Review of Electromyography Signal with Detection, Decomposition, Features And Classifier Theories
Reema Jain1 , Vijay Kumar Garg2
Section:Review Paper, Product Type: Journal Paper
Volume-7 ,
Issue-5 , Page no. 487-500, May-2019
CrossRef-DOI: https://doi.org/10.26438/ijcse/v7i5.487500
Online published on May 31, 2019
Copyright © Reema Jain, Vijay Kumar Garg . This is an open access article distributed under the Creative Commons Attribution License, which permits unrestricted use, distribution, and reproduction in any medium, provided the original work is properly cited.
View this paper at Google Scholar | DPI Digital Library
How to Cite this Paper
- IEEE Citation
- MLA Citation
- APA Citation
- BibTex Citation
- RIS Citation
IEEE Citation
IEEE Style Citation: Reema Jain, Vijay Kumar Garg, “Review of Electromyography Signal with Detection, Decomposition, Features And Classifier Theories,” International Journal of Computer Sciences and Engineering, Vol.7, Issue.5, pp.487-500, 2019.
MLA Citation
MLA Style Citation: Reema Jain, Vijay Kumar Garg "Review of Electromyography Signal with Detection, Decomposition, Features And Classifier Theories." International Journal of Computer Sciences and Engineering 7.5 (2019): 487-500.
APA Citation
APA Style Citation: Reema Jain, Vijay Kumar Garg, (2019). Review of Electromyography Signal with Detection, Decomposition, Features And Classifier Theories. International Journal of Computer Sciences and Engineering, 7(5), 487-500.
BibTex Citation
BibTex Style Citation:
@article{Jain_2019,
author = {Reema Jain, Vijay Kumar Garg},
title = {Review of Electromyography Signal with Detection, Decomposition, Features And Classifier Theories},
journal = {International Journal of Computer Sciences and Engineering},
issue_date = {5 2019},
volume = {7},
Issue = {5},
month = {5},
year = {2019},
issn = {2347-2693},
pages = {487-500},
url = {https://www.ijcseonline.org/full_paper_view.php?paper_id=4270},
doi = {https://doi.org/10.26438/ijcse/v7i5.487500}
publisher = {IJCSE, Indore, INDIA},
}
RIS Citation
RIS Style Citation:
TY - JOUR
DO = {https://doi.org/10.26438/ijcse/v7i5.487500}
UR - https://www.ijcseonline.org/full_paper_view.php?paper_id=4270
TI - Review of Electromyography Signal with Detection, Decomposition, Features And Classifier Theories
T2 - International Journal of Computer Sciences and Engineering
AU - Reema Jain, Vijay Kumar Garg
PY - 2019
DA - 2019/05/31
PB - IJCSE, Indore, INDIA
SP - 487-500
IS - 5
VL - 7
SN - 2347-2693
ER -
![]() |
![]() |
![]() |
433 | 240 downloads | 170 downloads |
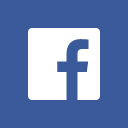
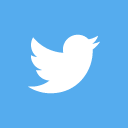
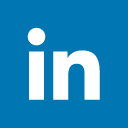
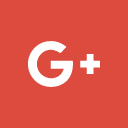
Abstract
Muscle is an essential organ of the body accountable for movements. EMG has a wide range of research from electrode design to recording methods, analytical methods, and various applications. The aim of this paper is to review EMG to understand and decomposition in a concise manner. Extraction and classification of features are has considered demanding tasks as it allows a consistent assessment of the neuromuscular diseases. This manuscript has described various methods of extraction and classification of features that would help to understand their nature and process of adoption. In the evaluation of EMG signals, a number of analysts had tried their hands, so in this paper, we have tried to integrate best of the best researchers that could be advantageous for further analysis. Comparison of the traditional researchers by J. L. Betthauser et al., O. W. Samuel Zhou Hui et al. and Xiangyang Zhu et al. has been conducted to interpret the optimum techniques for the evaluation Betthauser et al. has shown 89% of accuracy with Enhanced Adaptive Sparse Representation Classification (EASRC) technique, O. W. Samuel Zhou Hui et al. has shown 92% of accuracy with LDA and ANN technique and Xiangyang Zhu has used LDA-CA technique with 91% of accuracy.
