Mean Average Accuracy for Text And Non-Text Images Using SVM Classifier
Chowdhury Md. Mizan1 , Pradipta Karmakar2 , Sayak Dasgupta3 , Tridib Chakraborty4
Section:Research Paper, Product Type: Journal Paper
Volume-7 ,
Issue-5 , Page no. 1648-1651, May-2019
CrossRef-DOI: https://doi.org/10.26438/ijcse/v7i5.16481651
Online published on May 31, 2019
Copyright © Chowdhury Md. Mizan, Pradipta Karmakar, Sayak Dasgupta, Tridib Chakraborty . This is an open access article distributed under the Creative Commons Attribution License, which permits unrestricted use, distribution, and reproduction in any medium, provided the original work is properly cited.
View this paper at Google Scholar | DPI Digital Library
How to Cite this Paper
- IEEE Citation
- MLA Citation
- APA Citation
- BibTex Citation
- RIS Citation
IEEE Citation
IEEE Style Citation: Chowdhury Md. Mizan, Pradipta Karmakar, Sayak Dasgupta, Tridib Chakraborty, “Mean Average Accuracy for Text And Non-Text Images Using SVM Classifier,” International Journal of Computer Sciences and Engineering, Vol.7, Issue.5, pp.1648-1651, 2019.
MLA Citation
MLA Style Citation: Chowdhury Md. Mizan, Pradipta Karmakar, Sayak Dasgupta, Tridib Chakraborty "Mean Average Accuracy for Text And Non-Text Images Using SVM Classifier." International Journal of Computer Sciences and Engineering 7.5 (2019): 1648-1651.
APA Citation
APA Style Citation: Chowdhury Md. Mizan, Pradipta Karmakar, Sayak Dasgupta, Tridib Chakraborty, (2019). Mean Average Accuracy for Text And Non-Text Images Using SVM Classifier. International Journal of Computer Sciences and Engineering, 7(5), 1648-1651.
BibTex Citation
BibTex Style Citation:
@article{Mizan_2019,
author = {Chowdhury Md. Mizan, Pradipta Karmakar, Sayak Dasgupta, Tridib Chakraborty},
title = {Mean Average Accuracy for Text And Non-Text Images Using SVM Classifier},
journal = {International Journal of Computer Sciences and Engineering},
issue_date = {5 2019},
volume = {7},
Issue = {5},
month = {5},
year = {2019},
issn = {2347-2693},
pages = {1648-1651},
url = {https://www.ijcseonline.org/full_paper_view.php?paper_id=4465},
doi = {https://doi.org/10.26438/ijcse/v7i5.16481651}
publisher = {IJCSE, Indore, INDIA},
}
RIS Citation
RIS Style Citation:
TY - JOUR
DO = {https://doi.org/10.26438/ijcse/v7i5.16481651}
UR - https://www.ijcseonline.org/full_paper_view.php?paper_id=4465
TI - Mean Average Accuracy for Text And Non-Text Images Using SVM Classifier
T2 - International Journal of Computer Sciences and Engineering
AU - Chowdhury Md. Mizan, Pradipta Karmakar, Sayak Dasgupta, Tridib Chakraborty
PY - 2019
DA - 2019/05/31
PB - IJCSE, Indore, INDIA
SP - 1648-1651
IS - 5
VL - 7
SN - 2347-2693
ER -
![]() |
![]() |
![]() |
444 | 283 downloads | 201 downloads |
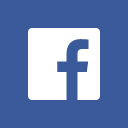
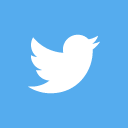
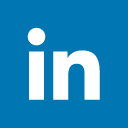
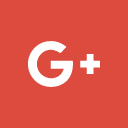
Abstract
Recognition of text and non-text images is a major challenge in the field of computer vision so as to efficiently extract the text from that image. The algorithm used for the extraction of the text from the images would have a higher efficiency if it is known beforehand that the image is a text image or a non-text image. However, there are many images such as old manuscripts where the extraction of the text becomes very difficult. In that case, the algorithm for the distinction between the text and non-text becomes very easy for text detection and have high accuracy and fast in detecting the text from the image. This method can also be applied to detect and extract the text from the signboards also. In our approach, we had built a system that takes any sort of image as an input. After the input of the image, it is then processed and converted into a binary image. Distance transform method is then applied and the measure of the distance between the various points in the image are then calculated. From the calculated points, duplicate points are merged into one point and are sorted in ascending order. The total area of the binary image is then calculated and also the image corresponding to each of the distance transform points are then calculated. The total area of the binary image is then divided by each of the area value of the corresponding distance transform points are the value extracted is known as the feature values. After getting all the feature values the whole value is then divided into small intervals and is then processed through the classifier. The accuracy of the classifier is then calculated and evaluated. This method is a very simple and accurate method for the calculation of the average accuracy of purely text and purely non-text images which can be further used to distinguish between text and non-text images. Experiment have been done with simple text and non-text image dataset and the efficiency of the proposed method is then demonstrated.
Key-Words / Index Term
text and non-text, distance transform, SVM classifier
References
[1]. Najwa Maria Chidiac, Pascal Damein and Charles Yacoub, “A robust algorithm for text extraction from images”, 39th International conference on Telecommunication and Signal Processing, 2016.
[2]. Radhika Patel and Suman K Mitra, “Extracting text from degraded documents”, 5th National Conference on Computer Vision, Pattern Recognition, Image Processing and Graphics, 2015.
[3]. R. Malik and SeongAh chin, “Extraction of text in images”, Proceedings of International Conference on Information Intelligence and Systems, 1999.
[4]. Sezer Karaoglu, Ran Tao, Theo Gevers and Arnold W. M. Smeulders, “Words matter: Scene Text for Image Clssification and Retrieval”, IEEE transactions on multimedia, vol. 19, no. 5, may 2017.
[5]. Chengquan Zhang, Cong Yao, Baoguang Shi and Xiang Bai, “Automatic discrimination of text and non-text natural images”, 13th International Conference on Document Analysis and Recognition, 2015.
[6]. Vishal Chowrasia, Sanjay Shilakari and Rajeev Pandey, “Implementation of Optical Character Recognition Using Machine Learning”,International Journal of Computer Science and Engineering, Vol.-6, Issue-6, jun 2018.