Person Re-identification with feature Aggregation
Madhavi Dayaram Bhamare1 , Nilesh R. Wankhade2
Section:Research Paper, Product Type: Journal Paper
Volume-7 ,
Issue-6 , Page no. 466-469, Jun-2019
CrossRef-DOI: https://doi.org/10.26438/ijcse/v7i6.466469
Online published on Jun 30, 2019
Copyright © Madhavi Dayaram Bhamare, Nilesh R. Wankhade . This is an open access article distributed under the Creative Commons Attribution License, which permits unrestricted use, distribution, and reproduction in any medium, provided the original work is properly cited.
View this paper at Google Scholar | DPI Digital Library
How to Cite this Paper
- IEEE Citation
- MLA Citation
- APA Citation
- BibTex Citation
- RIS Citation
IEEE Citation
IEEE Style Citation: Madhavi Dayaram Bhamare, Nilesh R. Wankhade, “Person Re-identification with feature Aggregation,” International Journal of Computer Sciences and Engineering, Vol.7, Issue.6, pp.466-469, 2019.
MLA Citation
MLA Style Citation: Madhavi Dayaram Bhamare, Nilesh R. Wankhade "Person Re-identification with feature Aggregation." International Journal of Computer Sciences and Engineering 7.6 (2019): 466-469.
APA Citation
APA Style Citation: Madhavi Dayaram Bhamare, Nilesh R. Wankhade, (2019). Person Re-identification with feature Aggregation. International Journal of Computer Sciences and Engineering, 7(6), 466-469.
BibTex Citation
BibTex Style Citation:
@article{Bhamare_2019,
author = {Madhavi Dayaram Bhamare, Nilesh R. Wankhade},
title = {Person Re-identification with feature Aggregation},
journal = {International Journal of Computer Sciences and Engineering},
issue_date = {6 2019},
volume = {7},
Issue = {6},
month = {6},
year = {2019},
issn = {2347-2693},
pages = {466-469},
url = {https://www.ijcseonline.org/full_paper_view.php?paper_id=4573},
doi = {https://doi.org/10.26438/ijcse/v7i6.466469}
publisher = {IJCSE, Indore, INDIA},
}
RIS Citation
RIS Style Citation:
TY - JOUR
DO = {https://doi.org/10.26438/ijcse/v7i6.466469}
UR - https://www.ijcseonline.org/full_paper_view.php?paper_id=4573
TI - Person Re-identification with feature Aggregation
T2 - International Journal of Computer Sciences and Engineering
AU - Madhavi Dayaram Bhamare, Nilesh R. Wankhade
PY - 2019
DA - 2019/06/30
PB - IJCSE, Indore, INDIA
SP - 466-469
IS - 6
VL - 7
SN - 2347-2693
ER -
![]() |
![]() |
![]() |
272 | 333 downloads | 146 downloads |
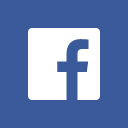
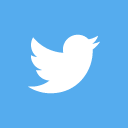
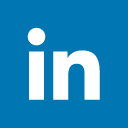
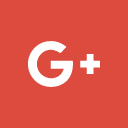
Abstract
Person Re-identification (re-ID) is a critical problem in video analytics applications such as security and surveillance. Although many approaches have been proposed, it remains a challenging problem since persons appearance usually undergoes dramatic changes across camera views due to changes in view angle, body pose and background clutter. Person re-id aims to retrieve a person of interest across spatially disjoint cameras. The system focuses on tackling the person re-ID problem with the proposed metric learning scheme. There is a discriminant metric learning strategy for this testing issue. Most existing metric learning algorithms, it takes both original data and auxiliary data during training which is motivated by the new machine learning paradigm - Learning Using Privileged Information. This system is based on features aggregation. Image dataset is load and the basic operation is performing that is to convert those load images into gray scale. And also create the HOG (Histogram of oriented gradient) descriptor, in this features extraction task completed based on EHD (Edge of histogram descriptor), CLD (Color Layout descriptor), and SCD (Scale Color descriptor). The system aggregates all Features and Generate Train metric. After that an unknown image is load which is comes through gray scale process and HOG descriptor. Classify that images and identify the correct image. Such system is used in many sectors for security purpose
Key-Words / Index Term
Person Re-identification, Metric Learning, Feature Aggregation, HOG descriptor
References
[1] Xun Yang, Meng Wang, Dacheng Tao, “Person Re-identification with Metric Learning using Privileged Information”, IEEE transactions on Image processing, 2017.
