Context-Aware Local Binary Feature Learning : An Approach For Face Recognition
Sushmitamai K. Ahire1 , Nilesh R. Wankhade2
Section:Research Paper, Product Type: Journal Paper
Volume-7 ,
Issue-6 , Page no. 462-465, Jun-2019
CrossRef-DOI: https://doi.org/10.26438/ijcse/v7i6.462465
Online published on Jun 30, 2019
Copyright © Sushmitamai K. Ahire, Nilesh R. Wankhade . This is an open access article distributed under the Creative Commons Attribution License, which permits unrestricted use, distribution, and reproduction in any medium, provided the original work is properly cited.
View this paper at Google Scholar | DPI Digital Library
How to Cite this Paper
- IEEE Citation
- MLA Citation
- APA Citation
- BibTex Citation
- RIS Citation
IEEE Style Citation: Sushmitamai K. Ahire, Nilesh R. Wankhade, “Context-Aware Local Binary Feature Learning : An Approach For Face Recognition,” International Journal of Computer Sciences and Engineering, Vol.7, Issue.6, pp.462-465, 2019.
MLA Style Citation: Sushmitamai K. Ahire, Nilesh R. Wankhade "Context-Aware Local Binary Feature Learning : An Approach For Face Recognition." International Journal of Computer Sciences and Engineering 7.6 (2019): 462-465.
APA Style Citation: Sushmitamai K. Ahire, Nilesh R. Wankhade, (2019). Context-Aware Local Binary Feature Learning : An Approach For Face Recognition. International Journal of Computer Sciences and Engineering, 7(6), 462-465.
BibTex Style Citation:
@article{Ahire_2019,
author = {Sushmitamai K. Ahire, Nilesh R. Wankhade},
title = {Context-Aware Local Binary Feature Learning : An Approach For Face Recognition},
journal = {International Journal of Computer Sciences and Engineering},
issue_date = {6 2019},
volume = {7},
Issue = {6},
month = {6},
year = {2019},
issn = {2347-2693},
pages = {462-465},
url = {https://www.ijcseonline.org/full_paper_view.php?paper_id=4572},
doi = {https://doi.org/10.26438/ijcse/v7i6.462465}
publisher = {IJCSE, Indore, INDIA},
}
RIS Style Citation:
TY - JOUR
DO = {https://doi.org/10.26438/ijcse/v7i6.462465}
UR - https://www.ijcseonline.org/full_paper_view.php?paper_id=4572
TI - Context-Aware Local Binary Feature Learning : An Approach For Face Recognition
T2 - International Journal of Computer Sciences and Engineering
AU - Sushmitamai K. Ahire, Nilesh R. Wankhade
PY - 2019
DA - 2019/06/30
PB - IJCSE, Indore, INDIA
SP - 462-465
IS - 6
VL - 7
SN - 2347-2693
ER -
![]() |
![]() |
![]() |
371 | 194 downloads | 133 downloads |
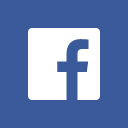
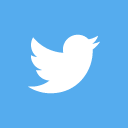
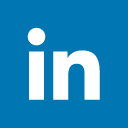
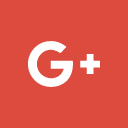
Abstract
This system uses Context-Aware Local Binary Feature Learning (CA-LBFL) Method for face recognition. Learning based methods such as DFD and CBFD learn features representation from raw pixel and they are more susceptible to noise where existing local feature descriptors are hand crafted and they require strong prior knowledge and heuristic. Proposed system uses contextual information for face recognition because context provides strong prior knowledge. It helps to enhance the robustness and stableness of various visual analysis tasks. to jointly learn multiple projections matrices for mapping we make use of context-aware local binary multi-scale feature learning (CA-LBMFL), where each projection matrix corresponds to a specific scale of pixel difference vector (PDV). PDVs are extracted from image and stored in a text file in the binary form. Face recognition is performed on the basis of this extracted features. For heterogeneous face matching we implement coupled learning methods based on CA-LBFL and CA-LBMFL. Experimental result is based on two widely used datasets LWF and YTF.
Key-Words / Index Term
Face representation,Face recognition,Heterogenous face,Context-Aware,Binary feature learning
References
[1] Yueqi Duan, Jianjiang Feng, Jie Zhou “Context-Aware Local Binary Feature Learning for Face Recognition”, IEEE Transaction Pattern Analysis and Machine Intelligence, DOI 10.1109 / TPAMI. 2017.2710183
[2] T. Ahonen, A. Hadid, and M. Pietikainen. Face description with local binary patterns: Application to face recognition. TPAMI, 28(12):2037–2041, 2006.
[3] binary patterns: Application to face recognition. TPAMI, 28(12):2037– 2041, 2006.
