Perspective Analysis of Voice Disorder Detection using various Approaches
P. Kokila1 , G. M. Nasira2
Section:Research Paper, Product Type: Journal Paper
Volume-7 ,
Issue-4 , Page no. 1208-1212, Apr-2019
CrossRef-DOI: https://doi.org/10.26438/ijcse/v7i4.12081212
Online published on Apr 30, 2019
Copyright © P. Kokila, G. M. Nasira . This is an open access article distributed under the Creative Commons Attribution License, which permits unrestricted use, distribution, and reproduction in any medium, provided the original work is properly cited.
View this paper at Google Scholar | DPI Digital Library
How to Cite this Paper
- IEEE Citation
- MLA Citation
- APA Citation
- BibTex Citation
- RIS Citation
IEEE Citation
IEEE Style Citation: P. Kokila, G. M. Nasira, “Perspective Analysis of Voice Disorder Detection using various Approaches,” International Journal of Computer Sciences and Engineering, Vol.7, Issue.4, pp.1208-1212, 2019.
MLA Citation
MLA Style Citation: P. Kokila, G. M. Nasira "Perspective Analysis of Voice Disorder Detection using various Approaches." International Journal of Computer Sciences and Engineering 7.4 (2019): 1208-1212.
APA Citation
APA Style Citation: P. Kokila, G. M. Nasira, (2019). Perspective Analysis of Voice Disorder Detection using various Approaches. International Journal of Computer Sciences and Engineering, 7(4), 1208-1212.
BibTex Citation
BibTex Style Citation:
@article{Kokila_2019,
author = {P. Kokila, G. M. Nasira},
title = {Perspective Analysis of Voice Disorder Detection using various Approaches},
journal = {International Journal of Computer Sciences and Engineering},
issue_date = {4 2019},
volume = {7},
Issue = {4},
month = {4},
year = {2019},
issn = {2347-2693},
pages = {1208-1212},
url = {https://www.ijcseonline.org/full_paper_view.php?paper_id=4188},
doi = {https://doi.org/10.26438/ijcse/v7i4.12081212}
publisher = {IJCSE, Indore, INDIA},
}
RIS Citation
RIS Style Citation:
TY - JOUR
DO = {https://doi.org/10.26438/ijcse/v7i4.12081212}
UR - https://www.ijcseonline.org/full_paper_view.php?paper_id=4188
TI - Perspective Analysis of Voice Disorder Detection using various Approaches
T2 - International Journal of Computer Sciences and Engineering
AU - P. Kokila, G. M. Nasira
PY - 2019
DA - 2019/04/30
PB - IJCSE, Indore, INDIA
SP - 1208-1212
IS - 4
VL - 7
SN - 2347-2693
ER -
![]() |
![]() |
![]() |
513 | 332 downloads | 254 downloads |
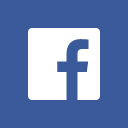
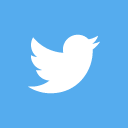
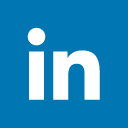
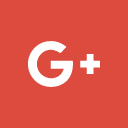
Abstract
Abstract— Automatic voice pathology detection and classification systems effectively contribute to the assessment of voice disorders, which helps clinicians to detect the existence of any voice pathologies and the type of pathology from which patients suffer in the early stage. This paper performs detailed study on various methodologies like feature extraction techniques, pattern recognition using machine learning, artificial intelligence, data mining, etc., used by various researches to detect the voice disorder using signal processing and voice recordings. The identification of an algorithm that discriminates between pathological and healthy voices with more accuracy is necessary to realize a valid and precise health system. The key contribution of this study is to investigate the performance of several machine learning techniques useful for voice pathology detection. This work provides detailed survey and comparison of the existing works pros and cons. This study also highlights the drawbacks in the existing methods and outlines the important factors to be considered while performing
Key-Words / Index Term
voice pathology, voice disorder, signal processing, machine learning, data mining and feature extraction
References
[1]. Davis B., “Acoustic Characteristics of Normal and Pathological Voices,” in Proceedings of Speech and Language: Advances in Basic Research and Practice, Orland, pp. 271-335, 1979.
[2]. Parsa V. and Jamieson G., “Interactions between Speech Coders and Disordered Speech,” Computer Journal of Speech Communication, vol. 40, no. 7, pp. 365-385, 2003.
[3]. Wang J. and Cheolwoo J., “Performance of Gaussian Mixture Model as a Classifier for Pathological Voice,” in Proceedings of the ASST in Auckland, Australian, pp. 165-169, 2006.
