Prediction of Diabetes with a BPNN-NB ensemble classifier
Issac P. J.1 , Allam Appa Rao2
Section:Research Paper, Product Type: Journal Paper
Volume-7 ,
Issue-5 , Page no. 1652-1657, May-2019
CrossRef-DOI: https://doi.org/10.26438/ijcse/v7i5.16521657
Online published on May 31, 2019
Copyright © Issac P. J., Allam Appa Rao . This is an open access article distributed under the Creative Commons Attribution License, which permits unrestricted use, distribution, and reproduction in any medium, provided the original work is properly cited.
View this paper at Google Scholar | DPI Digital Library
How to Cite this Paper
- IEEE Citation
- MLA Citation
- APA Citation
- BibTex Citation
- RIS Citation
IEEE Citation
IEEE Style Citation: Issac P. J., Allam Appa Rao, “Prediction of Diabetes with a BPNN-NB ensemble classifier,” International Journal of Computer Sciences and Engineering, Vol.7, Issue.5, pp.1652-1657, 2019.
MLA Citation
MLA Style Citation: Issac P. J., Allam Appa Rao "Prediction of Diabetes with a BPNN-NB ensemble classifier." International Journal of Computer Sciences and Engineering 7.5 (2019): 1652-1657.
APA Citation
APA Style Citation: Issac P. J., Allam Appa Rao, (2019). Prediction of Diabetes with a BPNN-NB ensemble classifier. International Journal of Computer Sciences and Engineering, 7(5), 1652-1657.
BibTex Citation
BibTex Style Citation:
@article{J._2019,
author = {Issac P. J., Allam Appa Rao},
title = {Prediction of Diabetes with a BPNN-NB ensemble classifier},
journal = {International Journal of Computer Sciences and Engineering},
issue_date = {5 2019},
volume = {7},
Issue = {5},
month = {5},
year = {2019},
issn = {2347-2693},
pages = {1652-1657},
url = {https://www.ijcseonline.org/full_paper_view.php?paper_id=4466},
doi = {https://doi.org/10.26438/ijcse/v7i5.16521657}
publisher = {IJCSE, Indore, INDIA},
}
RIS Citation
RIS Style Citation:
TY - JOUR
DO = {https://doi.org/10.26438/ijcse/v7i5.16521657}
UR - https://www.ijcseonline.org/full_paper_view.php?paper_id=4466
TI - Prediction of Diabetes with a BPNN-NB ensemble classifier
T2 - International Journal of Computer Sciences and Engineering
AU - Issac P. J., Allam Appa Rao
PY - 2019
DA - 2019/05/31
PB - IJCSE, Indore, INDIA
SP - 1652-1657
IS - 5
VL - 7
SN - 2347-2693
ER -
![]() |
![]() |
![]() |
456 | 277 downloads | 204 downloads |
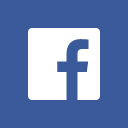
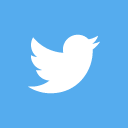
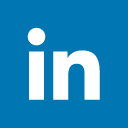
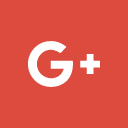
Abstract
Disease prediction techniques play a major role in the recent times since it is crucial to predict the risks of a disease in advance for leading a healthier life. Diabetes is one of the diseases that affect lots of people. Since it is increasing rapidly, more and more people are being affected by diabetes based diseases like Diabetes Nephropathy (DN) and Diabetes Mellitus (DM). Most people suffering from diabetes do not know a lot about their health quality or the risk factors faced until they get diagnosed with the disease. This disease is a major cause of renal failure, blindness, stroke, and cardiovascular diseases. Most of the deaths occurring from Type 2 DM and the linked diseases take place at the initial stages. In this study, a novel machine learning technique is implemented that combines Back Propagation Neural Network (BPNN) and Naïve Bayes(NB) classifiers for predicting diabetes, and thereby detecting the associated diseases like DM and DN. Further, the proposed technique is analyzed for different evaluation metrics like accuracy, precision, recall and false positive rate. Finally, the performance of the proposed approach is compared with existing techniques like BPNN and NB. The proposed approach has a prediction accuracy of 93% which is higher than the conventional techniques.
Key-Words / Index Term
Diabetes, Prediction, BPNN, NB, Ensemble
References
[1] S. Thomas and J. Karalliedde, “Diabetic nephropathy,” Medicine (Baltimore)., vol. 47, no. 2, pp. 86–91, Feb. 2019.
[2] The Statistics Portal, “Diabetes - Statistics and Facts,” 2019. [Online]. Available: https://www.statista.com/topics/1723/diabetes/. [Accessed: 29-Apr-2019].
[3] World Health Organization, “Global Status Report On Noncommunicable Diseases,” 2014. [Online]. Available: https://apps.who.int/iris/bitstream/handle/10665/148114/9789241564854_eng.pdf?sequence=1. [Accessed: 31-Jan-2019].
[4] M. Motwani et al., “Machine learning for prediction of all-cause mortality in patients with suspected coronary artery disease: a 5-year multicentre prospective registry analysis.,” Eur. Heart J., vol. 38, no. 7, pp. 500–507, Feb. 2017.
