Deep learning aiding Health Informatics in Drug discovery
Malvika Jasrotia1 , Prabhpreet Kaur2
Section:Research Paper, Product Type: Journal Paper
Volume-7 ,
Issue-5 , Page no. 315-320, May-2019
CrossRef-DOI: https://doi.org/10.26438/ijcse/v7i5.315320
Online published on May 31, 2019
Copyright © Malvika Jasrotia, Prabhpreet Kaur . This is an open access article distributed under the Creative Commons Attribution License, which permits unrestricted use, distribution, and reproduction in any medium, provided the original work is properly cited.
View this paper at Google Scholar | DPI Digital Library
How to Cite this Paper
- IEEE Citation
- MLA Citation
- APA Citation
- BibTex Citation
- RIS Citation
IEEE Citation
IEEE Style Citation: Malvika Jasrotia, Prabhpreet Kaur, “Deep learning aiding Health Informatics in Drug discovery,” International Journal of Computer Sciences and Engineering, Vol.7, Issue.5, pp.315-320, 2019.
MLA Citation
MLA Style Citation: Malvika Jasrotia, Prabhpreet Kaur "Deep learning aiding Health Informatics in Drug discovery." International Journal of Computer Sciences and Engineering 7.5 (2019): 315-320.
APA Citation
APA Style Citation: Malvika Jasrotia, Prabhpreet Kaur, (2019). Deep learning aiding Health Informatics in Drug discovery. International Journal of Computer Sciences and Engineering, 7(5), 315-320.
BibTex Citation
BibTex Style Citation:
@article{Jasrotia_2019,
author = {Malvika Jasrotia, Prabhpreet Kaur},
title = {Deep learning aiding Health Informatics in Drug discovery},
journal = {International Journal of Computer Sciences and Engineering},
issue_date = {5 2019},
volume = {7},
Issue = {5},
month = {5},
year = {2019},
issn = {2347-2693},
pages = {315-320},
url = {https://www.ijcseonline.org/full_paper_view.php?paper_id=4242},
doi = {https://doi.org/10.26438/ijcse/v7i5.315320}
publisher = {IJCSE, Indore, INDIA},
}
RIS Citation
RIS Style Citation:
TY - JOUR
DO = {https://doi.org/10.26438/ijcse/v7i5.315320}
UR - https://www.ijcseonline.org/full_paper_view.php?paper_id=4242
TI - Deep learning aiding Health Informatics in Drug discovery
T2 - International Journal of Computer Sciences and Engineering
AU - Malvika Jasrotia, Prabhpreet Kaur
PY - 2019
DA - 2019/05/31
PB - IJCSE, Indore, INDIA
SP - 315-320
IS - 5
VL - 7
SN - 2347-2693
ER -
![]() |
![]() |
![]() |
414 | 310 downloads | 189 downloads |
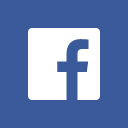
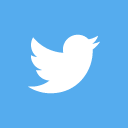
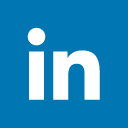
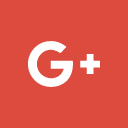
Abstract
The changes that occur are exciting and more challenging in our industry. There has been a massive increase in the amount of data in health informatics in the last decade. Over the years, deep learning is raising with its extraordinary success in the research areas of artificial intelligence. If we form larger neural network and then we train it with more available data and fast enough computers their performance then continues to arise. Earlier, machine-learning tools such as QSAR (quantitative structure-activity relationship) modeling have been used to identify potential biological active molecules from millions candidate compounds for drug discovery. But, in this era of big data machine learning approaches lack efficiency. Hence, deep learning evolved as a solution to the problem of big data. In this paper we discuss various deep learning approaches studied for various applications of health informatics with special reference to drug discovery.
Key-Words / Index Term
Deep learning, Deep neural network, Convolutional neural network, Recurrent neural network, Deep Autoencoder, Health informatics
References
[1] Jacobson Ralph (2013, April 24) 2.5 quintillion bytes of data created every day. How does CPG & Retail manage it? https://www.ibm.com/blogs/insights-on-business/consumer-products/2-5- quintillion-bytes-of-data-created-every-day-how-does-cpg-retail-manage-it/ (accessed on august 15, 2018)
[2] Goodfellow, I., Bengio, Y., Courville, A., &Bengio, Y. (2016). Deep learning (Vol. 1). Cambridge: MIT press.
[3] Yao, X. J., Panaye, A., Doucet, J. P., Zhang, R. S., Chen, H. F., Liu, M. C., ... & Fan, B. T. (2004). Comparative study of QSAR/QSPR correlations using support vector machines, radial basis function neural networks, and multiple linear regression. Journal of chemical information and computer sciences, 44(4), 1257-1266.