[4]. Yu P., Ouaknine M., Revis J., and Giovanni A., “Objective Voice Analysis for Dysphonic Patients: A Multiparametric Protocol Including Acoustic and Aerodynamic Measurements,” Computer Journal of Voice, vol. 15, no. 4, pp. 529-542, 2001
[5]. J. I. Godino-Llorente, P. G´omez-Vilda, N. S´aenz-Lech´on, M. Blanco Velasco, F. Cruz-Rold´an, and M. A. Ferrer-Ballester, “Support vector machines applied to the detection of voice disorders,” Lecture notes in computer science, vol. 3817, p. 219, 2005.
[6]. N. Souissi and A. Cherif, “Dimensionality reduction for voice disorders identification system based on mel frequency cepstral coefficients and support vector machine,” in Modelling, Identification and Control (ICMIC), 2015 7th International Conference on. IEEE, 2015, pp. 1–6.
[7]. W. Barry and M. P¨utzer, “Saarbrucken voice database,” Institute of Phonetics, Universit¨at des Saarlandes, http://www. stimmdatenbank.coli. uni-saarland. de, 2007.
[8]. M. El Emary, M. Fezari, and F. Amara, “Towards developing a voice pathologies detection system,” Journal of Communications Technology & Electronics, vol. 59, no. 11, p. 1280, 2014.
[9]. E. S. Fonseca, R. C. Guido, P. R. Scalassara, C. D. Maciel, and J. C. Pereira, “Wavelet time-frequency analysis and least squares support vector machines for the identification of voice disorders,” Computers in Biology and Medicine, vol. 37, no. 4, pp. 571–578, 2007.
[10]. J. Wang and C. Jo, “Vocal folds disorder detection using pattern recognition methods,” in Engineering in Medicine and Biology Society, 2007. EMBS 2007. 29th Annual International Conference of the IEEE. IEEE, pp. 3253–3256, 2007.
[11]. Henríquez, P., Alonso, J. B., Ferrer, M. A., Travieso, C. M., Godino-Llorente, J. I., Díaz-di-María, F. Characterization of Healthy and Pathological Voice Through Measures Based on Nonlinear Dynamics. IEEE Transactions on Audio, Speech, and Language Processing, Vol. 17, No. 6, 1186-1195, August 2009.
[12]. Forero, L. A., Kohler, M., Vellasco, M., Cataldo, E. Analysis and Classification of Voice Pathologies Using Glottal Signal Parameters. Journal of Voice, 30(5):549-556, 2015.
[13]. Markaki, M., Stylianou, Y. Voice Pathology Detection and Discrimination Based on Modulation Spectral Features. IEEE Transactions on Audio, Speech, and Language Processing, Vol. 19, No. 7, 1938-1948, 2011.
[14]. Panek, D., Skalski, A., Gajda, J., Tadeusiewicz, R. Acoustic Analysis Assessment in Speech Pathology Detection. Int. J. Appl. Math. Comput. Sci., 2015, Vol. 25, No. 3, 631–643.
[15]. Al-nasheri, A., Muhammad, G., Alsulaiman, M., Ali, Z. Investigation of Voice Pathology Detection and Classification on Different Frequency Regions Using Correlation Functions. Journal of Voice, 31(1):3-15, 2016.
[16]. Sellam, V., Jagadeesan, J. Classification of Normal and Pathological Voice Using SVM and RBFNN. Journal of Signal and Information Processing, 2014, 5, 1-7.
[17]. Hugo Cordeiro, Carlos Meneses Ribeiro, Speaker Characterization with MLSFs, The Journal for Nurse Practitioners. Elsevier, 2016
[18]. S. C. Punitha¸ N. A. Sheela Selvakumari, Voice Pathology Identification System using SVM Classifier, International Journal of Advance Research in Computer Science and Management Studies, Volume 5, Issue 1, January 2017
[19]. Laura Verde, Giuseppe De Pietro, Voice Disorder Identification by using Machine Learning Techniques, IEEE. Translations and content mining, pp 1-11, 2018.
[20]. Joao Paulo Teixeiraa, Paula Odete Fernandesa, Nuno Alvesa, Vocal Acoustic Analysis – Classification of Dysphonic Voices with Artificial Neural Networks, ScienceDirect, Procedia Computer Science 121 (2017) 19–26
[21]. Zhijian Wang, Ping Yu, Nan Yan, Lan Wang, Manwa L. Ng, Automatic Assessment of Pathological Voice Quality Using Multidimensional Acoustic Analysis Based on the GRBAS Scale, Sign Process Syst (82:241–251), 2016