Predictive Analytic for Blood Request by Using Moving Average and Linear Regression
P. Limsaiprom1 , S. Rattanachan2 , N. Phuangphairoj3 , M. Kaenchuwongk4
Section:Research Paper, Product Type: Journal Paper
Volume-7 ,
Issue-5 , Page no. 1323-1329, May-2019
CrossRef-DOI: https://doi.org/10.26438/ijcse/v7i5.13231329
Online published on May 31, 2019
Copyright © P. Limsaiprom, S. Rattanachan, N. Phuangphairoj, M. Kaenchuwongk . This is an open access article distributed under the Creative Commons Attribution License, which permits unrestricted use, distribution, and reproduction in any medium, provided the original work is properly cited.
View this paper at Google Scholar | DPI Digital Library
How to Cite this Paper
- IEEE Citation
- MLA Citation
- APA Citation
- BibTex Citation
- RIS Citation
IEEE Style Citation: P. Limsaiprom, S. Rattanachan, N. Phuangphairoj, M. Kaenchuwongk, “Predictive Analytic for Blood Request by Using Moving Average and Linear Regression,” International Journal of Computer Sciences and Engineering, Vol.7, Issue.5, pp.1323-1329, 2019.
MLA Style Citation: P. Limsaiprom, S. Rattanachan, N. Phuangphairoj, M. Kaenchuwongk "Predictive Analytic for Blood Request by Using Moving Average and Linear Regression." International Journal of Computer Sciences and Engineering 7.5 (2019): 1323-1329.
APA Style Citation: P. Limsaiprom, S. Rattanachan, N. Phuangphairoj, M. Kaenchuwongk, (2019). Predictive Analytic for Blood Request by Using Moving Average and Linear Regression. International Journal of Computer Sciences and Engineering, 7(5), 1323-1329.
BibTex Style Citation:
@article{Limsaiprom_2019,
author = {P. Limsaiprom, S. Rattanachan, N. Phuangphairoj, M. Kaenchuwongk},
title = {Predictive Analytic for Blood Request by Using Moving Average and Linear Regression},
journal = {International Journal of Computer Sciences and Engineering},
issue_date = {5 2019},
volume = {7},
Issue = {5},
month = {5},
year = {2019},
issn = {2347-2693},
pages = {1323-1329},
url = {https://www.ijcseonline.org/full_paper_view.php?paper_id=4408},
doi = {https://doi.org/10.26438/ijcse/v7i5.13231329}
publisher = {IJCSE, Indore, INDIA},
}
RIS Style Citation:
TY - JOUR
DO = {https://doi.org/10.26438/ijcse/v7i5.13231329}
UR - https://www.ijcseonline.org/full_paper_view.php?paper_id=4408
TI - Predictive Analytic for Blood Request by Using Moving Average and Linear Regression
T2 - International Journal of Computer Sciences and Engineering
AU - P. Limsaiprom, S. Rattanachan, N. Phuangphairoj, M. Kaenchuwongk
PY - 2019
DA - 2019/05/31
PB - IJCSE, Indore, INDIA
SP - 1323-1329
IS - 5
VL - 7
SN - 2347-2693
ER -
![]() |
![]() |
![]() |
281 | 233 downloads | 105 downloads |
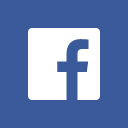
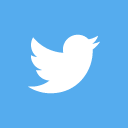
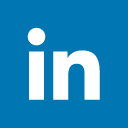
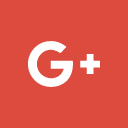
Abstract
The main objective of this research predicted the number of blood request in each month of the organization. In this research, we compared 3 statistical estimation methods (simple moving average, exponential moving average, and linear regression) to predict the number of requested blood in each month using historical time series data. Reducing the error of the expected requested blood would be beneficial for planning and decision making to prepare for the target of blood donation. Data used in this experiment, were the number of requested blood components (red blood cells) from 5 years which divided into two periods. The first period, from January 2014 to December 2017, was used as training set for building linear regression models. The second period, from January 2018 to December 2018, was used as test set to evaluate the regression models and to compare with simple moving average and exponential moving average. The results presented that the exponential moving average method had significantly lowered errors than both the simple moving average method and the linear regression method.
Key-Words / Index Term
Requested blood, Simple moving average, Exponential moving average, Linear regression
References
[1] K. Saritha and S. Abraham, “Statistical Predictability in Big Data Analytics with Data Partitioning”, International Journal of computer Science and Engineering, Vol.06, Issue.06, pp.80-55, 2018.
[2] N.M. Annigeri, S. Shetty, A.P. Patil, “Analysing the supervised learning methods for predicting of healthcare data in cloud environment: A Survey”, International Journal of computer Science and Engineering, Vol.06, Issue.3, pp.434-438, 2018.
[3] M. Fernandes, “Data Mining: A Comparative Study of its Various Techniques and its Process, International Journal of computer Science and Engineering, Vol.5, Issue.1, pp.19-23, 2017.
[4] J.V.N. Lakshmi, A. Sheshasaayee, “A Big Data Analytical Approach for Analyzing Temperature Dataset using Machinee Learning Techniques”, International Journal of computer Science and Engineering, Vol.5, Issue.3, pp.92-97, 2017.
[5] R. Daosa-nguan, C. Hansa, S.Maneerat, “Comparative study of suitable models for forecasting Number of patients with epidemiological surveillance in Bangkok”, Research and Development Journal, KMUTT, Vol.38, No.1, pp.33-55, 2015.
[6] S. Ronachai, K. Kittisak and K. Nittaya, “Forecasting the demand for power distribution units with support vector regression. 3 alternate examination forms”, Journal of Science and Technology Ubon Ratchathani University. Vol.19, Issue.1, pp.215-232, 2017.
[7] T. Chalermchat, “Comparison of forecasting methods for electricity consumption in Nakhon Phanom province”, Narasuan University Journal: Science and Technology, Vol.25, Issue.4, pp.124-137, 2017.
[8] C. Thanaporn and C. Paramet, “Forecasting of the services of the customers in retail business”, Journal of King Mongkut`s University of Technology North Bangkok, Vol.23, Issue.3, pp.664-674, 2013.
[9] P. Komon, “The forecasting model of manpower suitable for workload: a case study of a branch shop, CP All Public Company Limited”, Panyapiwat Journal. Vol.5, Issue.2, pp.103-119, 20142.
[10] S. Nicha and K. Sumet, “Forecasting water demand for consumption in the future using Arya model and Garch model”, KKU Res. J. (be), Vol.11, Issue.1, pp.45-55, 2012.
[11] E. Agthe,, Donald. and R.B. Billings, “Dynamic Modals of Residential Water Demand”, Water Resources Research, Vol.16, Issue.June, pp.476-480, 1980.
[12] E.L. Danielson, “An analysis of Residential Demand for water using Micro Time Series Data”, Water Resources Research, Vol.15, Issue.August, pp.763-767, 1979.
[13] S.J. Henry, and R.B. Bruce, “Urban Residential Demand for Water in the United States”, Land Economics, Vol.55, Issue.February, pp.43-58, 1979.
[14] W. Charles and F.P. Linaweaver,Jr, “The Impact of Price on Residential Water Demand and Its Relation to System Design and Price Structure”, Water Resources Resarch, Vol.3, Issue.February, pp.13-32, 1967.