[4] https://en.wikipedia.org/wiki/Deep_learning (accessed on august 15, 2018)
[5] Bilal AfaanArtificial Neural Networks and Deep Learning. Retrieved from https://becominghuman.ai/artificial-neural-networks-and-deep-learning-a3c9136f2137 (accessed on august 15, 2018)
[6] King Paul (2016, April 17) https://www.quora.com/How-do-artificial-neural-networks-work (accessed on august 15, 2018)
[7] Ruder, S. (2016). An overview of gradient descent optimization algorithms. arXiv preprint arXiv:1609.04747.
[8] Chen, H., Engkvist, O., Wang, Y., Olivecrona, M., &Blaschke, T. (2018). The rise of deep learning in drug discovery. Drug discovery today.
[9] Cortes, C. and Vapnik, V. (1995) Support-vector networks. Mach. Learn. 20, 273–297
[10] Ho, T.K. (1998) The random subspace method for constructing decision forests. IEEE Trans. Pattern Anal. Mach. Intell. 20, 832–844
[11] Schmidhuber, J. (2015). Deep learning in neural networks: An overview. Neural networks, 61, 85-117.
[12] Lee, H., Grosse, R., Ranganath, R., & Ng, A. Y. (2011). Unsupervised learning of hierarchical representations with convolutional deep belief networks. Communications of the ACM, 54(10), 95-103.
[13] Fernández, S., Graves, A., &Schmidhuber, J. (2007). An application of recurrent neural networks to discriminative keyword spotting. In International Conference on Artificial Neural Networks (pp. 220-229). Springer, Berlin, Heidelberg.
[14] Sak, H., Senior, A., &Beaufays, F. (2014). Long short-term memory recurrent neural network architectures for large scale acoustic modeling. In Fifteenth annual conference of the international speech communication association.
[15] Bengio, Y. (2009) Learning deep architectures for AI. Found. Trends Mach. Learn. 2, 1–127
[16] Krizhevsky, A., & Hinton, G. E. (2011, April). Using very deep autoencoders for content-based image retrieval. In ESANN.
[17] Ravı, D., Wong, C., Deligianni, F., Berthelot, M., Andreu-Perez, J., Lo, B., & Yang, G. Z. (2017). Deep learning for health informatics. IEEE journal of biomedical and health informatics, 21(1), 4-21.
[18] Y. LeCun, L. Bottou, Y. Bengio, and P. Haffner, “Gradient-based learning applied to document recognition,” Proc. IEEE, vol. 86, no. 11,pp. 2278–2324, Nov. 1998.
[19] D. H. Hubel and T. N.Wiesel, “Receptive fields, binocular interaction and functional architecture in the cat’s visual cortex,” J. Physiol., vol. 160, no. 1, pp. 106–154, 1962.
[20] A. Krizhevsky, I. Sutskever, and G. E. Hinton, “Imagenet classification with deep convolutional neural networks,” in Proc. Adv. Neural Inf.Process. Syst., 2012, pp. 1097–1105.
[21]M. D. Zeiler and R. Fergus, “Visualizing and understanding convolutional networks,” in Proc. Eur. Conf. Comput. Vision, 2014, pp. 818–833.
[22] C. Szegedyet al., “Going deeper with convolutions,” in Proc. Conf. Comput. Vis. Pattern Recognit., 2015, pp. 1–9.
[23] R. J. Williams and D. Zipser, “A learning algorithm for continually running fully recurrent neural networks,” Neural Comput., vol. 1, no. 2, pp. 270–280, 1989
[24] Y. Bengio, P. Simard, and P. Frasconi, “Learning long-term dependencies with gradient descent is difficult,” IEEE Trans. Neural Netw., vol. 5, no. 2,pp. 157–166, Mar. 1994.
[25] G. E. Hinton and R. R. Salakhutdinov, “Reducing the dimensionality of data with neural networks,” Science, vol. 313, no. 5786, pp. 504–507, 2006.
[26] C. Poultney et al., “Efficient learning of sparse representations with an energy-based model,” in Proc. Adv. Neural Inf. Process. Syst., 2006, pp. 1137–1144.
[27] P. Vincent, H. Larochelle, Y. Bengio, and P.-A. Manzagol, “Extracting and composing robust features with denoising autoencoders,” in Proc. Int. Conf. Mach. Learn., 2008, pp. 1096–1103.
[28] S. Rifai, P. Vincent, X. Muller, X. Glorot, and Y. Bengio, “Contractive auto-encoders: Explicit invariance during feature extraction,” in Proc.Int. Conf. Mach. Learn., 2011, pp. 833–840.
