An Ontology-Based Contextual Knowledge Representation for Semantic Image Segmentation
T. Sreedhar1 , S. Sathappan2
Section:Research Paper, Product Type: Journal Paper
Volume-7 ,
Issue-6 , Page no. 675-682, Jun-2019
CrossRef-DOI: https://doi.org/10.26438/ijcse/v7i6.675682
Online published on Jun 30, 2019
Copyright © T. Sreedhar, S. Sathappan . This is an open access article distributed under the Creative Commons Attribution License, which permits unrestricted use, distribution, and reproduction in any medium, provided the original work is properly cited.
View this paper at Google Scholar | DPI Digital Library
How to Cite this Paper
- IEEE Citation
- MLA Citation
- APA Citation
- BibTex Citation
- RIS Citation
IEEE Style Citation: T. Sreedhar, S. Sathappan, “An Ontology-Based Contextual Knowledge Representation for Semantic Image Segmentation,” International Journal of Computer Sciences and Engineering, Vol.7, Issue.6, pp.675-682, 2019.
MLA Style Citation: T. Sreedhar, S. Sathappan "An Ontology-Based Contextual Knowledge Representation for Semantic Image Segmentation." International Journal of Computer Sciences and Engineering 7.6 (2019): 675-682.
APA Style Citation: T. Sreedhar, S. Sathappan, (2019). An Ontology-Based Contextual Knowledge Representation for Semantic Image Segmentation. International Journal of Computer Sciences and Engineering, 7(6), 675-682.
BibTex Style Citation:
@article{Sreedhar_2019,
author = { T. Sreedhar, S. Sathappan},
title = {An Ontology-Based Contextual Knowledge Representation for Semantic Image Segmentation},
journal = {International Journal of Computer Sciences and Engineering},
issue_date = {6 2019},
volume = {7},
Issue = {6},
month = {6},
year = {2019},
issn = {2347-2693},
pages = {675-682},
url = {https://www.ijcseonline.org/full_paper_view.php?paper_id=4611},
doi = {https://doi.org/10.26438/ijcse/v7i6.675682}
publisher = {IJCSE, Indore, INDIA},
}
RIS Style Citation:
TY - JOUR
DO = {https://doi.org/10.26438/ijcse/v7i6.675682}
UR - https://www.ijcseonline.org/full_paper_view.php?paper_id=4611
TI - An Ontology-Based Contextual Knowledge Representation for Semantic Image Segmentation
T2 - International Journal of Computer Sciences and Engineering
AU - T. Sreedhar, S. Sathappan
PY - 2019
DA - 2019/06/30
PB - IJCSE, Indore, INDIA
SP - 675-682
IS - 6
VL - 7
SN - 2347-2693
ER -
![]() |
![]() |
![]() |
303 | 185 downloads | 123 downloads |
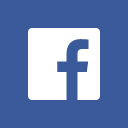
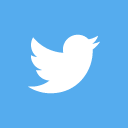
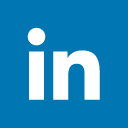
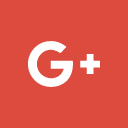
Abstract
Contextual Hierarchical Model (CHM) was a semantic image segmentation model which learned contextual information in a hierarchical framework. A Logistic Disjunctive Normal Networks (LDNN) classifier was used in each hierarchy level of CHM for semantic image segmentation. The class average accuracy of CHM may be affected due to the absence of global constraint. So, different Conditional Random Field (CRF) models were introduced to define global constraints through energy functions on a discrete random field. The efficiency of CHM based semantic image segmentation was greatly depended on the performance of LDNN. The performance of LDNN was enhanced by using a proximal gradient which minimizes the quadratic error of LDNN with fast convergence rate. Moreover, a Grey Wolf Optimization (GWO) algorithm was introduced to optimize the user specified weight and bias terms of LDNN which reduce the time complexity of LDNN. In this paper, CHM based semantic image segmentation is further improved by using ontology-based contextual knowledge representation in CHM. The ontology-based contextual knowledge representation constructs a relation based on taxonomic relations. In order to tackle the complex types of relations in images, a fuzzification is introduced in the ontology which is used to define the semantic relation between the concepts more effectively. Based on the fuzzified taxonomic relation, a relation is constructed which is given as additional input to the CHM for semantic image segmentation. The ontological taxonomic knowledge representation adjusts the segmentation results of CHM based on taxonomic relations. The experimental results show that the proposed Ontology-based contextual knowledge representation with CHM- Higher order Hierarchical CRF-Improved Optimized LDNN (OCHM-HHCRF-IOLDNN) has better performance in terms of class accuracy, pixel accuracy, F-measure and G-mean than the other method.
Key-Words / Index Term
Semantic image segmentation, Contextual Hierarchical Model, Logistic Disjunctive Normal Networks, ontology-based contextual knowledge representation, fuzzification
References
[1] H. F. Ates, S. Sunteci, “Multi-hypothesis contextual modeling for semantic segmentation”, Pattern Recognition, Vol.117, pp.104-110, 2019.
