Integrated User Profiles for Effective Mining in Complex Online Systems
A. K. Shingarwade1 , P. N. Mulkalwar2
Section:Research Paper, Product Type: Journal Paper
Volume-7 ,
Issue-5 , Page no. 153-159, May-2019
CrossRef-DOI: https://doi.org/10.26438/ijcse/v7i5.153159
Online published on May 31, 2019
Copyright © A. K. Shingarwade, P. N. Mulkalwar . This is an open access article distributed under the Creative Commons Attribution License, which permits unrestricted use, distribution, and reproduction in any medium, provided the original work is properly cited.
View this paper at Google Scholar | DPI Digital Library
How to Cite this Paper
- IEEE Citation
- MLA Citation
- APA Citation
- BibTex Citation
- RIS Citation
IEEE Style Citation: A. K. Shingarwade, P. N. Mulkalwar, “Integrated User Profiles for Effective Mining in Complex Online Systems,” International Journal of Computer Sciences and Engineering, Vol.7, Issue.5, pp.153-159, 2019.
MLA Style Citation: A. K. Shingarwade, P. N. Mulkalwar "Integrated User Profiles for Effective Mining in Complex Online Systems." International Journal of Computer Sciences and Engineering 7.5 (2019): 153-159.
APA Style Citation: A. K. Shingarwade, P. N. Mulkalwar, (2019). Integrated User Profiles for Effective Mining in Complex Online Systems. International Journal of Computer Sciences and Engineering, 7(5), 153-159.
BibTex Style Citation:
@article{Shingarwade_2019,
author = {A. K. Shingarwade, P. N. Mulkalwar},
title = {Integrated User Profiles for Effective Mining in Complex Online Systems},
journal = {International Journal of Computer Sciences and Engineering},
issue_date = {5 2019},
volume = {7},
Issue = {5},
month = {5},
year = {2019},
issn = {2347-2693},
pages = {153-159},
url = {https://www.ijcseonline.org/full_paper_view.php?paper_id=4214},
doi = {https://doi.org/10.26438/ijcse/v7i5.153159}
publisher = {IJCSE, Indore, INDIA},
}
RIS Style Citation:
TY - JOUR
DO = {https://doi.org/10.26438/ijcse/v7i5.153159}
UR - https://www.ijcseonline.org/full_paper_view.php?paper_id=4214
TI - Integrated User Profiles for Effective Mining in Complex Online Systems
T2 - International Journal of Computer Sciences and Engineering
AU - A. K. Shingarwade, P. N. Mulkalwar
PY - 2019
DA - 2019/05/31
PB - IJCSE, Indore, INDIA
SP - 153-159
IS - 5
VL - 7
SN - 2347-2693
ER -
![]() |
![]() |
![]() |
375 | 294 downloads | 192 downloads |
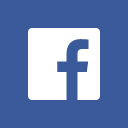
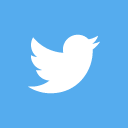
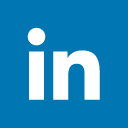
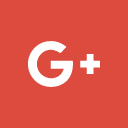
Abstract
User profiles include but are not limited to social media profile, professional online profile, e-commerce profile and search profile. Each individual user nowadays has multiple user profiles, due to the fact that these users are constantly using online and offline services. These profiles are not mutually exclusive as the search habits of a user directly showcase the user`s shopping behaviour, and so on. Due to the presence of so many profiles of a single entity, there is a wide research area which has opened up in the recent years. Companies and researchers are harnessing this gap in order to provide better user experience via integrating multiple profiles and helping them to learn from one another. In this paper, we define a framework via which the user`s social and e-commerce profiles can be combined in order to better recommend their buying patterns to companies based on the items purchased by the friends which the user`s follow closely. Mining positive and negative rules (MOPNAR), firefly, top k rules and association rule mining is used in order to mine the usage patterns, and the results shows that an accuracy of more than 70% is observed when compared with the real time buying patterns.
Key-Words / Index Term
Profile, integrated, online, MOPNAR, firefly, e-commerce, social
References
[1] X. Tao, Y. Li, and N. Zhong, "A customized philosophy display for web data gathering," IEEE Transactions on Knowledge and Data Engineering, Vol. 23, No. 4, pp. 496– 511, April 2011.