[29] J. Masci, U. Meier, D. Cires¸an, and J. Schmidhuber, “Stacked convolutional auto-encoders for hierarchical feature extraction,” in Proc. Int.Conf. Artif. Neural Netw., 2011, pp. 52–59.
[30] J. Ngiam, A. Coates, A. Lahiri, B. Prochnow, Q. V. Le, and A. Y. Ng, “On optimization methods for deep learning,” in Proc. Int. Conf. Mach. Learn., 2011, pp. 265–272.
[31] P. Domingos, “A few useful things to know about machine learning,” Commun. ACM, vol. 55, no. 10, pp. 78–87, 2012.
[32] V. N. Vapnik, “An overview of statistical learning theory,” IEEE Trans.NeuralNetw., vol. 10, no. 5, pp. 988–999, Sep. 1999.
[33] C. M. Bishop, “Pattern recognition,” Mach. Learn., vol. 128, pp. 1–737, 2006
[34] R. Fakoor, F. Ladhak, A. Nazi, and M. Huber, “Using deep learning to enhance cancer diagnosis and classification,” in Proc. Int. Conf. Mach. Learn., 2013, pp. 1–7.
[35] D. Quang, Y. Chen, and X. Xie, “Dann: A deep learning approach for annotating the pathogenicity of genetic variants,” Bioinformatics, vol. 31, p. 761–763, 2014.
[36] C. Angermueller, H. Lee, W. Reik, and O. Stegle, “Accurate prediction of single-cell dna methylation states using deep learning,” bioRxiv, 2016, Art. no. 055715.
[37] B. Ramsundar, S. Kearnes, P. Riley, D. Webster, D. Konerding, and V. Pande, “Massively multitask networks for drug discovery,” ArXiv e-prints, Feb. 2015
[38] D. Nie, H. Zhang, E. Adeli, L. Liu, and D. Shen, “3d deep learningfor multi-modal imaging-guided survival time prediction of brain tumorpatients,” in Proc. MICCAI, 2016, pp. 212–220. [Online]. Available:http://dx.doi.org/10.1007/978-3-319-46723-8_25
[39] J. Kleesieket al., “Deep MRI brain extraction: A 3D convolutionalneural network for skull stripping,” NeuroImage, vol. 129, pp. 460–469,2016.
[40] B. Jiang, X. Wang, J. Luo, X. Zhang, Y. Xiong, and H. Pang, “Convolutionalneural networks in automatic recognition of trans-differentiatedneural progenitor cells under bright-field microscopy,” in Proc. Instrum.Meas., Comput., Commun. Control, 2015, pp. 122–126.
[41] H.-I. Suk et al., “Hierarchical feature representation and multimodal fusion with deep learning for ad/mci diagnosis,” NeuroImage, vol. 101, pp. 569– 582, 2014.
[42] D. C. Rose, I. Arel, T. P. Karnowski, and V. C. Paquit, “Applying deeplayered clustering to mammography image analytics,” in Proc. Biomed.Sci. Eng. Conf., 2010, pp. 1–4.
[43] Y. Zhou and Y. Wei, “Learning hierarchical spectral-spatial features for hyperspectral image classification,” IEEE Trans. Cybern., vol. 46, no. 7, pp. 1667–1678, Jul. 2016.
[44] L. Sun, K. Jia, T.-H. Chan, Y. Fang, G. Wang, and S. Yan, “DL-SFA: Deeply-learned slow feature analysis for action recognition,” in Proc. IEEE Conf. Comput. Vis. Pattern Recognit., 2014, pp. 2625–2632.
[45] C.-D. Huang, C.-Y. Wang, and J.-C. Wang, “Human action recognition system for elderly and children care using three stream convnet,” in Proc. Int. Conf. Orange Technol., 2015, pp. 5–9.
[46] M. Zeng et al., “Convolutional neural networks for human activity recognition using mobile sensors,” in Proc. MobiCASE, Nov. 2014, pp. 197– 205. [Online]. Available: http://dx.doi.org/10.4108/icst. mobicase.2014.257786
[47] S. Ha, J. M. Yun, and S. Choi, “Multi-modal convolutional neural networks for activity recognition,” in Proc. Int. Conf. Syst., Man, Cybern., Oct. 2015, pp. 3017–3022.
[48] D. Ravi, C. Wong, B. Lo, and G. Z. Yang, “Deep learning for human activity recognition: A resource efficient implementation on low-power devices,” in Proc. 13th Int.Conf.Wearable Implantable Body Sens.Netw., Jun. 2016, pp. 71–76.
