A Comparative study on Logit leaf model (LLM) and Support leaf model (SLM) for predicting the customer churn
Kunchaparthi Jyothsna Latha1 , Markapudi Baburao2 , Chaduvula Kavitha3
Section:Research Paper, Product Type: Journal Paper
Volume-7 ,
Issue-5 , Page no. 1628-1632, May-2019
CrossRef-DOI: https://doi.org/10.26438/ijcse/v7i5.16281632
Online published on May 31, 2019
Copyright © Kunchaparthi Jyothsna Latha, Markapudi Baburao, Chaduvula Kavitha . This is an open access article distributed under the Creative Commons Attribution License, which permits unrestricted use, distribution, and reproduction in any medium, provided the original work is properly cited.
View this paper at Google Scholar | DPI Digital Library
How to Cite this Paper
- IEEE Citation
- MLA Citation
- APA Citation
- BibTex Citation
- RIS Citation
IEEE Style Citation: Kunchaparthi Jyothsna Latha, Markapudi Baburao, Chaduvula Kavitha, “A Comparative study on Logit leaf model (LLM) and Support leaf model (SLM) for predicting the customer churn,” International Journal of Computer Sciences and Engineering, Vol.7, Issue.5, pp.1628-1632, 2019.
MLA Style Citation: Kunchaparthi Jyothsna Latha, Markapudi Baburao, Chaduvula Kavitha "A Comparative study on Logit leaf model (LLM) and Support leaf model (SLM) for predicting the customer churn." International Journal of Computer Sciences and Engineering 7.5 (2019): 1628-1632.
APA Style Citation: Kunchaparthi Jyothsna Latha, Markapudi Baburao, Chaduvula Kavitha, (2019). A Comparative study on Logit leaf model (LLM) and Support leaf model (SLM) for predicting the customer churn. International Journal of Computer Sciences and Engineering, 7(5), 1628-1632.
BibTex Style Citation:
@article{Latha_2019,
author = {Kunchaparthi Jyothsna Latha, Markapudi Baburao, Chaduvula Kavitha},
title = {A Comparative study on Logit leaf model (LLM) and Support leaf model (SLM) for predicting the customer churn},
journal = {International Journal of Computer Sciences and Engineering},
issue_date = {5 2019},
volume = {7},
Issue = {5},
month = {5},
year = {2019},
issn = {2347-2693},
pages = {1628-1632},
url = {https://www.ijcseonline.org/full_paper_view.php?paper_id=4462},
doi = {https://doi.org/10.26438/ijcse/v7i5.16281632}
publisher = {IJCSE, Indore, INDIA},
}
RIS Style Citation:
TY - JOUR
DO = {https://doi.org/10.26438/ijcse/v7i5.16281632}
UR - https://www.ijcseonline.org/full_paper_view.php?paper_id=4462
TI - A Comparative study on Logit leaf model (LLM) and Support leaf model (SLM) for predicting the customer churn
T2 - International Journal of Computer Sciences and Engineering
AU - Kunchaparthi Jyothsna Latha, Markapudi Baburao, Chaduvula Kavitha
PY - 2019
DA - 2019/05/31
PB - IJCSE, Indore, INDIA
SP - 1628-1632
IS - 5
VL - 7
SN - 2347-2693
ER -
![]() |
![]() |
![]() |
410 | 609 downloads | 166 downloads |
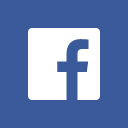
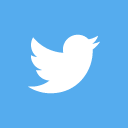
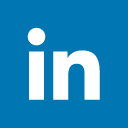
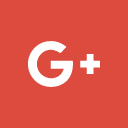
Abstract
Decision trees, logistic regression and support vector machine are very popular algorithms for predicting the customer churn with comprehensibility and well-built predictive performance and. Regardless of the strengths they are having flaws, decision trees having problem to handle the linear relations among the variables, logistic regression is having difficulties to handle interaction effects among the variables, and support vector machine performs marginally better than logistic regression. Consequently a new hybrid algorithm named as support leaf model (SLM) was proposed to classify the data. The idea following the support leaf (SLM) is that implementation of different models on segments of the data gives better predictive performance rather than on the entire dataset, the comprehensibility is maintained from the models which are constructed on the leaves. The SLM consists of two phases, one is segmentation phase and another one is prediction phase. In first stage by using decision tree the customer segments are identified and in the second stage a model is created for every leaf of the tree. To measure the predictive performance area under the receiver operating characteristics curve (AUC) and top decile lift (TDL) are used. Based on the performance metrics AUC and TDL, logit leaf model (LLM) works well when compared with support leaf model (SLM).
