ijcse/v7i6.10921095
Online published on Jun 30, 2019
Copyright © C. Durai . This is an open access article distributed under the Creative Commons Attribution License, which permits unrestricted use, distribution, and reproduction in any medium, provided the original work is properly cited.
View this paper at Google Scholar | DPI Digital Library
How to Cite this Paper
- IEEE Citation
- MLA Citation
- APA Citation
- BibTex Citation
- RIS Citation
IEEE Style Citation: C. Durai, “Indirect Occupancy Detection using Environmental SensorData for Smart Office Buildings,” International Journal of Computer Sciences and Engineering, Vol.7, Issue.6, pp.1092-1095, 2019.
MLA Style Citation: C. Durai "Indirect Occupancy Detection using Environmental SensorData for Smart Office Buildings." International Journal of Computer Sciences and Engineering 7.6 (2019): 1092-1095.
APA Style Citation: C. Durai, (2019). Indirect Occupancy Detection using Environmental SensorData for Smart Office Buildings. International Journal of Computer Sciences and Engineering, 7(6), 1092-1095.
BibTex Style Citation:
@article{Durai_2019,
author = {C. Durai},
title = {Indirect Occupancy Detection using Environmental SensorData for Smart Office Buildings},
journal = {International Journal of Computer Sciences and Engineering},
issue_date = {6 2019},
volume = {7},
Issue = {6},
month = {6},
year = {2019},
issn = {2347-2693},
pages = {1092-1095},
url = {https://www.ijcseonline.org/full_paper_view.php?paper_id=4686},
doi = {https://doi.org/10.26438/ijcse/v7i6.10921095}
publisher = {IJCSE, Indore, INDIA},
}
RIS Style Citation:
TY - JOUR
DO = {https://doi.org/10.26438/ijcse/v7i6.10921095}
UR - https://www.ijcseonline.org/full_paper_view.php?paper_id=4686
TI - Indirect Occupancy Detection using Environmental SensorData for Smart Office Buildings
T2 - International Journal of Computer Sciences and Engineering
AU - C. Durai
PY - 2019
DA - 2019/06/30
PB - IJCSE, Indore, INDIA
SP - 1092-1095
IS - 6
VL - 7
SN - 2347-2693
ER -
![]() |
![]() |
![]() |
322 | 309 downloads | 185 downloads |
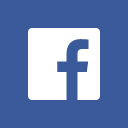
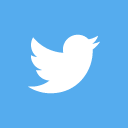
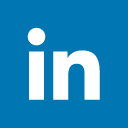
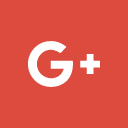
Abstract
Buildings are one of the largest energy consumers around the world. Towards reducing the energy wastage in buildings, occupancy based control systems are becoming more wide-spread in commercial office buildings. Accurate identification of occupancy is crucial for such automated energy monitoring systems and for other potential applications such as personal comfort, air quality, and energy auditing. However, efficient and accurate ways to identifying occupancy in large scale buildings is a challenging task. Several approaches have been studied in literature ranging from direct to indirect approaches. In this paper, we present a nonintrusive and indirect occupancy detection approach using environmental sensor data as proxy. Specially, we used temperature and Carbon dioxide sensor dataset available in public domain. We employed the wide used k-means clustered algorithm for identifying occupied and unoccupied state (binary occupancy) of an office room. The proposed approach is validated on a public dataset with one week of environmental sensor data and results are analyzed using confusion matrix. Our experimental results show that the accuracy of detecting binary occupancy is 87.57%. We planned to extend our approach using other environmental sensor data.
Key-Words / Index Term
Smart buildings, energy management, occupancy detection, data mining, and clustering
References
[1] IEA, World Energy Outlook, IEA Publications, Paris, 2013, pp. 2013
[2] S. D’Oca, T. Hong, and J. Langevin, "The human dimensions of energy use in buildings: A review" Renewable and Sustainable Energy Reviews, vol. 81, pp. 731–742, 2018.