[49] P. Pouladzadeh, P. Kuhad, S. V. B. Peddi, A. Yassine, andS. Shirmohammadi, “Food calorie measurement using deep learningneural network,” in Proc. IEEE Int. Instrum. Meas. Technol. Conf. Proc.,2016, pp. 1–6.
[50] P. Kuhad, A. Yassine, and S. Shimohammadi, “Using distance estimationand deep learning to simplify calibration in food calorie measurement,”in Proc. IEEE Int. Conf. Comput. Intell. Virtual Environ. Meas. Syst.Appl., 2015, pp. 1–6.
[51] H. Shin, L. Lu, L. Kim, A. Seff, J. Yao, and R. M. Summers, “Interleaved text/image deep mining on a large-scale radiology database for automated image interpretation,” CoRR, vol. abs/1505.00670, 2015. [Online]. Available: http://arxiv.org/abs/1505.00670
[52] Z. Che, S. Purushotham, R. Khemani, and Y. Liu, “Distilling knowledge from deep networks with applications to healthcare domain,” ArXiv e-prints, Dec. 2015.
[53] R. Miotto, L. Li, B. A. Kidd, and J. T. Dudley, “Deep patient: An unsupervised representation to predict the future of patien
[54] E. Putin et al., “Deep biomarkers of human aging: Application of deep neural networks to biomarker development,” Aging, vol. 8, no. 5, pp. 1–021, 2016.
[55] J. Futoma, J. Morris, and J. Lucas, “A comparison of models for predicting early hospital readmissions,” J. Biomed. Informat., vol. 56, pp. 229–238, 2015.
[56] Z. C. Lipton, D. C. Kale, C. Elkan, and R. C. Wetzel, “Learning to diagnose with LSTM recurrent neural networks,” CoRR, vol. abs/1511.03677, 2015. [Online]. Available: http://arxiv.org/abs/1511.03677
[57] B. T. Ong, K. Sugiura, and K. Zettsu, “Dynamically pre-trained deep recurrent neural networks using environmental monitoring data for predicting pm2. 5,” Neural Comput. Appl., vol. 27, pp. 1–14, 2015.
[58] B. Zou, V. Lampos, R. Gorton, and I. J. Cox, “On infectious intestinal disease surveillance using social media content,” in Proc. 6th Int. Conf. Digit. Health Conf., 2016, pp. 157–161.
[59] V. R. K. Garimella, A. Alfayad, and I. Weber, “Social media image analysis for public health,” in Proc. CHIConf. Human Factors Comput.Syst., 2016, pp. 5543–5547. [Online]. Available: http://doi.acm. org/10.1145/2858036.2858234
[60] L. Zhao, J. Chen, F. Chen, W. Wang, C.-T. Lu, and N. Ramakrishnan, “Simnest: Social media nested epidemic simulation via online semisupervised deep learning,” in Proc. IEEE Int. Conf. Data Mining, 2015, pp. 639–648.
[61] E. Horvitz and D. Mulligan, “Data, privacy, and the greater good,” Science, vol. 349, no. 6245, pp. 253–255, 2015.
[62] Molloy, C. (2017). Drug discovery tomorrow: how to Catapult ourselves into the future.
[63] Zhang, L., Tan, J., Han, D., & Zhu, H. (2017). From machine learning to deep learning: progress in machine intelligence for rational drug discovery. Drug discovery today.
[64] Miotto, R., Wang, F., Wang, S., Jiang, X., & Dudley, J. T. (2017). Deep learning for healthcare: review, opportunities and challenges. Briefings in bioinformatics.
[65] Xu, Y., Dai, Z., Chen, F., Gao, S., Pei, J., & Lai, L. (2015). Deep learning for drug-induced liver injury. Journal of chemical information and modeling, 55(10), 2085-2093.
[66] Altae-Tran, H., Ramsundar, B., Pappu, A. S., & Pande, V. (2017). Low data drug discovery with one-shot learning. ACS central science, 3(4), 283-293.
[67] Olivecrona, M., Blaschke, T., Engkvist, O., & Chen, H. (2017). Molecular de-novo design through deep reinforcement learning. Journal of cheminformatics, 9(1), 48.
[68] Wallach, I., Dzamba, M., & Heifets, A. (2015). AtomNet: A deep convolutional neural network for bioactivity prediction in structure-based drug discovery. arXiv preprint arXiv:1510.02855.
[69] Byvatov, E., Fechner, U., Sadowski, J., & Schneider, G. (2003). Comparison of support vector machine and artificial neural network systems for drug/nondrug classification. Journal of chemical information and computer sciences, 43(6), 1882-1889.