Diagnosis of Dyslexia Students Using Classification Mining Techniques
H. Selvi1 , M.S. Saravanan2
Section:Research Paper, Product Type: Journal Paper
Volume-7 ,
Issue-5 , Page no. 28-33, May-2019
CrossRef-DOI: https://doi.org/10.26438/ijcse/v7i5.2833
Online published on May 31, 2019
Copyright © H. Selvi, M.S. Saravanan . This is an open access article distributed under the Creative Commons Attribution License, which permits unrestricted use, distribution, and reproduction in any medium, provided the original work is properly cited.
View this paper at Google Scholar | DPI Digital Library
How to Cite this Paper
- IEEE Citation
- MLA Citation
- APA Citation
- BibTex Citation
- RIS Citation
IEEE Style Citation: H. Selvi, M.S. Saravanan, “Diagnosis of Dyslexia Students Using Classification Mining Techniques,” International Journal of Computer Sciences and Engineering, Vol.7, Issue.5, pp.28-33, 2019.
MLA Style Citation: H. Selvi, M.S. Saravanan "Diagnosis of Dyslexia Students Using Classification Mining Techniques." International Journal of Computer Sciences and Engineering 7.5 (2019): 28-33.
APA Style Citation: H. Selvi, M.S. Saravanan, (2019). Diagnosis of Dyslexia Students Using Classification Mining Techniques. International Journal of Computer Sciences and Engineering, 7(5), 28-33.
BibTex Style Citation:
@article{Selvi_2019,
author = {H. Selvi, M.S. Saravanan},
title = {Diagnosis of Dyslexia Students Using Classification Mining Techniques},
journal = {International Journal of Computer Sciences and Engineering},
issue_date = {5 2019},
volume = {7},
Issue = {5},
month = {5},
year = {2019},
issn = {2347-2693},
pages = {28-33},
url = {https://www.ijcseonline.org/full_paper_view.php?paper_id=4193},
doi = {https://doi.org/10.26438/ijcse/v7i5.2833}
publisher = {IJCSE, Indore, INDIA},
}
RIS Style Citation:
TY - JOUR
DO = {https://doi.org/10.26438/ijcse/v7i5.2833}
UR - https://www.ijcseonline.org/full_paper_view.php?paper_id=4193
TI - Diagnosis of Dyslexia Students Using Classification Mining Techniques
T2 - International Journal of Computer Sciences and Engineering
AU - H. Selvi, M.S. Saravanan
PY - 2019
DA - 2019/05/31
PB - IJCSE, Indore, INDIA
SP - 28-33
IS - 5
VL - 7
SN - 2347-2693
ER -
![]() |
![]() |
![]() |
701 | 645 downloads | 269 downloads |
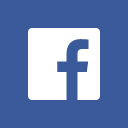
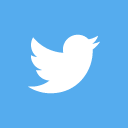
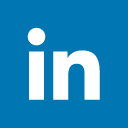
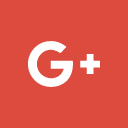
Abstract
Now a day, all over the world 70-80% of people with poor reading skills are likely dyslexic. One in five a student, or 15-20% of the population, has a language based learning disability. Dyslexia is the most common of the language based learning disabilities. Nearly the same percentage of males and females has dyslexia. Children suffering from a learning disability might face difficulties with reading, writing or mathematics but they excel in other areas of interests. It is in the interest of the society and especially the parents to identify the problem early in the development of the child and steer him/her towards a preferred field. They might lose their sense of self-worth and blame themselves for their situation. The model being proposed is a Web-based tool incorporating machine learning techniques (Decision trees) for predicting whether children (8-10 years) are at a risk of having Specific Learning Disability by showing the areas of learning disability on the basis of the clinical information and research.
Key-Words / Index Term
Dyslexia, Weka, SVM, Naïve Bayes, J48 Decision Tree, Neural Network
References
[1] Disability World, 2003,”UNICEF and Disabled Children and Youths”, Disability World, No. 19, (online at www.disabil ity-world.org/ 06 − 08 03/index.htm).
[2] Athanasios S. Drigas and Rodi-Eleni Ioannidou,”A Review on Arti-ficial Intelligence in Special Education”, Ag.Paraskevi, 15310, Athens, Greece, 2013.