[2] K. L. Skillen, L. Chen, C. D. Nugent, M. P. Donnelly, W. Burns, and I. Solheim, "Ontological client demonstrating and semantic standard based thinking for personalisation of assistance on-request benefits in inescapable conditions," Future Generation Computer Systems, Vol. 34, pp. 97 – 109, 2014, Special Section: Distributed Solutions for Ubiquitous Computing and Ambient Intelligence.
[3] L. Zhao, R. Ichise, "Cosmology reconciliation for connected information," Journal on Data Semantics, Vol. 3, No. 4, pp. 237– 254, 2014.
[4] A. Hawalah, M. Fasli, "Dynamic client profiles for web personalisation," Expert Systems with Applications, Vol. 42, No. 5, pp. 2547 – 2569, 2015.
[5] D. Le-Phuoc, H. Nguyen Mau Quoc, Hung Ngo Quoc, T. Tran Nhat, and M. Hauswirth, "The chart of things: A stage towards the live learning diagram of associated things," Web Semantics: Science, Services and Agents on the World Wide Web, Vol. 3738, pp. 25 – 35, 2016.
[6] J. Wang, Yi Zhang, "Opportunity demonstrate for internet business proposal: Right item; ideal time," Proceedings of the 36th International ACM SIGIR Conference on Research and Development in Information Retrieval, New York, NY, USA, SIGIR `13, pp. 303– 312, ACM, 2013.
[7] K. Christidis, G. Mentzas, "A subject based recommender framework for electronic commercial center stages," Expert Systems with Applications, Vol. 40, No. 11, pp. 4370 – 4379, 2013.
[8] J. Lu, Dianshuang Wu, M. Mao, Wei Wang, and G. Zhang, "Recommender framework application advancements: A study," Decision Support Systems, Vol. 74, pp. 12 – 32, 2015.
[9] N. Manouselis, H. Drachsler, R. Vuorikari, H. Hummel, and R. Koper, Recommender Systems in Technology Enhanced Learning, pp. 387– 415, Springer US, Boston, MA, 2011.
[10] A. Klanja-Milievi, B. Vesin, M. Ivanovi, and Z. Budimac, "E-learning personalization dependent on half breed proposal methodology and learning style distinguishing proof," Computers and Education, Vol. 56, No. 3, pp. 885 – 899, 2011.
[11] D. Mladeni, M. Grar and M. Grobelnik, "Client profiling for intrigue centered perusing history," Proceedings of the SKIDD, ACM, 2005.
[12] A. K. Shingarwade, P. N. Mulkalwar, “Reducing Cluster Formation Delay for Real Time Data using Automatic Bisecting Hierarchical Clustering”, Proceeding of Third National Conference on “Recent Advances in Science and Engineering” (NC-RACE 18), pp 1-8, Oct 2018.
[13] D. Martn, A. Rosete, J. Alcala-Fdez,and F. Herrera, “A New Multiobjective Evolutionary Algorithm for Mining a Reduced Set of Interesting Positive and Negative Quantitative Association Rules”, IEEE Trans. Evol. Computing, Vol. 18, NO. 1, Feb 2014.
[14] A. K. Shingarwade, P. N. Mulkalwar, “Development of Top K Rules For Association Rule Mining on E-Commerce Dataset”, Proceeding of Global Journal of Engineering Science & Researches, pp 304-309, Feb 2019.
[15] X. S. Yang, “Nature-Inspired Metaheuristic Algorithms”, Luniver Press, 2008.
[16] X. S. Yang, “Firefly algorithms for multimodal optimisation”, Proc. 5th Symposium on Stochastic Algorithms, Foundations and Applications, (Eds. O. Watanabe and T. Zeugmann), Lecture Notes in Computer Science, 5792: 169-178, 2009.
[17] A. K. Shingarwade, P. N. Mulkalwar, “Study of Text Content Mining for E-Commerce Web Sites” International Journal of Advanced Research in Computer Science, Vol 8, No. 4, May – June 2017.
[18] A. K. Shingarwade, P. N. Mulkalwar, “Comparative Analysis of Clustering Techniques Based on Validity Measures”, Proceedings of the International Conference on Recent Trends in Science & Technology ICRTST Special Issue No. 25, pp 139-144, 2018.