Dual Threshold Based Classification Technique (DTBCT) For Assessing Liver Abnormalities from Medical Images
R. Malathi1 , S. Ravichandran2
Section:Research Paper, Product Type: Journal Paper
Volume-7 ,
Issue-5 , Page no. 1436-1439, May-2019
CrossRef-DOI: https://doi.org/10.26438/ijcse/v7i5.14361439
Online published on May 31, 2019
Copyright © R. Malathi, S. Ravichandran . This is an open access article distributed under the Creative Commons Attribution License, which permits unrestricted use, distribution, and reproduction in any medium, provided the original work is properly cited.
View this paper at Google Scholar | DPI Digital Library
How to Cite this Paper
- IEEE Citation
- MLA Citation
- APA Citation
- BibTex Citation
- RIS Citation
IEEE Citation
IEEE Style Citation: R. Malathi, S. Ravichandran, “Dual Threshold Based Classification Technique (DTBCT) For Assessing Liver Abnormalities from Medical Images,” International Journal of Computer Sciences and Engineering, Vol.7, Issue.5, pp.1436-1439, 2019.
MLA Citation
MLA Style Citation: R. Malathi, S. Ravichandran "Dual Threshold Based Classification Technique (DTBCT) For Assessing Liver Abnormalities from Medical Images." International Journal of Computer Sciences and Engineering 7.5 (2019): 1436-1439.
APA Citation
APA Style Citation: R. Malathi, S. Ravichandran, (2019). Dual Threshold Based Classification Technique (DTBCT) For Assessing Liver Abnormalities from Medical Images. International Journal of Computer Sciences and Engineering, 7(5), 1436-1439.
BibTex Citation
BibTex Style Citation:
@article{Malathi_2019,
author = {R. Malathi, S. Ravichandran},
title = {Dual Threshold Based Classification Technique (DTBCT) For Assessing Liver Abnormalities from Medical Images},
journal = {International Journal of Computer Sciences and Engineering},
issue_date = {5 2019},
volume = {7},
Issue = {5},
month = {5},
year = {2019},
issn = {2347-2693},
pages = {1436-1439},
url = {https://www.ijcseonline.org/full_paper_view.php?paper_id=4426},
doi = {https://doi.org/10.26438/ijcse/v7i5.14361439}
publisher = {IJCSE, Indore, INDIA},
}
RIS Citation
RIS Style Citation:
TY - JOUR
DO = {https://doi.org/10.26438/ijcse/v7i5.14361439}
UR - https://www.ijcseonline.org/full_paper_view.php?paper_id=4426
TI - Dual Threshold Based Classification Technique (DTBCT) For Assessing Liver Abnormalities from Medical Images
T2 - International Journal of Computer Sciences and Engineering
AU - R. Malathi, S. Ravichandran
PY - 2019
DA - 2019/05/31
PB - IJCSE, Indore, INDIA
SP - 1436-1439
IS - 5
VL - 7
SN - 2347-2693
ER -
![]() |
![]() |
![]() |
360 | 272 downloads | 152 downloads |
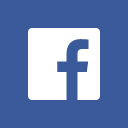
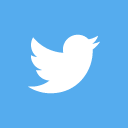
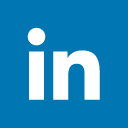
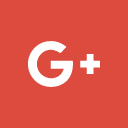
Abstract
Healthcare systems have been using data mining to predict disease in recent years. Early prediction of liver diseases is important to save human life, mainly to decrease mortality rates by taking appropriate disease control measures. This paper explores early predictions of liver disease through various classification techniques. The liver disease dataset selected for this study consists of 15 CT scan images of the liver. The images were segmented with GLCM features. The main purpose of this paper is to propose a hybrid classifier algorithm for predicting liver diseases involving multiple techniques. The proposed technique is also compared with existing classifiers like Naïve Bayes, K nearest neighbor and support vector machine (SVM) on the scales of sensitivity, specificity and classification accuracy. Experimental results of the proposed hybrid classifier algorithm were found to better in predicting liver diseases.
Key-Words / Index Term
Classification, GLCM, liver disease, prediction, standard deviation
References
[1] Bendi Venkata Ramana, Surendra. Prasad Babu. M, Venkateswarlu. N.B, A Critical Study of Selected Classification Algorithms for Liver Disease Diagnosis, International Journal of Database Management Systems ( IJDMS ), Vol.3, No.2, May 2011 page no 101-114
[2] Grimaldi. M, Cunningham. P, Kokaram. A, An evaluation of alternative featureselection strategies and ensemble techniques for classifying music, in: Work-shop in Multimedia Discovery and Mining, ECML/PKDD03, Dubrovnik, Croatia, 2003.
[3] Chaitrali S. Dangare, Sulabha S. Apte, “Improved Study of Heart Disease Prediction System using Data Mining Classification Techniques”, International Journal of Computer Applications (0975 – 888), Volume 47– No.10, June 2012, page no 44-48
[4] Omar S.Soliman, Eman Abo Elhamd, Classification of Hepatitis C Virus using Modified Particle Swarm Optimization and Least Squares Support Vector Machine, International Journal of Scientific & Engineering Research, Volume 5, Issue 3, March-2014 122
[5] Karthik. S, Priyadarishini. A, Anuradha. J and Tripathi. B. K, Classification and Rule Extraction using Rough Set for Diagnosis of Liver Disease and its Types, Advances in Applied Science Research, 2011, 2 (3): page no 334-345
[6] Dhamodharan. S, Liver Disease Prediction Using Bayesian Classification, Special Issue, 4th National Conference on Advanced Computing, Applications & Technologies, May 2014, page no 1-3.
[7] Rosalina. A.H, Noraziah. A. Prediction of Hepatitis Prognosis Using Support Vector Machine and Wrapper Method, IEEE, (2010), 2209- 22
[8] Kotsiantis. S.B, Increasing the Classification Accuracy of Simple Bayesian Classifier, AIMSA, pp. 198-207, 2004
[9] Milan Kumari, Sunila Godara, Comparative Study of Data Mining Classification Methods in Cardiovascular Disease Prediction, International Journal of Computer Sci ence and Technology, Vol. 2, Iss ue 2, June 2011, page no 304-308
[10] Gur Emre Güraksın, Hüseyin Hakli, Harun U˘guz, Support vector machines classification based on particle swarmoptimization for bone age determination, Elsevier publications, Science direct, page no 597- 602
[11] Han, J.; Kamber, M., “Data Mining Concepts and Techniques”. 2nd Edition, Morgan Kaufmann, San Francisco.
[12] Patrick Breheny, Kernel density classification, STA 621: Nonparametric Statistics October 25
[13] Pushpalatha. S, Jagdesh Pandya, Data model comparison for Hepatitis diagnosis, International Journal of Emerging Research in Management &Technology ISSN: 2278-9359 (Volume-3, Issue-7) 2014, page no 138-141