[3] Julie M. David, Kannan Balakrishnan: Machine Learning Approach for Prediction of Learning Disabilities in School Age Children, Int. J. of Computer Applications, ISSN-0975-8887, 9(10), Nov. 2010, pp 7-14. http://www.ijcaonline.org/archives/volume9/ number 1/1432-1931.
[4] Manghirmalani et al, “Learning Disability Diagnosis and Classifi-cation-a Soft Computing Approach”, IEEE World Congress on In-formation and Communication Technologies (WICT); https://doi.org/10.1109/WICT.2011.6141292.
[5] Kohli, M., Prasad, T.V, “Identifying Dyslexic Students by Using Artificial Neural Networks”, Proceedings of the World Congress on Engineering, London, U.K, vol. 1(2010).
[6] Anuradha, J et al, “Diagnosis of ADHD using SVM algorithm”, Proceedings of the Third Annual ACM Bangalore Conference (2010) https://doi.org/10.1145/1754288.1754317.
[7] Hernadez, J et al, “Learning Difficulties Diagnosis for Children’s Basic Education using Expert Systems”, WSEAS Transactions on Information Science and Applications (2009).
[8] Jain et al, “Computational Diagnosis of Learning Disability”, Inter-national Journal of Recent Trends in Engineering (2009).
[9] Arthi. K and Tamilarasi, A, ”Prediction of autistic disorder using neuro fuzzy system by applying ANN technique”, International Journal of Developmental Neuroscience 26, 699–704 (2008).
[10] Lineu C. Fonseca et al, “Quantitative EEG in children with learning disabilities”, Analysis of band power, 64(2-B):376-381, 2006.
[11] Martin Macas et al, “Bioinspired methods for analysis and classifi-cation of reading eye movements of dyslexic children”, Department of Cybernetics, Czech Technical University in Prague, Czech Re-public NiSls Symposium 2005.
[12] Md. Mustafizur Rahman, S. M. Ferdous, Syed Ishtiaque, “Increas-ing Intelligibility in the Speech of the Autistic Children by an Inter-active Computer Game”, Multimedia(ISM), pp 383 – 387, 2010.
[13] Joan F. Santos, NiritBrosh, Tiago H. Falk, Lonnie Zwaigenbaum, Susan E. Bryson, Wendy Roberts, Isabel M. Smith, Peter Szatmari and Jessica A. Brian, “Very early detection of autism spectrum dis-orders based on acoustic analysis of pre-verbal vocalizations of 18-month old toddlers”, International Conference on Acoustics, Speech and Signal Processing, pp 7567 – 7571, 2013.
[14] Suresh P. and Raja, B. K, 2011, “A Review on Analysis and Quan-tification of Specific Learning Disability (SLD) with fMRI using Image Processing Techniques”, IJCA Proceedings on International Conference on VLSI, Communications and Instrumentation (ICVCI) , vol. 5, pp. 24-29, Foundation of Computer Science.
[15] Prud’hommeaux et al., “Classification of atypical language inau-tism”, in Proceedings of the 2nd Workshop on Cognitive Modelin-gand Computational Linguistics, pp: 88-96, 2011.
[16] Kathleen T Quach et al., “Application of Artificial Neural Net-worksin Classification of Autism Diagnosis Based on Gene Expres-sionSignatures”.
[17] Alexander Genkin et al., “Large-scale Bayesian logistic regression fortext categorization”, Technometrics, pp: 291-304, 2007. RachnaAhuja et al, / (IJCSIT) International Journal of Computer Science and Information Technologies https://doi.org/10.1198/004017007000000245.
[18] Morris, R. D., 1988, “Classification of learning disabilities: Old problems and new approaches”, Journal of Consulting and Clinical Psychology, vol. 56, no. 6, pp.789-794. https://doi.org/10.1037/0022-006X.56.6.789.
[19] Folorunsho, Olaiya. "Comparative Study of Different Data Mining Techniques Performance in knowledge Discovery from Medical Database." International Journal 3, no. 3 (2013). International Journal of Engineering & Technology 3411
[20] Cohen, I.L., Sudhalter, V., Landong-Jimenez, D. and Keogh, M., 1993, “A Neural Network Approach to the Classification of Au-tism”, Journal of Autism and Developmental Disorders, vol. 23, no. 3, pp. 443-466. https://doi.org/10.1007/BF01046050.
[21] James Freeman and David Skapura, “Neural networks: Algorithms, applications and Programming Techniques”, Pearson Education, 2007.