[5] A. Chandiok and D. K. Chaturvedi, “Machine learning techniques for cognitive decision making,” in 2015 IEEE Workshop on Computational Intelligence: Theories, Applications and Future Directions (WCI), 2015, pp. 1–6.
[6] W. Raghupathi and V. Raghupathi, “Big data analytics in healthcare: promise and potential,” Heal. Inf. Sci. Syst., vol. 2, no. 1, p. 3, Dec. 2014.
[7] I. Segura-Bedmar, C. Colón-Ruíz, M. Á. Tejedor-Alonso, and M. Moro-Moro, “Predicting of anaphylaxis in big data EMR by exploring machine learning approaches,” J. Biomed. Inform., vol. 87, pp. 50–59, Nov. 2018.
[8] Anupriya, Saranya, and Deepika, “Mining Health Data in Multimodal Data Series for Disease Prediction,” Int. J. Sci. Res. Comput. Sci. Eng., vol. 6, no. 2, pp. 96–99, 2018.
[9] R. Wang-Sattler et al., “Novel biomarkers for pre-diabetes identified by metabolomics,” Mol. Syst. Biol., vol. 8, Sep. 2012.
[10] S. F. Weng, J. Reps, J. Kai, J. M. Garibaldi, and N. Qureshi, “Can machine-learning improve cardiovascular risk prediction using routine clinical data?,” PLoS One, vol. 12, no. 4, p. e0174944, Apr. 2017.
[11] A. Samant and S. Kadge, “Classification of a Retinal Disease based on Different Supervised Learning Techniques,” Int. J. Sci. Res. Netw. Secur. Commun., vol. 5, no. 3, pp. 1–5, 2017.
[12] A. Dagliati et al., “Machine Learning Methods to Predict Diabetes Complications,” J. Diabetes Sci. Technol., 2018.
[13] M. Makino et al., “Artificial Intelligence Predicts Progress of Diabetic Kidney Disease-Novel Prediction Model Construction with Big Data Machine Learning,” Diabetes, vol. 67, no. Supplement 1, pp. 539-P, May 2018.
[14] C. Zhu, C. U. Idemudia, and W. Feng, “Improved logistic regression model for diabetes prediction by integrating PCA and K-means techniques,” Informatics Med. Unlocked, p. 100179, Apr. 2019.
[15] H. Wu, S. Yang, Z. Huang, J. He, and X. Wang, “Type 2 diabetes mellitus prediction model based on data mining,” Informatics Med. Unlocked, vol. 10, pp. 100–107, 2018.
[16] B. M. Patil, R. C. Joshi, and D. Toshniwal, “Hybrid prediction model for Type-2 diabetic patients,” Expert Syst. Appl., vol. 37, no. 12, pp. 8102–8108, Dec. 2010.
[17] A. Ahmad, A. Mustapha, E. D. Zahadi, N. Masah, and N. Y. Yahaya, “Comparison between Neural Networks against Decision Tree in Improving Prediction Accuracy for Diabetes Mellitus,” in Digital Information Processing and Communications, 2011, pp. 537–545.
[18] A. Marcano-Cedeño, J. Torres, and D. Andina, A Prediction Model to Diabetes Using Artificial Metaplasticity, vol. 6687. 2011.
[19] V. V. Vijayan and C. Anjali, “Decision support systems for predicting diabetes mellitus — A Review,” in 2015 Global Conference on Communication Technologies (GCCT), 2015, pp. 98–103.
[20] D. Jeevanandhini, E. G. Raj, V. D. Kumar, and N. Sasipriyaa, “Prediction of Type2 Diabetes Mellitus Based on Data Mining,” Int. J. Eng. Res. Technol., vol. 6, no. 4, 2018.
[21] G. Yirui, L. Yuqian, W. G. Xiaotian, Z. Luning, Z. Hongyan, and W. Bingyuan, “Application of artificial neural network to predict individual risk of type 2 diabetes mellitus,” J. Zhengzhou Univ. Sci., vol. 49, no. 3, 2014.
[22] N. LaPierre, C. J.-T. Ju, G. Zhou, and W. Wang, “MetaPheno: A critical evaluation of deep learning and machine learning in metagenome-based disease prediction,” Methods, Mar. 2019.
[23] S. Mezzatesta, C. Torino, P. De Meo, G. Fiumara, and A. Vilasi, “A machine learning-based approach for predicting the outbreak of cardiovascular diseases in patients on dialysis,” Comput. Methods Programs Biomed., vol. 177, pp. 9–15, Aug. 2019.
[24] M.-Y. Day and C.-C. Tsai, “A Study on Machine Learning for Imbalanced Datasets with Answer Validation of Question Answering,” in 2016 IEEE 17th International Conference on Information Reuse and Integration (IRI), 2016, pp. 513–519.
[25] Kaggle, “Pima Indians Diabetes Database,” Kaggle, 2019. [Online]. Available: https://www.kaggle.com/uciml/pima-indians-diabetes-database. [Accessed: 31-Jan-2019].