Key-Words / Index Term
Customer churn prediction, Hybrid algorithm, Logit leaf model, Support leaf Model, Predictive analytics
References
[1] Michael C. Mozer, Richard Wolniewicz, David B. Grimes,“Predicting Subscriber Dissatisfaction and Improving Retention in the Wireless Telecommunications Industry,” IEEE Transactions On Neural Networks, Vol. 11, pp. 690-696, September 2000.
[2] Colgate, M., Stewart, K., & Kinsella, R. (1996). Customer defection: A study of the student market in Ireland. International Journal of Bank Marketing, 14(3), 23–29.
[3] Ganesh, J., Arnold, M. J., & Reynolds, K. E. (2000). Understanding the customer base of service providers: An examination of the differences between switchers and stayers. Journal of Marketing, 64(3), 65–87.
[4] Shaffer, G., & Zhang, Z. J. (2002). Competitive one-to-one promotions. Management Science, , 48(9), 1143–1160.
[5] Blattberg, R. C., Kim, B. D., & Neslin, S. A. (2010). Database marketing: Analyzing and managing customers. New York, NY: Springer.
[6] Verbeke, W., Dejaeger, K., Martens, D., Hur, J., & Baesens, B. (2012). New insights into churn prediction in the telecommunication sector: A profit driven data mining approach. European Journal of Operational Research, 218(1), 211–229.
[7] Gustafsson, A., Johnson, M. D., & Roos, I. (2005). The effects of customer satisfaction, relationship commitment dimension, and triggers on customer retention. Journal of Marketing, 69(4), 210–218.
[8] Seret, A., Verbraken, T., Versailles, S., & Baesens, B. (2012). A new SOM-based method for profile generation: Theory and application in direct marketing. European Journal of Operational Research, 220, 199–209.
[9] Ali Dehghan, Theodore B. Trafalis, “Examining Churn and Loyalty Using Support Vector Machine”, Scienceedu Press, Vol. 1, (4), pp. 153- 161, December 2012.
[10] Marie Fernandes, “Data mining: A Comparative Study of its various Techniques and its”, IJSRCSE, Volume-5, Issue-1, pp.19-23, February (2017).
[11] G. Sathyadevi, “Application of CART Algorithm in hepatitis Disease Diagnosis”, IEEE-International Conference on Recent Trends in Information Technology, ICRTIT 2011.
[12] mi zhou, “A hybrid feature selection method based on fisher score and genetic algorithm” , Journal of Mathematical Sciences: Advances and Applications Volume 37, 2016, Pages 51-78
[13] Coussement, K., Lessman, S., & Verstraeten, G. (2017). A comparative analysis of data preparation algorithms for customer churn prediction: A case study in the telecommunication. Decision Support Systems, 95, 27–36.
[14] Arno De Caigny, Kristof Coussement, Koen W. De Bock, “A new hybrid classification algorithm for customer churn prediction based on logistic regression and decision trees”, European journal of operational research 269 (2018) 760-772.
[15] S. JabeenBegum, B. Swaathi, “A Survey for identifying Parkinson’s disease by Binary Bat Algorithm”, IJSRCSE, Vol 7,Issue 2, pp.17-23, April (2019).