Proposed Model for Emotions Based Recommender Systems Using Reviews
Veepu Uppal1 , Rajesh Kumar Singh2
Section:Review Paper, Product Type: Journal Paper
Volume-7 ,
Issue-6 , Page no. 401-405, Jun-2019
CrossRef-DOI: https://doi.org/10.26438/ijcse/v7i6.401405
Online published on Jun 30, 2019
Copyright © Veepu Uppal, Rajesh Kumar Singh . This is an open access article distributed under the Creative Commons Attribution License, which permits unrestricted use, distribution, and reproduction in any medium, provided the original work is properly cited.
View this paper at Google Scholar | DPI Digital Library
How to Cite this Paper
- IEEE Citation
- MLA Citation
- APA Citation
- BibTex Citation
- RIS Citation
IEEE Citation
IEEE Style Citation: Veepu Uppal, Rajesh Kumar Singh, “Proposed Model for Emotions Based Recommender Systems Using Reviews,” International Journal of Computer Sciences and Engineering, Vol.7, Issue.6, pp.401-405, 2019.
MLA Citation
MLA Style Citation: Veepu Uppal, Rajesh Kumar Singh "Proposed Model for Emotions Based Recommender Systems Using Reviews." International Journal of Computer Sciences and Engineering 7.6 (2019): 401-405.
APA Citation
APA Style Citation: Veepu Uppal, Rajesh Kumar Singh, (2019). Proposed Model for Emotions Based Recommender Systems Using Reviews. International Journal of Computer Sciences and Engineering, 7(6), 401-405.
BibTex Citation
BibTex Style Citation:
@article{Uppal_2019,
author = {Veepu Uppal, Rajesh Kumar Singh},
title = {Proposed Model for Emotions Based Recommender Systems Using Reviews},
journal = {International Journal of Computer Sciences and Engineering},
issue_date = {6 2019},
volume = {7},
Issue = {6},
month = {6},
year = {2019},
issn = {2347-2693},
pages = {401-405},
url = {https://www.ijcseonline.org/full_paper_view.php?paper_id=4564},
doi = {https://doi.org/10.26438/ijcse/v7i6.401405}
publisher = {IJCSE, Indore, INDIA},
}
RIS Citation
RIS Style Citation:
TY - JOUR
DO = {https://doi.org/10.26438/ijcse/v7i6.401405}
UR - https://www.ijcseonline.org/full_paper_view.php?paper_id=4564
TI - Proposed Model for Emotions Based Recommender Systems Using Reviews
T2 - International Journal of Computer Sciences and Engineering
AU - Veepu Uppal, Rajesh Kumar Singh
PY - 2019
DA - 2019/06/30
PB - IJCSE, Indore, INDIA
SP - 401-405
IS - 6
VL - 7
SN - 2347-2693
ER -
![]() |
![]() |
![]() |
395 | 299 downloads | 155 downloads |
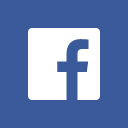
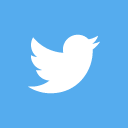
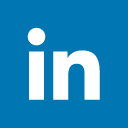
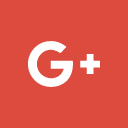
Abstract
Information Analysis and extraction is difficult due to huge amount of data on the Internet. Recommender Systems provide efficient and useful information for user according to their preferences. Large numbers of research have been accomplished on Emotion based Recommender systems Techniques. These techniques extract the human emotions for any items from reviews. In this paper we summarize the existing techniques to extract emotions from reviews written by users for different items and propose a new method to design a dynamic search engine which will extract the online reviews and recommend items of different category on the basis of user search. Further our proposed technique will recommend items to user by combination of online reviews and ratings of product too. The spam reviews will be identified and removed.
Key-Words / Index Term
Recommender system, emotions, collaborative, content based reviews
References
[1]F.O.IsinkayeY.O.FolajimiandB.A.Ojokoh“Recommendation systems: Principles, methods and evaluation”,Egyptian Informatics Journal,Volume 16, Issue 3, November 2015, pp: 261-273
[2] Badrul Sarwar, George Karypis, Joseph Konstan, and John Riedl “Item-Based Collaborative Filtering Recommendation Algorithms”Proceedings:10th international conference on World Wide Web, Pages 285-295 Hong Kong, Hong Kong — May 01 - 05, 2001 ACM New York, NY.
[3] Micheal j.Pazzami and Daniel Bilsus “Content based Recommendation System”Book The adaptive web Springer-Verleg Berlin, Heidlbergpp: 325-341. ISBN:978-3-540-72078-2,2007.
[4] Simon Philio and P.B Shola “A Paper Reccomender System Based on the Past Rating of the User”International journal of Advanced Computer Technology, Volume 3,Issue 6,pp 40-46, Dec 2015.
[5] Dhoha Almazro, Ghadeer Shahatah, Lamia Albdulkarim, Mona Kherees, Romy Martinez and William Nzoukou “A Survey Paper on Recommender Systems”Published 2010 in ArXiv.
[6] R. W. Picard, “Affective computing: challenges” International Journal of Human-Computer Studies, Vol. 59, No. 1, pp. 55--64, 2003.
[7] Russel JA (1980) A circumplex model of affect. J Pers Soc Psychol 39:1161–1178. doi:10.1037/h0077714
[8] Miller GA (1995) WordNet: a lexical database for English. Commun ACM 38(11):39–41
[9] Kumar A, Kumar N, Hussain M, et al “Semantic clustering-based cross-Domain recommendation” Proc Computational Intelligence and Data Mining (CIDM): pp: 137–141, 2014.
[10] SaraswatM, Chakraverty S,Mahajan N, Tokas N (2016) “On using reviews and comments for cross domain recommendations and decision making”. In: Computing for Sustainable Global Development (INDIACom), 2016 3rd International Conference on IEEE, p 3656–3659
[11] Winoto P and Tang TY“The role of user mood in movie recommendations”Expert Systems with Applications,Elsevier Volume 37, Issue 8, August 2010, Pages 6086-6092
[12] Peng Li,Seiji Yamada “A Movie Recommender System based on Inductive Learning” IEEE Conference on Cybernetics and Intelligent Systems Singapore, 1-3 December, 2004.
[13] Shi Y, Larson M and Hanjalic A“Mining mood-specific movie similarity with matrix factorization forcontext-aware recommendation” Proc of the workshop on context-aware movie recommendation, pp: 34–40, 2010.
[14] Peleja, Filipa, et al. "A recommender system for the TV on the web: integrating unrated reviews and movie ratings" Multimedia systems 19.6 (2013): 543-558.
[15] Chen, Guanliang, and Li Chen. "Augmenting service recommender systems by incorporating contextual opinions from user reviews" User Modeling and User-Adapted Interaction 25.3 (2015): 295-329
[16] Sun, Lihua, Junpeng Guo, and Yanlin Zhu. "Applying uncertainty theory into the restaurant recommender system based on sentiment analysis of online Chinese reviews." World Wide Web (2018): 1-18.
[17] Zhijun Yan, Meiming Xing, Dongsong Zhang,Baizhang Ma (2015) “Exprs: An Extended Page rank method for product feature extraction from online consumer reviews” Information & Management 52.7 (2015): 850-858.
[18] Sulthana, A. Razia, and Subburaj Ramasamy. "Ontology and context based recommendation system using Neuro-Fuzzy Classification" Computers & Electrical Engineering (2018).