A Comparative Analysis of Different Machine Learning Classification Algorithms for Predicting Chronic Kidney Disease
Bhawna Sharma1 , Sheetal Gandotra2 , Utkarsh Sharma3 , Rahul Thakur4 , Alankar Mahajan5
Section:Research Paper, Product Type: Journal Paper
Volume-7 ,
Issue-6 , Page no. 8-13, Jun-2019
CrossRef-DOI: https://doi.org/10.26438/ijcse/v7i6.813
Online published on Jun 30, 2019
Copyright © Bhawna Sharma, Sheetal Gandotra, Utkarsh Sharma, Rahul Thakur, Alankar Mahajan . This is an open access article distributed under the Creative Commons Attribution License, which permits unrestricted use, distribution, and reproduction in any medium, provided the original work is properly cited.
View this paper at Google Scholar | DPI Digital Library
How to Cite this Paper
- IEEE Citation
- MLA Citation
- APA Citation
- BibTex Citation
- RIS Citation
IEEE Style Citation: Bhawna Sharma, Sheetal Gandotra, Utkarsh Sharma, Rahul Thakur, Alankar Mahajan, “A Comparative Analysis of Different Machine Learning Classification Algorithms for Predicting Chronic Kidney Disease,” International Journal of Computer Sciences and Engineering, Vol.7, Issue.6, pp.8-13, 2019.
MLA Style Citation: Bhawna Sharma, Sheetal Gandotra, Utkarsh Sharma, Rahul Thakur, Alankar Mahajan "A Comparative Analysis of Different Machine Learning Classification Algorithms for Predicting Chronic Kidney Disease." International Journal of Computer Sciences and Engineering 7.6 (2019): 8-13.
APA Style Citation: Bhawna Sharma, Sheetal Gandotra, Utkarsh Sharma, Rahul Thakur, Alankar Mahajan, (2019). A Comparative Analysis of Different Machine Learning Classification Algorithms for Predicting Chronic Kidney Disease. International Journal of Computer Sciences and Engineering, 7(6), 8-13.
BibTex Style Citation:
@article{Sharma_2019,
author = {Bhawna Sharma, Sheetal Gandotra, Utkarsh Sharma, Rahul Thakur, Alankar Mahajan},
title = {A Comparative Analysis of Different Machine Learning Classification Algorithms for Predicting Chronic Kidney Disease},
journal = {International Journal of Computer Sciences and Engineering},
issue_date = {6 2019},
volume = {7},
Issue = {6},
month = {6},
year = {2019},
issn = {2347-2693},
pages = {8-13},
url = {https://www.ijcseonline.org/full_paper_view.php?paper_id=4500},
doi = {https://doi.org/10.26438/ijcse/v7i6.813}
publisher = {IJCSE, Indore, INDIA},
}
RIS Style Citation:
TY - JOUR
DO = {https://doi.org/10.26438/ijcse/v7i6.813}
UR - https://www.ijcseonline.org/full_paper_view.php?paper_id=4500
TI - A Comparative Analysis of Different Machine Learning Classification Algorithms for Predicting Chronic Kidney Disease
T2 - International Journal of Computer Sciences and Engineering
AU - Bhawna Sharma, Sheetal Gandotra, Utkarsh Sharma, Rahul Thakur, Alankar Mahajan
PY - 2019
DA - 2019/06/30
PB - IJCSE, Indore, INDIA
SP - 8-13
IS - 6
VL - 7
SN - 2347-2693
ER -
![]() |
![]() |
![]() |
1559 | 739 downloads | 243 downloads |
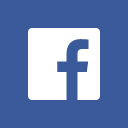
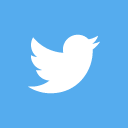
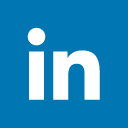
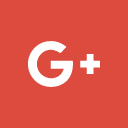
Abstract
Chronic kidney disease (CKD) is a condition characterized by a gradual loss of kidney function over time. It includes risk of cardiovascular disease and end-stage renal disease. In this paper, we use Machine Learning approach for predicting CKD. In this paper, we present a comparative analysis of seven different machine learning algorithms. This study starts with twenty-four parameters in addition to the class attribute and twenty five percent of the data set is used to test the predictions. Algorithms are trained using fivefold cross-validation and performance of the system is assessed using classification accuracy, confusion matrix, specificity and sensitivity.
Key-Words / Index Term
CKD, Machine Learning, Logistic Regression, Support Vector Machine, Random Forest
References
[1] Chaitanya Gupte and Shruti Gadewar, “Diagnosis of Parkinson’s Disease using Acoustic Analysis of Voice”, International Journal of Scientific Research in Network Security and Communication, Vol.5, Issue.3, pp.14-18, 2017.
[2] Pallvi Dehariya, “An Artificial Immune System and Neural Network to Improve the Detection Rate in Intrusion Detection System”, International Journal of Scientific Research in Network Security and Communication, Vol.4, Issue.1, pp.1-4, 2016.
[3] Antje Erler, Martin Beyer, Juliana J. Petersen, Kristina Saal, Thomas Rath, Justine Rochon, Walter E. Haefeli and Ferdinand M. Gerlach, “How to improve drug dosing for patients with renal impairment in primary care – a cluster-randomized controlled trial”, BMC Family Practice, Vol.13, Issue.1, Article.91, pp.1-8, 2012.
[4] S. Venkata Lakshmi, M. K. Meena and N. S. Kiruthika, “Diagnosis of Chronic Kidney Disease using Random Forest Algorithms”, International Journal of Research in Engineering, Science and Management, Vol.2, Issue.3, pp.559-562, 2019.
[5] R. Xi, N. Lin and Y. Chen, “Compression and Aggregation for Logistic Regression Analysis in Data Cubes”, IEEE Transactions on Knowledge and Data Engineering, Vol.21, Issue.4, pp.479-492, 2009.
[6] R. G. Brereton, and G. R. Lloyd, “Support Vector Machines for classification and regression”, Analyst, Vol.135, Issue.2, pp.230-267, 2010.
[7] Galit Shmueli, Nitin R. Patel and Peter C. Bruce, “Data Mining for Business Intelligence: Concepts, Techniques, and Applications in Microsoft Office Excel with XLMiner”, Wiley Publishing, pp.250-268, 2010.
[8] Afzal Ahmad, Mohammad Asif and Shaikh Rohan Ali, “Review Paper on Shallow Learning and Deep Learning Methods for Network Security”, International Journal of Scientific Research in Computer Science and Engineering, Vol.6, Issue.5, pp.45-54, 2018.
[9] J. Ross Quinlan, “C4.5: Programs for Machine Learning by J. Ross Quinlan.”, Morgan Kaufmann Publishers, Inc., pp.17-26, 1993.
[10] Deepika Mallampati, “An Efficient Spam Filtering using Supervised Machine Learning Techniques”, International Journal of Scientific Research in Computer Science and Engineering, Vol.6, Issue.2, pp.33-37, 2018.
[11] M. S. Anbarasi and V. Janani, “Ensemble classifier with Random Forest algorithm to deal with imbalanced healthcare data”, In International Conference on Information Communication and Embedded Systems (ICICES), Chennai, India, pp.1–7, 2017.
[12] Hanyu Zhang, Che-Lun Hung, William Cheng-Chung Chu, Ping-Fang Chiu and Chuan Yi Tang, “Chronic Kidney Disease Survival Prediction with Artificial Neural Networks”, In IEEE International Conference on Bioinformatics and Biomedicine (BIBM), Madrid, Spain, pp.1-6, 2018.