Key-Words / Index Term
Electromyography, Motor Unit Action Potential, Detection, Decomposition, Features, Classifiers
References
[1] DA Winter, “Pathologic gait diagnosis with computer-averaged electromyographic profiles”, Archives of physical medicine and rehabilitation, Vol. 65, No.7, pp.393-398, 1984.
[2] J. Fang , G.C. Agarwal and B.T. Shahani, “Decomposition of EMG signal by wavelet spectrum matching”, In Proceedings of the 19th Annual International Conference of the IEEE Engineering in Medicine and Biology Society.`Magnificent Milestones and Emerging Opportunities in Medical Engineering`(Cat. No. 97CH36136), Vol. 3, pp. 1253-1256, 1997.
[3] P. Bonato, T. D`Alessio and M. Knaflitz, “A statistical method for the measurement of the muscle activation intervals from surface myoelectric signal gait”, IEEE Transactions on biomedical engineering, Vol. 45, No. 3, pp. 287-299,1998.
[4] A. Merlo , D. Farina, and R. Merletti, “A fast and reliable technique for muscle activity detection from surface EMG signals”, IEEE Transactions on Biomedical Engineering , Vol. 50 , No. 3,pp.316-323,2003.
[5] Zennaro , P. Wellig , V.M. Koch , G.S. Moschytz and T. Laubli, “A software package for the decomposition of long-term multichannel EMG signals using wavelet coefficients”, IEEE Transactions on Biomedical Engineering, Vol. 50, No.1,pp. 58 – 69,2003.
[6] Yamada, Rie, Junichi Ushiba, Yutaka Tomita, and Yoshihisa Masakado. "Decomposition of the electromyographic signal by principal component analysis of wavelet coefficients." In IEEE EMBS Asian-Pacific Conference on Biomedical Engineering, 2003. pp. 118-119. IEEE, 2003.
[7] Lanyi Xu and A. Adler, “An improved method for muscle activation detection during gait”, In Canadian Conference on Electrical and Computer Engineering (IEEE Cat. No. 04CH37513), Vol. 1, pp. 357-360, 2004.
[8] M. B. I. Reaz, M. S. Hussain and F. Mohd-Yasin, “Techniques of EMG signal analysis: detection, processing, classification and Applications”, (Correction), Biological procedures online, Vol. 8, No. 1, pp.163-163, 2006.
[9] Kavita Mahajan, M. R Vargantwar, Sangita M. Rajput, “Classification of EEG using PCA, ICA and Neural Network”, International Journal of Engineering and Advanced Technology (lJEAT), Vol. l, No. 1, pp.80-83, 2011.
[10] Dixon, Anna MR, Emily G. Allstot, Debashish Gangopadhyay, and David J. Allstot. "Compressed sensing system considerations for ECG and EMG wireless biosensors." IEEE Transactions on Biomedical Circuits and Systems 6, no. 2 ,pp. 156-166,2012..
[11] J. Sedlak, D. Spulak, R. Cmejla, R. Bacakova, M. Chrastkova and B. Kracmar, “Segmentation of surface EMG signals”, International Conference on Applied Electronics, Pilsen, pp. 1-4,2013.
[12] Muhammad Ibn Ibrahimy, Md. Rezwanul Ahsan and Othman Omran Khalifa, “Design and Optimization of Levenberg-Marquardt based Neural Network Classifier for EMG Signals to Identify Hand Motions”, Measurement Science Review, Vol. 13, No. 3, pp. 142-152, 2013.
[13] T. Matsubara and J. Morimoto, “Bilinear Modeling of EMG Signals to Extract User-Independent Features for Multiuser Myoelectric Interface”, IEEE Transactions on Biomedical Engineering, Vol. 60, No. 8, pp. 2205-2213, 2013.
[14] B. M. S. U. Doulah, W.-P. Zhu, and M. O. Ahmad, Fellow, IEEE, “Wavelet Domain Feature Extraction Scheme Based on Dominant Motor Unit Action Potential of EMG Signal for Neuromuscular Disease Classification”, IEEE Trans. Biomed. Eng., Vol. 8, No. 2, pp.155-164, April 2014.