[3] Rashid, H., Arjunan, P., Singh, P. and Singh, A., 2016, June. Collect, compare, and score: a generic data-driven anomaly detection method for buildings. In Proceedings of the Seventh International Conference on Future Energy Systems Poster Sessions (p. 12). ACM.
[4] V. Garg, N. K., Bansal, Smart occupancy sensors to reduce energy consumption. Energy and Buildings, 2000, 32(1):81-87.
[5] J. Brooks, S. Goyal, R. Subramany, Y. Lin, T. Middelkoop, L. Arpan, L. Carloni, P. Barooah, An experimental investigation of occupancy-based energy-efficient control of commercial building indoor climate, in: Proceeding of the IEEE 53rd Annual Conference on, IEEE, Decision and Control (CDC), Los Angeles, CA, 2014, pp. 5680–5685.
[6] Chen, Zhenghua, Chaoyang Jiang, and Lihua Xie. "Building occupancy estimation and detection: A review." Energy and Buildings (2018).
[7] Jung, Wooyoung, and Farrokh Jazizadeh. "Human-in-the-loop HVAC operations: A quantitative review on occupancy, comfort, and energy-efficiency dimensions." Applied Energy 239 (2019): 1471-1508.
[8] Duarte C, Van Den Wymelenberg K, Rieger C. Revealing occupancy patterns in an office building through the use of occupancy sensor data. Energy and Buildings 2013;67:587–95.
[9] Agarwal Y, Balaji B, Gupta R, Lyles J, Wei M, Weng T. Occupancy-driven energy management for smart building automation. Proceedings of the 2nd ACM workshop on embedded sensing systems for energy-efficiency in building. ACM; 2010.
[10] Kamthe A, Jiang L, Dudys M, Cerpa A. SCOPES: Smart cameras object position estimation system. Proceedings of the 6th European conference on wireless sensor networks. Cork, Ireland: Springer Verlag; 2009. p. 279–95.
[11] Y. Benezeth, H. Laurent, B. Emile, C. Rosenberger, Towards a sensor for detecting human presence and characterizing activity, Energy Build. 43 (2) (2011) 305–314.
[12] M. Jin, N. Bekiaris-Liberis, K. Weekly, C. Spanos, A. Bayen, Sensing by proxy: Occupancy detection based on indoor CO2 concentration, UBICOMM 2015, 2015, 14.
[13] L.M. Candanedo, V. Feldheim, Accurate occupancy detection of an office room from light, temperature, humidity and CO2 measurements using statistical learning models, Energy and Buildings, 2016, 112:28-39.
[14] A. Beltran, V.L. Erickson, A.E. Cerpa, Thermosense: occupancy thermal based sensing for hvac control, in: Proceedings of the 5th ACM Workshop on Embedded Systems For Energy-Efficient Buildings, ACM, Rome, Italy, 2013, pp. 11:11–11:18.
[15] N. Li, G. Calis, B. Becerik-Gerber, Measuring and monitoring occupancy with an RFID based system for demand-driven HVAC operations, Automat. Construct. 24 (2012) 89–99.
[16] E. Hailemariam, R. Goldstein, R. Attar, A. Khan, Real-time occupancy detection using decision trees with multiple sensor types, in: Proceedings of the 2011 Symposium on Simulation for Architecture and Urban Design, Society for Computer Simulation International, San Diego, CA, 2011, pp. 141–148.
[17] Z. Yang, N. Li, B. Becerik-Gerber, M. Orosz, A multi-sensor based occupancy estimation model for supporting demand driven HVAC operations, in: Proceedings of the 2012 Symposium on Simulation for Architecture and Urban Design, Society for Computer Simulation International, San Diego, CA, USA, 2012, pp. 49–56.
[18] Jain, Anil K. "Data clustering: 50 years beyond K-means." Pattern recognition letters 31, no. 8 (2010): 651-666.