Diseased area Detection & Quantification of Betel-vine leaves, Affected by Leaf rot disease
Sannihita Pattanaik1 , Chandra Sekhar Panda2
Section:Research Paper, Product Type: Journal Paper
Volume-7 ,
Issue-5 , Page no. 573-577, May-2019
CrossRef-DOI: https://doi.org/10.26438/ijcse/v7i5.573577
Online published on May 31, 2019
Copyright © Sannihita Pattanaik, Chandra Sekhar Panda . This is an open access article distributed under the Creative Commons Attribution License, which permits unrestricted use, distribution, and reproduction in any medium, provided the original work is properly cited.
View this paper at Google Scholar | DPI Digital Library
How to Cite this Paper
- IEEE Citation
- MLA Citation
- APA Citation
- BibTex Citation
- RIS Citation
IEEE Style Citation: Sannihita Pattanaik, Chandra Sekhar Panda, “Diseased area Detection & Quantification of Betel-vine leaves, Affected by Leaf rot disease,” International Journal of Computer Sciences and Engineering, Vol.7, Issue.5, pp.573-577, 2019.
MLA Style Citation: Sannihita Pattanaik, Chandra Sekhar Panda "Diseased area Detection & Quantification of Betel-vine leaves, Affected by Leaf rot disease." International Journal of Computer Sciences and Engineering 7.5 (2019): 573-577.
APA Style Citation: Sannihita Pattanaik, Chandra Sekhar Panda, (2019). Diseased area Detection & Quantification of Betel-vine leaves, Affected by Leaf rot disease. International Journal of Computer Sciences and Engineering, 7(5), 573-577.
BibTex Style Citation:
@article{Pattanaik_2019,
author = {Sannihita Pattanaik, Chandra Sekhar Panda},
title = {Diseased area Detection & Quantification of Betel-vine leaves, Affected by Leaf rot disease},
journal = {International Journal of Computer Sciences and Engineering},
issue_date = {5 2019},
volume = {7},
Issue = {5},
month = {5},
year = {2019},
issn = {2347-2693},
pages = {573-577},
url = {https://www.ijcseonline.org/full_paper_view.php?paper_id=4282},
doi = {https://doi.org/10.26438/ijcse/v7i5.573577}
publisher = {IJCSE, Indore, INDIA},
}
RIS Style Citation:
TY - JOUR
DO = {https://doi.org/10.26438/ijcse/v7i5.573577}
UR - https://www.ijcseonline.org/full_paper_view.php?paper_id=4282
TI - Diseased area Detection & Quantification of Betel-vine leaves, Affected by Leaf rot disease
T2 - International Journal of Computer Sciences and Engineering
AU - Sannihita Pattanaik, Chandra Sekhar Panda
PY - 2019
DA - 2019/05/31
PB - IJCSE, Indore, INDIA
SP - 573-577
IS - 5
VL - 7
SN - 2347-2693
ER -
![]() |
![]() |
![]() |
311 | 201 downloads | 201 downloads |
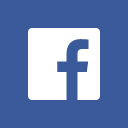
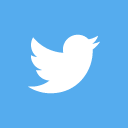
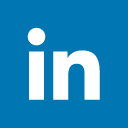
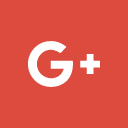
Abstract
With natural calamities, plant disease also plays a major role in severe damage of agricultural product. Hence it is very much important to prevent the crop from being affected by different type of diseases. Likewise betel-vine which is also known as “the green gold of India” is affected by different kind of diseases during its short life period. But leaf rot disease affects the plant all over the year, which is a great loss to the farmer, as twenty million of people of our country make their livelihood directly or indirectly from betel vine. Here I proposed two methods to detect the affected area and quantify the area exactly in betel vine leaf so that, leaves can be protected from severe damage by applying exact amount of pesticides as needed in time and this is the novel aim behind this research work. Hence two methods are simulated namely Otsu’s global thresholding and K-means clustering to get the ROI clearly after segmentation and finally made a comparison to know, which one is giving better result. By applying Otsu’s methodology (PM-1), it is evident from table that the precision of (PM-1) is very high, but the recall value is low, as the average recall value is only 52%. But experimental results shown that K-means is better one with very high precision and high recall value where, the average recall value is 0.9366 or 93.66%.
Key-Words / Index Term
Segmentation, ROI, Detection, Quantify, Precision, Recall
References
[1] M Jhuria, A kumar and R Borse, ”Image processing for Smart farming, detection of Disease and Fruit Grading,” Proceedings of the 2013 International Conference on Image Information processing, pp. 521-526, 2016
[2] A K Dey, M Sharma, M.R.Meshram, ”Image Processing Based
Leaf Rot Disease, Detection of Betel Vine”, Proceedings of the 2016 International Conference on computational Modeling and Security, CMS, pp.748-754 , 2016
[3] J. Vijaykumar, S. Arumugam, ”Early Detection of Powdery mildew Disease for Betel vine plants Using Digital Image Analysis”, International Journal of Modern Engineering Research, vol.2.Issue 4, pp. 2581-2583, 2012
[4] P.Tamilshankar, Dr.T.Gunasekar,”Computer Aided Diseases Identification for Betel Leaf”, International Research Journal of Engineering Technology ,Vol 2, Issue 9, pp. 2577-2581, 2015
[5] J.Vijayakumar, ”Powdery mildew disease Identification in Pachaikodi variety of Betel vine plants using Histogram and Neural Network based Digital Imaging Techniques, International Research Journal of Engineering Technology, Vol 3, Issue 2, pp. 145-1152, 2016
[6] Abdullah NE, Rahim AA, Hashim H, Kamal MM “Classification of Rubber tree leaf diseases using multilayer Perceptron neural network”, Proceedings of IEEE 2007 5th Student Conference on Research and Development. , pp 16.
[7] M Bhange, H.A.Hingoliwala, “Smart Framing: Pomegranate Disease Detection Using Image Processing”, Proceedings of 2015 International Symposium on Computer Vision and Internet (VisionNet’15),pp. 280-288
[8] Ehsan Kiani, Tofik Mamedov, “ Identification of Plant Disease Infection using soft-computing : Application to modern botany”,9th International Conference on Theory and application of Soft Computing ,Computing with Words and Perception, pp. 24-25 ,2017
[9] Riddhi H. Shaparia, Narendra M.Patel, Zankhana H.Shah “Flower Classification using Different Color Channel”, International Journal of Scientific Research in Computer Science and Engineering, Vol.7, issue 2,pp. 1-6, 2019
[10] V. Davis, S. Devane, "Diagnosis of Brain Hemorrhage Using Artificial Neural Network", International Journal of Scientific Research in Network Security and Communication, Vol.5, Issue.1, pp.20-23, 2017