Network Intrusion Detection Exploitation Machine Learning Strategies with the Utilization of Feature Elimination Mechanism
Safura A. Mashayak1 , Balaji R. Bombade2
Section:Research Paper, Product Type: Journal Paper
Volume-7 ,
Issue-5 , Page no. 1292-1300, May-2019
CrossRef-DOI: https://doi.org/10.26438/ijcse/v7i5.12921300
Online published on May 31, 2019
Copyright © Safura A. Mashayak, Balaji R. Bombade . This is an open access article distributed under the Creative Commons Attribution License, which permits unrestricted use, distribution, and reproduction in any medium, provided the original work is properly cited.
View this paper at Google Scholar | DPI Digital Library
How to Cite this Paper
- IEEE Citation
- MLA Citation
- APA Citation
- BibTex Citation
- RIS Citation
IEEE Style Citation: Safura A. Mashayak, Balaji R. Bombade, “Network Intrusion Detection Exploitation Machine Learning Strategies with the Utilization of Feature Elimination Mechanism,” International Journal of Computer Sciences and Engineering, Vol.7, Issue.5, pp.1292-1300, 2019.
MLA Style Citation: Safura A. Mashayak, Balaji R. Bombade "Network Intrusion Detection Exploitation Machine Learning Strategies with the Utilization of Feature Elimination Mechanism." International Journal of Computer Sciences and Engineering 7.5 (2019): 1292-1300.
APA Style Citation: Safura A. Mashayak, Balaji R. Bombade, (2019). Network Intrusion Detection Exploitation Machine Learning Strategies with the Utilization of Feature Elimination Mechanism. International Journal of Computer Sciences and Engineering, 7(5), 1292-1300.
BibTex Style Citation:
@article{Mashayak_2019,
author = {Safura A. Mashayak, Balaji R. Bombade},
title = {Network Intrusion Detection Exploitation Machine Learning Strategies with the Utilization of Feature Elimination Mechanism},
journal = {International Journal of Computer Sciences and Engineering},
issue_date = {5 2019},
volume = {7},
Issue = {5},
month = {5},
year = {2019},
issn = {2347-2693},
pages = {1292-1300},
url = {https://www.ijcseonline.org/full_paper_view.php?paper_id=4405},
doi = {https://doi.org/10.26438/ijcse/v7i5.12921300}
publisher = {IJCSE, Indore, INDIA},
}
RIS Style Citation:
TY - JOUR
DO = {https://doi.org/10.26438/ijcse/v7i5.12921300}
UR - https://www.ijcseonline.org/full_paper_view.php?paper_id=4405
TI - Network Intrusion Detection Exploitation Machine Learning Strategies with the Utilization of Feature Elimination Mechanism
T2 - International Journal of Computer Sciences and Engineering
AU - Safura A. Mashayak, Balaji R. Bombade
PY - 2019
DA - 2019/05/31
PB - IJCSE, Indore, INDIA
SP - 1292-1300
IS - 5
VL - 7
SN - 2347-2693
ER -
![]() |
![]() |
![]() |
802 | 177 downloads | 116 downloads |
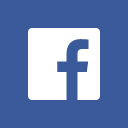
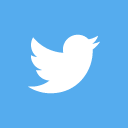
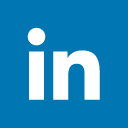
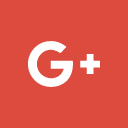
Abstract
The momentous concerns is to discard irrelevant features to boost up the detection rate. There are major problems associated with the feasibility and tolerance with the inception of recent technologies. To realize this objective, we tend to illustrate our model manipulating recursive feature elimination mechanism to reject inutile attributes that are operated on Decision Tree Classifier (DTC) and random forest algorithm (RFA). The experiment is carried on New Subset Labeled version of the KDD`99 dataset (NSL-KDD) dataset that is associated degree updated version of Knowledge Discovery and Data Mining 1999 (KDD’99) dataset. The proposed methodology is discriminated with other strategies illustrated by the previous researchers. It is classified into four distinct categories illustrates the attack classes and one as normal traffic. The model’s capability has been increased to thirteen category classification to compare the tolerance when the number of attack categories will increase. It offers excellent performance analysis metrics to assess the exploitation of our model.
