Human Activity Classification for Surveillance using Machine Learning and Image Processing Algorithm
Arvind Malge1 , Mallikarjuna Shastry P.M2
Section:Research Paper, Product Type: Journal Paper
Volume-7 ,
Issue-6 , Page no. 343-346, Jun-2019
CrossRef-DOI: https://doi.org/10.26438/ijcse/v7i6.343346
Online published on Jun 30, 2019
Copyright © Arvind Malge, Mallikarjuna Shastry P.M . This is an open access article distributed under the Creative Commons Attribution License, which permits unrestricted use, distribution, and reproduction in any medium, provided the original work is properly cited.
View this paper at Google Scholar | DPI Digital Library
How to Cite this Paper
- IEEE Citation
- MLA Citation
- APA Citation
- BibTex Citation
- RIS Citation
IEEE Style Citation: Arvind Malge, Mallikarjuna Shastry P.M, “Human Activity Classification for Surveillance using Machine Learning and Image Processing Algorithm,” International Journal of Computer Sciences and Engineering, Vol.7, Issue.6, pp.343-346, 2019.
MLA Style Citation: Arvind Malge, Mallikarjuna Shastry P.M "Human Activity Classification for Surveillance using Machine Learning and Image Processing Algorithm." International Journal of Computer Sciences and Engineering 7.6 (2019): 343-346.
APA Style Citation: Arvind Malge, Mallikarjuna Shastry P.M, (2019). Human Activity Classification for Surveillance using Machine Learning and Image Processing Algorithm. International Journal of Computer Sciences and Engineering, 7(6), 343-346.
BibTex Style Citation:
@article{Malge_2019,
author = {Arvind Malge, Mallikarjuna Shastry P.M},
title = {Human Activity Classification for Surveillance using Machine Learning and Image Processing Algorithm},
journal = {International Journal of Computer Sciences and Engineering},
issue_date = {6 2019},
volume = {7},
Issue = {6},
month = {6},
year = {2019},
issn = {2347-2693},
pages = {343-346},
url = {https://www.ijcseonline.org/full_paper_view.php?paper_id=4555},
doi = {https://doi.org/10.26438/ijcse/v7i6.343346}
publisher = {IJCSE, Indore, INDIA},
}
RIS Style Citation:
TY - JOUR
DO = {https://doi.org/10.26438/ijcse/v7i6.343346}
UR - https://www.ijcseonline.org/full_paper_view.php?paper_id=4555
TI - Human Activity Classification for Surveillance using Machine Learning and Image Processing Algorithm
T2 - International Journal of Computer Sciences and Engineering
AU - Arvind Malge, Mallikarjuna Shastry P.M
PY - 2019
DA - 2019/06/30
PB - IJCSE, Indore, INDIA
SP - 343-346
IS - 6
VL - 7
SN - 2347-2693
ER -
![]() |
![]() |
![]() |
422 | 427 downloads | 205 downloads |
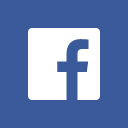
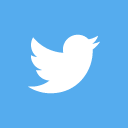
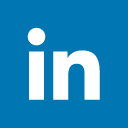
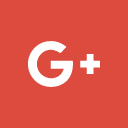
Abstract
Today`s world surveillance system plays a major role in the security industry. In the video monitoring system, moving object detection was frequently used. Motion estimation is also an important part of video processing monitoring, such as video filtering and compression of video frames. Video Surveillance System is a powerful tool for tracking people and their public safety operations. The reason for having a monitoring system is not only to place cameras in the human eye place, but also to allow them to automatically acknowledge activities. This paper creates a smart recognition of the system of human activity. At each stage of the suggested system, image processing techniques are used A system was built based on the Caltech database of human activity features acquired from frame sequences. Relevance Vector classifier used in the dataset to classify the model of activity. Classification results show high effectiveness throughout the training, testing and validation stages.
Key-Words / Index Term
Human activity recognition, relevance vector classifier, histogram of gradients, Background subtraction
References
[1] Hossen, Muhammad Kamal, and Sabrina Hoque Tuli. "A surveillance system based on motion detection and motion estimation using optical flow." In Informatics, Electronics and Vision (ICIEV), 2016 5th International Conference on, pp. 646-651. IEEE, 2016.
[2] Htike, Kyaw Kyaw, Othman O. Khalifa, Huda Adibah Mohd Ramli, and Mohammad AM Abushariah. "Human activity recognition for video surveillance using sequences of postures." In e-Technologies and Networks for Development (ICeND), 2014 Third International Conference on, pp. 79-82. IEEE, 2014.
[3] M. Thida, Y. L. Yong, P. Climent-P´erez, H.-l. Eng, and P. Remagnino, “A literature review on video analytics of crowded scenes,” in Intelligent Multimedia Surveillance. Springer, 2013, pp. 17–36..
[4] Kaur, Rajvir, and Sonit Singh. "Background modelling, detection and tracking of human in video surveillance system." In Computational Intelligence on Power, Energy and Controls with their impact on Humanity (CIPECH), 2014 Innovative Applications of, pp. 54-58. IEEE, 2014.
[5] Meng, Binghao, Lu Zhang, Fan Jin, Lu Yang, Hong Cheng, and Qian Wang. "Abnormal Events Detection Using Deep Networks for Video Surveillance." In International Conference on Cognitive Systems and Signal Processing, pp. 197-204. Springer, Singapore, 2016.
[6] Peng, Qiwei, Gongyi Hong, Min Feng, Yuan Xia, Lei Yu, Xu Wang2and, and Yang Li. "Off-position detection based on convolutional neural network." (2016).
[7] Sapana, Miss, K Mishra, and K S Bhagat. 2015. “A Survey on Human Motion Detection and Surveillance.” International Journal of Advanced Research in Electronics and Communication Engineering 4 (4).
[8] Automated Daily Human Activity Recognition for Video Surveillance Using Neural Network Mohanad Babiker1, Othman O. khalifa1, Kyaw Kyaw Htike2, Aisha Hassan1, Muhamed Zaharadeen1 1Department of Electrical and Computer Engineering International Islamic University Malaysia 2Department of Information Technology UCSI University, Malaysia
[9] An Approach of Understanding Human Activity Recognition and Detection for Video Surveillance using HOG Descriptor and SVM Classifier Jagadesh B Assistant Professor, Department of ECE VVCE, Mysuru jagadeesh.b@vvce.ac.in Meghana M N Department of ECE VVCE, Mysuru.