[2] X. Liu, Z. Deng, Y. Yang, “Recent progress in semantic image segmentation”, Artificial Intelligence Review, pp.1-18, 2018.
[3] P. Umorya, R. Singh, “A comparative based review on image segmentation of medical image and its technique”, International Journal of Scientific Research in Computer Science and Engineering, Vol.5, Issue.2, pp.71-76, 2017.
[4] N. Ghuse, Y. Deore, A. Potgantwar, “Efficient image processing based liver cancer detection method”, International Journal of Scientific Research in Network Security and Communication, Vol.5, Issue.3, pp.33-38, 2017.
[5] Z. Tu, X. Bai, “Auto-context and its application to high-level vision tasks and 3d brain image segmentation”, IEEE transactions on pattern analysis and machine intelligence, Vol.32, Issue.10, pp.1744-1757, 2010.
[6] C. Li, A. Kowdle, A. Saxena, T. Chen, “Toward holistic scene understanding: Feedback enabled cascaded classification models”, IEEE transactions on pattern analysis and machine intelligence, Vol.34, Issue.7, pp.1394-1408, 2012.
[7] M. Seyedhosseini, T. Tasdizen, “Semantic image segmentation with contextual hierarchical models”, IEEE transactions on pattern analysis and machine intelligence, Vol.38, Issue.5, pp.951-964, 2016.
[8] T. Sreedhar, S. Sathappan, “Different Conditional Random Field based Contextual Hierarchical Model for Semantic Image Segmentation”, Journal of Advanced Research in Dynamical and Control Systems, Vol.11, Issue.1, pp.48-57, 2019.
[9] M. Seyedhosseini, M. Sajjadi, T. Tasdizen, “Image segmentation with cascaded hierarchical models and logistic disjunctive normal networks”, In the Proceedings of the IEEE International Conference on Computer Vision, pp.2168-2175, 2013.
[10] T. Sreedar, S. Sathappan, “An Improved Optimized Logistic Distinctive Classifier for Efficient Semantic Image Segmentation”, International Conference on IoT, Social, Mobile, Analytics and Cloud in Computational Vision and Bio-Engineering Lecture Notes in Computational Vision and Biomechanics”, Communicated, 2019.
[11] N. Singh, S.B. Singh, “A modified mean Gray Wolf optimization approach for benchmark and biomedical problems”, Evolutionary Bioinformatics, Vol.13, pp.1-27, 2017.
[12] D. Zomahoun, “Collaborative Semantic Annotation of Images: Ontology-Based Model”, Signal & Image Processing: An International Journal (SIPIJ), Vol.4, Issue.6, pp.71-81, 2013.
[13] Z. Ren, G. Shakhnarovich, “Image segmentation by cascaded region agglomeration”, In 2013 IEEE conference on Computer vision and pattern recognition (CVPR), pp.2011-2018, 2013.
[14] M. Seyedhosseini, T. Tasdizen, “Multi-class multi-scale series contextual model for image segmentation”, IEEE Transactions on Image Processing, Vol.22, Issue.11, pp.4486-4496, 2013.
[15] D. Pei, Z. Li, R. Ji, F. Sun, “Efficient semantic image segmentation with multi-class ranking prior”, Computer Vision and Image Understanding, Vol.120, pp.81-90, 2014.
[16] L. L. Wang, N.H. Yung, “Hybrid graphical model for semantic image segmentation”, Journal of Visual Communication and Image Representation, Vol.28, pp.83-96, 2015.
[17] M. Zand, S. Doraisamy, A.A. Halin, M.R. Mustaffa, “Ontology-based semantic image segmentation using mixture models and multiple CRFs”, IEEE Transactions on Image Processing, Vol.25, Issue.7, pp.3233-3248, 2016.
[18] K. Chen, K. Fu, M. Yan, X. Gao, X. Sun, X. Wei, “Semantic Segmentation of Aerial Images with Shuffling Convolutional Neural Networks”, IEEE Geoscience and Remote Sensing Letters, Vol.15, Issue.2, pp.173-177, 2018.
[19] J. Li, W. Speier, K. C. Ho, K. V. Sarma, A. Gertych, B.S. Knudsen, C.W. Arnold, “An EM-based semi-supervised deep learning approach for semantic segmentation of histopathological images from radical prostatectomies”, Computerized Medical Imaging and Graphics, Vol.69, pp.125-133, 2018.
[20] Y. Sun, X. Zhang, Q. Xin, J. Huang, “Developing a multi-filter convolutional neural network for semantic segmentation using high-resolution aerial imagery and LiDAR data”, ISPRS Journal of Photogrammetry and Remote Sensing, Vol.143, pp.3-14, 2018.
[21] A.B. Benitez, D. Zhong, S.F. Chang, J.R. Smith, “MPEG-7 MDS content description tools and applications”, In International Conference on Computer Analysis of Images and Patterns, Springer, Berlin, Heidelberg, pp.41-52, 2001.