Key-Words / Index Term
Intrusion Detection System, DTC, RFA, KDD, Machine Learning
References
[1] N. Shone, T. N. Ngoc, Vu Dinh Phai, and Qi Shi, “A Deep Learning Approach to Network Intrusion Detection”, IEEE Transactions on Emerging Topics in Computational Intelligence, Vol. 2, NO. 1, pp. 41-50, Feb. 2018.
[2] Z. Liang, G. Zhang, J. X. Huang, and Q. V. Hu, “Deep learning for healthcare decision making with EMRs,” In the Proceedings of the 2014 IEEE International Conference on Bioinformatics. Biomed., pp. 556–559, Nov. 2014.
[3] L. You, Y. Li, Y. Wang, J. Zhang, and Y. Yang, “A deep learning-based RNNs model for an automatic security audit of short messages,” In the Proceedings of the 16th International Symposium on Communications and Information Technologies, Qingdao, China, pp. 225–229, Sep. 2016.
[4] A. Luckow, M. Cook, N. Ashcraft, E. Weill, E. Djerekarov, and B. Vorster, “Deep learning in the automotive industry: Applications and tools,” In the Proceedings of IEEE International Conference on Big Data, pp. 3759–3768. Dec. 2016.
[5] R. Polishetty, M. Roopaei, and P. Rad, “A next-generation secure cloud-based deep learning license plate recognition for smart cities,” Proceedings of 15th IEEE International Conference on Machine Learning Application, Anaheim, CA, USA, pp. 286–293, Dec. 2016.
[6] H. Lee, Y. Kim, and C. O. Kim, “A deep learning model for robust wafer fault monitoring with sensor measurement noise,” IEEE Transaction Semicond. Manuf., vol. 30, no. 1, pp. 23–31, Feb. 2017.
[7] F. Falcini, G. Lami, and A. M. Costanza, “Deep learning in automotive software,” IEEE Softw., vol. 34, no. 3, pp. 56–63, May 2017.
[8] S. P. Shashikumar, A. J. Shah, Q. Li, G. D. Clifford, and S. Nemati, “A deep learning approach to monitoring and detecting atrial fibrillation using wearable technology,” in Proc. IEEE EMBS Int. Conf. Biomed. Health Information, FL, USA, pp. 141–144, 2017.
[9] C. Yin, Y. Zhu, J. Fei, and X. He, “A Deep Learning Approach for Intrusion Detection Using Recurrent Neural Networks” IEEE Access, vol. 5, pp. 21954–21961, Nov. 2017.
[10] D. Farid, N. Harbi, and Z. Rahman, "Combining Nave Bayes and Decision Tree for Adaptive Intrusion Detection," International Journal of Network Security & Its Applications, Vol. 2, No. 2, pp. 12-25, April 2010.
[11] J. Zhang, M. Zulkernine, A. Haque, “Random-Forests-Based Network Intrusion Detection Systems,” IEEE Transactions on Systems, pp. 649–659, Sep 2008.
[12] T. A. Tang, L. Mhamdi, D. McLernon, S. A. R. Zaidi, and M. Ghogho, ‘‘Deep learning approach for network intrusion detection in software-defined networking,’’ In the Proceedings of 2016 International Conference on Wireless Networks and Mobile Communications (WINCOM), pp. 258–263, Oct. 2016.
[13] B. Senthilnayaki, K. Venkatalakshmi, and A. Kannan, " Intrusion Detection Using Optimal Genetic Feature Selection and SVM based Classifier," In the Proceedings of 3rd International Conference on Signal Processing Communication and Networking (ICSCN) Intrusion, pp.1–4,2015.
[14] L. Dhanabal, S. P. Shantharajah "A Study on NSL_KDD Dataset for Intrusion Detection System Based on Classification Algorithms," International Journal of Advanced Research in Computer and Communication Engineering, vol. 4, issue 6, pp. 446-452, June 2015.
[15] F. Zhang, and D. Wang, "An Effective Feature Selection Approach for Network Intrusion Detection," In the Proceedings of Eighth International Conference on Networking, Architecture, and Storage, 2013.