[15] A. B. M. S. U. Doulah, S. A. Fattah, W. Zhu, and M. O. Ahmad, “Wavelet Domain Feature Extraction Scheme Based on Dominant Motor Unit Action Potential of EMG Signal for Neuromuscular Disease Classification”, IEEE Transactions on Biomedical Circuits and Systems, Vol. 8, No. 2, pp. 155-164, 2014.
[16] T. Kamali, R. Boostani and H. Parsaei, “A Multi-Classifier Approach to MUAP Classification for Diagnosis of Neuromuscular Disorders”, IEEE Transactions on Neural Systems and Rehabilitation Engineering, Vol. 22, No. 1, pp. 191-200, 2014.
[17] V. Kehri, R. Ingle, R. Awale, S. OimbeS.S. Lakshmi , V. Selvam, and M. Pallikonda Rajasekaran, “EEG signal classification using Principal Component Analysis and Wavelet Transform with Neural Network," International Conference on Communication and Signal Processing, Melmaruvathur, pp. 687-690.IEEE, 2014.
[18] Neetu Gupta, “Comparative Study of type-1 and Type-2 Fuzzy Systems”, International Journal of Engineering Research and General Science, Vol. 2, No. 4, pp.195-198, 2014.
[19] P. Geethanjali and K. K. Ray, “A Low-Cost Real-Time Research Platform for EMG Pattern Recognition-Based Prosthetic Hand”, IEEE/ASME Transactions on Mechatronics, Vol. 20, No. 4, pp. 1948-1955, 2015.
[20] I.Elamvazuthi, N.H.X.Duy,Z. Ali,S.W.Su,M.K.A. Ahamed Khan and S.Parasuramand, “Electromyography (EMG) based Classification of Neuromuscular Disorders using Multi-Layer Perceptron”, Procedia Computer Science, Vol. 76, pp. 223-228,2015.
[21] S.M.Mane, R.A.Kambli, F.S.Kazi Prof.and N.M.Singh Prof., “Hand Motion Recognition from Single Channel Surface EMG Using Wavelet & Artificial Neural Network”, Procedia Computer Science, Vol.49, pp. 58-65, 2015.
[22] S. Thongpanja, A. Phinyomark, F. Quaine, Y. Laurillau, C. Limsakul, P. Phukpattaranont, “Probability density functions of stationary surface EMG signals in noisy environments”, IEEE Trans. Instru. Meas, Vol. 65, pp. 1547-1557, 2016.
[23] J. A. Spanias, E. J. Perreault and L. J. Hargrove, “Detection of and Compensation for EMG Disturbances for Powered Lower Limb Prosthesis Control", IEEE Transactions on Neural Systems and Rehabilitation Engineering, Vol. 24, No. 2, pp. 226-234, 2016.
[24] J. Wu, L. Sun and R. Jafari, “A Wearable System for Recognizing American Sign Language in Real-Time Using IMU and Surface EMG Sensors”, IEEE Journal of Biomedical and Health Informatics, Vol. 20, No. 5, pp. 1281-1290, 2016.
[25] Cemil Altın and Orhan Er, “Comparison of Different Time and Frequency Domain Feature Extraction Methods on Elbow Gesture’s EMG”, European Journal of Interdisciplinary Studies, Vol. 2 No.3, pp.35-44, 2016.
[26] G. Jang, J. Kim, S.n Lee, and Y. Choi, “EMG-Based Continuous Control Scheme With Simple Classifier for Electric-Powered Wheelchair”, IEEE Transactions on Industrial Electronics, Vol. 63 , No. 6 , pp.3695 – 3705, 2016.
[27] N. Nazmi, Mohd A. Abdul Rahman, S.I. Yamamoto, S. A. Ahmad, H. Zamzuri and S. A. Mazlan, “A Review of Classification Techniques of EMG Signals during Isotonic and Isometric Contractions”, Sensors, pp.2-28, Vol. 16, No. 8, 2016.
[28] M. I. Ibrahimy, M. R. Ahsan and O. O. Khalifa, “Design and optimization of a neural classifier to identify around shoulder motions”, Optik, Vol. 127, No. 7, pp.3564-3568, 2016.
[29] A. Boyali and N. Hashimoto, “Spectral Collaborative Representation based Classification for hand gestures recognition on electromyography signals”, Biomedical Signal Processing and Control, Vol. 24, pp. 11-18,2016.