[4] Y.Bengio,P.Lamblin,D.Popovici and H.Larochelle.Greedy Layerwise training of deep networks,In NIPS
[5] E.Meyers and L.Wolf .using biologically inspired feature for face processing.IJCV,76(1):93-104,2008.
[6] P. N. Belhumeur, J. P. Hespanha, and D. J. Kriegman. Eigenfaces vs.
Fisherfaces: Recognition using class specific linear projection. TPAMI, 19(7):711–720, 1997.
[7] Z. Lei, S. Z. Li, R. Chu, and X. Zhu. Face recognition with local Gabor textons. In ICB, pages 49–57. 2007.
[8] Q. V. Le, A. Karpenko, J. Ngiam, and A. Y. Ng. ICA with reconstruction cost for efficient overcomplete feature learning. In NIPS, pages 1017– 1025, 2011.
[9] Z. Cao, Q. Yin, X. Tang, and J. Sun. Face recognition with learning based descriptor. In CVPR, pages 2707–2714,2010.
[10] X. Cao, D. Wipf, F. Wen, G. Duan, and J. Sun. A practical transfer
learning algorithm for face verification. In ICCV, pages 3208–
3215,2013.
[11] C. Liu and H. Wechsler. Gabor feature based classification using the enhanced Fisher linear discriminant model for face recognition.TIP, 11(4):467–476, 2002.
[12] J. Lu, V. E. Liong, and J. Zhou. Simultaneous local binary feature learning and encoding for face recognition. In ICCV, pages 3721– 3729,2015
[13] D. Lin and X. Tang. Inter-modality face recognition. In ECCV,pages 13–26. 2006.
[14] A. Mignon and F. Jurie. CMML: a new metric learning approach for cross modal matching.In ACCV,pages 14–27, 2012.
[15] O. M. Parkhi, A. Vedaldi, and A. Zisserman. Deep face recognition.in BMVC, volume 1, pages 1–12, 2015.
[16] ] H. K. Galoogahi and T. Sim. Inter-modality face sketch recognitionin ICME , pages 224–229, 2012.
[17] X. Gao, J. Zhong, J. Li, and C. Tian. Face sketch synthesis algorithm based on E-HMM and selective ensemble. TCSVT, 18(4):487–496, 2008
[18] M. Turk and A. Pentland. Eigenfaces for recognition.Journal of CognitiveNeuroscience,3(1):71–86,1991.
[19] Z. Lei, M. Pietikainen, and S. Z. Li. Learning discriminant face descriptor. TPAMI, 36(2):289–302, 2013.
[20] G. E. Hinton, S. Osindero, and Y.-W. Teh. A fast learning algorithm for deep belief nets. Neural Computation, 18(7):1527–1554, 2006.
[21] D. G. Lowe. Distinctive image features from scale-invariant keypoints.IJCV, 60(2):91–110, 2004.
[22] H. Bay, T. Tuytelaars, and L. Van Gool. SURF: Speeded up robustfeatures. In ECCV, pages 404–417, 2006.
[23] G. Sharma, S. ul Hussain, and F. Jurie. Local higher-order statistics (LHS) for texture categorization and facial analysis. In ECCV, pages 1-12-2012.
[24] G. B. Huang, H. Lee, and E. Learned-Miller. Learning hierarchical representations for face verification with convolutional deep belief network. In CVPR, pages 2518–2525, 2012.
[25] S. Rifai, P. Vincent, X. Muller, X. Glorot, and Y. Bengio. Contractive auto-encoders : Explicit invariance during feature extraction. In ICML,pages 833–840, 2011.
[26] E. Rublee, V. Rabaud, K. Konolige, and G. Bradski. ORB: An efficient alternative to SIFT or SURF. In ICCV, pages 2564–2571, 2011.
[27] G. Huang, M. Ramesh, T. Berg, and E. Learned-Miller.Labeled faces in the wild: A database for studying face recognition in unconstrained environment . Technical report, 07-49, UMass Amherst, 2007.
[28] L. Wolf, T. Hassner, and I. Maoz. Face recognition in unconstrained video with matched background similarity. In CVPR, pages 529–534,201
[29] J. Lu, V. E. Liong, Z. Xiuzhuang, and J. Zhou. Learning compact binary face descriptor for face recognition. TPAMI, pages 2041–2056, 2015.
[30] M. Turk and A. Pentland. Eigenfaces for recognition. Journal of
Cognitive Neuroscience, 3(1):71–86, 1991.
[31] G.Sowmiya , V. Kumutha.Facial Expression Recognition Using Static Facial Image. Isroset-Journal Vol.6 , Issue.2 , pp.72-75, Apr-2018
[32] Takrim Ul Islam Laskar , Parismita Sarma.Facial Landmark Detection for Expression Analysis .IJCSE Journal,Volume-7 , Issue-5 , Page no. 1617-1622, May-2019.