[2] L. Zheng, Y. Yang, and A. G. Hauptmann, “Person re-identification: Past, present and future”, arXiv preprint arXiv: 1610.02984, 2016.
[3] T. Matsukawa, T. Okabe, E. Suzuki, and Y. Sato, “Hierarchical Gaussian descriptor for person re-identification”, in IEEE Conference on Computer Vision and Pattern Recognition, pp. 13631372,2016.
[4] S. Liao, Y. Hu, X. Zhu, and S. Z. Li, “Person re-identification by local maximal occurrence representation and metric learning”, in IEEE conference on Computer Vision and Pattern Recognition, pp. 21972206, 2015.
[5] A. Mignon and F. Jurie, Pcca, “A new approach for distance learning from sparse pairwise constraints”, in IEEE Conference on Computer Vision and Pattern Recognition, pp. 26662672, 2012.
[6] S. Liao and S. Z. Li, “Efficient psd constrained asymmetric metric learning for person re-identification”, in IEEE International Conference on Computer vision, pp. 36853693, 2015.
[7] L. Ma, X. Yang, and D. Tao, “Person re-identification over camera networks using multi-task distance metric learning”, IEEE Transactions on Image Processing.
[8] L. Zhang, T. Xiang, and S. Gong, “Learning a discriminative null space for person re-identification”, in IEEE Conference on Computer Vision and Pattern Recognition, 2016.
[9] M. Kstinger, M. Hirzer, P. Wohlhart, P. M. Roth, and H. Bischof, “Large scale metric learning from equivalence constraints”, in IEEE Conference on Computer Vision and Pattern Recognition, pp. 22882295, 2012.
[10] S. Paisitkriangkrai, C. Shen, and A. van den Hengel, “Learning to rank in person re-identification with metric ensembles”, in IEEE Conference on Computer Vision and Pattern Recognition, pp. 18461855, 2015.
[11] Z. Li, S. Chang, F. Liang, T. S. Huang, L. Cao, and J. R. Smith, “Learning locally-adaptive decision functions for person verification”, in IEEE Conference on Computer Vision and Pattern Recognition, pp. 36103617, 2013.
[12] Xiong, M. Gou, O. Camps, and M. Sznaier, “Person re-identification using kernel-based metric learning methods”, in European Conference on Computer Vision, pp. 116,2014.
[13] E. P. Xing, M. I. Jordan, S. Russell, and A. Y. Ng, “Distance metric learning with application to clustering with side-information”, in NIPS, pp. 505–512, 2002.
[14] A. Globerson and S. T. Roweis, “Metric learning by collapsing classes”, in NIPS, pp. 451–458, 2005.
[15] M. Schultz and T. Joachims, “Learning a distance metric from relative comparisons,” in NIPS, 2004.
[16] J. V. Davis, B. Kulis, P. Jain, S. Sra, and I. S. Dhillon, “Information theoretic metric learning,” in ICML, pp. 209–216, 2007.
[17] C. Jose and F. Fleuret, “Scalable metric learning via weighted
Approximate rank component analysis”, in European Conference
on Computer Vision. Springer, pp. 875–890, 2016.
[18] H. Liu, J. Feng, M. Qi, J. Jiang, and S. Yan, “End-to-end
Comparative attention networks for person re-identification”,
IEEE Transactions on Image Processing, 2017.