[30] Amrutha N and Arul V H, “A Review on Noises in EMG Signal and its Removal”, International Journal of Scientific and Research Publications, Vol. 7, No. 5, pp. 23-27, 2017.
[31] Djelouat, Hamza, Hamza Baali, Abbes Amira, and Faycal Bensaali. "IoT Based Compressive Sensing for ECG Monitoring." In 2017 IEEE International Conference on Internet of Things (iThings) and IEEE Green Computing and Communications (GreenCom) and IEEE Cyber, Physical and Social Computing (CPSCom) and IEEE Smart Data (SmartData), pp. 183-189. IEEE, 2017.
[32] Djelouat, Hamza, Hamza Baali, Abbes Amira, and Faycal Bensaali. "Joint sparsity recovery for compressive sensing based EEG system." In 2017 IEEE 17th International Conference on Ubiquitous Wireless Broadband (ICUWB), pp. 1-5. IEEE, 2017.
[33] X. Zhu,J. Liu,D. Zhang,D. Zhang and N. Jiang, “Cascaded Adaptation Framework for Fast Calibration of Myoelectric Control”, IEEE Transactions On Neural Systems And Rehabilitation Engineering, Vol. 25, No.3, pp. 254-264,2017.
[34] Sunil Ray, “Understanding Support Vector Machine algorithm from examples (along with code)”, Analytics Vidhya, Vol. 13, 2017
[35] S. W. Lee, T. Yi, J. Jun, and Z. Bien, “Design of a Gait Phase Recognition System That Can Cope With EMG Electrode Location Variation”, IEEE Transactions on Automation Science and Engineering, Vol. 14, No. 3, pp. 1429-1439, 2017.
[36] R. Zhou, Q. Luo, X. Feng, and C. Li, “Design of a wireless multi-channel surface EMG signal acquisition system”, 3rd IEEE International Conference on Computer and Communications (ICCC), Chengdu, pp. 279-283.IEEE,2017.
[37] M. Gandolla, S. Ferrante, G. Ferrigno, D. Baldassini, F. Molteni, E. Guanziroli, M. Cotti Cottini, C. Seneci and A. Pedrocchi, “Artificial neural network EMG classifier for functional hand grasp movements prediction”, Journal of International Medical Research, Vol. 45, No. 6, pp.1831-1847,2017.
[38] Y. Paul, V. Goyal, and R. A. Jaswal, “Comparative analysis between SVM & KNN classifier for EMG signal classification on elementary time domain features”, 4th International Conference on Signal Processing, Computing and Control (ISPCC), Solan, pp. 169-175,2017.
[39] N. Srisuwan, P. P. Limsakul, “Comparison of feature evaluation criteria for speech recognition based on electromyography”, Medical and Biological Engineering and Computing Vol 56,no.6, pp. 1-11, 2017.
[40] N. Sengar, M. K. Dutta and R. Burget, “Detection of the neuromuscular disease using EMG signals in wavelet domain”, 4th IEEE Uttar Pradesh Section International Conference on Electrical, Computer and Electronics (UPCON), Mathura, pp. 624-627.2017.
[41] N. S. Jong, M. Kiatweerasakul and P. Phukpattaranont, “Channel Reduction in Speech Recognition System based on Surface Electromyography”, 15th International Conference on Electrical Engineering/Electronics, Computer, Telecommunications and Information Technology (ECTI-CON), Chiang Rai, Thailand, pp. 184-187.IEEE,2018.
[42] Y. Tsai and J. Ko, “Implementation of a portable multi-channel EMG signal detection system for Android-based smartphones by using USB-OTG interface”, 2018 IEEE International Conference on Applied System Invention (ICASI), Chiba, pp. 766-769.IEEE,2018.
[43] M. Z. Jamal and K. Kim, “A finely machined toothed silver electrode surface for the improved acquisition of EMG signals”, IEEE Sensors Applications Symposium (SAS), Seoul, pp. 1-5.IEEE,2018.
[44] A. T. Koçak and A. Yilmaz, “Segmentation and classification of contractions in uterine 16 channels EMG signals for preterm birth prediction”, 26th Signal Processing and Communications Applications Conference (SIU), Izmir, pp. 1-4.IEEEs, 2018.
[45] G. Borelli, J. Jovic Bonnet, Y. Rosales Hernandez, K. Matsuda and J. Damerau, “Spectral-Distance-Based Detection of EMG Activity From Capacitive Measurements”, IEEE Sensors Journal, Vol. 18, No. 20, pp. 8502-8509, 15 Oct.15, 2018.
[46] L. Liao, Y. Tseng, H. Chiang, and W. Wang, “EMG-based Control Scheme with SVM Classifier for Assistive Robot Arm”, International Automatic Control Conference (CACS), Taoyuan, pp. 1-5.IEEE,2018.
[47] M. Ghofrani Jahromi, H. Parsaei, A. Zamani and D. W. Stashuk, “Cross-Comparison of Motor Unit Potential Features Used in EMG Signal Decomposition”, IEEE Transactions on Neural Systems and Rehabilitation Engineering, Vol. 26, No. 5, pp. 1017-1025, 2018.
[48] S. Pancholi and A. M. Joshi, “Portable EMG Data Acquisition Module for Upper Limb Prosthesis Application", IEEE Sensors Journal, Vol. 18, No. 8, pp. 3436-3443, 2018.
[49] J. L. Betthauser, C. L. Hunt,L. E. Osborn,M. R. Masters,G. Lévay,R. R. Kaliki and N. V. Thakor, “Limb Position Tolerant Pattern Recognition for Myoelectric Prosthesis Control with Adaptive Sparse Representations From Extreme Learning”, IEEE Transactions on Biomedical Engineering, Vol. 65, No. 4, pp. 770-778, 2018.
[50] O. W. SamuelZhouHui, L. Xiangxin, W. Hui, Z. Haoshi, A. K.Sangaiah and L.Guanglin, “Pattern recognition of electromyography signals based on novel time-domain features for amputees` limb motion classification”, Computers & Electrical Engineering, Vol.67,pp. 646-655,2018.
[51] M. Jochumsena, A. Waris, E. N. Kamavuako, “The effect of arm position on the classification of hand gestures with intramuscular EMG”, Biomedical Signal Processing and Control, Vol. 43,pp.1-8, 2018.
[52] P. Xia , J. Hu, and Y. Peng, “EMG-Based Estimation of Limb Movement Using Deep Learning With Recurrent Convolutional Neural Networks”, Artificial Organs, Vol. 42, No. 5, pp. E67-E77,2018.
[53] A. Waris, I. Khan Niazi, M. Jamil, O. Gilani, K. Englehart, W. Jensen, M. Shafique, and E. N. Kamavuako, “The Effect of Time on EMG classification of hand motions in able-bodied and transradial amputees”, Journal of Electromyography and Kinesiology, Vol. 40, pp. 72-80,2018.
[54] I.Vujaklija, V. Shalchyan, E. N. Kamavuako, N. Jiang, H. R. Marateb and D. Farina, “Online mapping of EMG signals into kinematics by autoencoding”, Journal of NeuroEngineering and Rehabilitation, Vol. 15, No.1, pp.1-9, 2018.
[55] M.Z. U. Rehman, A. Waris, S. O. Gilani, M. Jochumsen, I. K. Niazi, M. Jamil, D. Farina, and E. N.Kamavuako, “Multiday EMG-Based Classification of Hand Motions with Deep Learning Techniques”, Sensors, Vol. 18, No. 8, pp.1-16,2018.
[56] Matthew W.Flood, Bente Rona Jensen, Anne-Sofie Malling, and Madeleine M.Lowery, “Increased EMG intermuscular coherence and reduced signal complexity in Parkinson’s disease”, Clinical Neurophysiology, Vol. 130, No. 2, pp. 259-269,2019.
[57] Andras Hegyi, Daniel Csala, Annamária Peter, Taija Finni, and Neil J Cronin, “High‐density electromyography activity in various hamstring exercises”, Scandinavian journal of medicine & science in sports, Vol.29, No. 1, pp. 34-43,2019.
[58] N.Nazmia,Mohd. A.A Rahman, S.I.Yamamoto and S.Anom Ahmad, “Walking gait event detection based on electromyography signals using artificial neural network”, Biomedical Signal Processing and Control, Vol. 47, pp. 334-343,2019.