Analysis the Breast Cancer using Back Propagation with Deep Neural Network
K. Anastraj1 , T. Chakravarthy2
Section:Research Paper, Product Type: Journal Paper
Volume-7 ,
Issue-4 , Page no. 844-847, Apr-2019
CrossRef-DOI: https://doi.org/10.26438/ijcse/v7i4.844847
Online published on Apr 30, 2019
Copyright © K. Anastraj, T. Chakravarthy . This is an open access article distributed under the Creative Commons Attribution License, which permits unrestricted use, distribution, and reproduction in any medium, provided the original work is properly cited.
View this paper at Google Scholar | DPI Digital Library
How to Cite this Paper
- IEEE Citation
- MLA Citation
- APA Citation
- BibTex Citation
- RIS Citation
IEEE Citation
IEEE Style Citation: K. Anastraj, T. Chakravarthy, “Analysis the Breast Cancer using Back Propagation with Deep Neural Network,” International Journal of Computer Sciences and Engineering, Vol.7, Issue.4, pp.844-847, 2019.
MLA Citation
MLA Style Citation: K. Anastraj, T. Chakravarthy "Analysis the Breast Cancer using Back Propagation with Deep Neural Network." International Journal of Computer Sciences and Engineering 7.4 (2019): 844-847.
APA Citation
APA Style Citation: K. Anastraj, T. Chakravarthy, (2019). Analysis the Breast Cancer using Back Propagation with Deep Neural Network. International Journal of Computer Sciences and Engineering, 7(4), 844-847.
BibTex Citation
BibTex Style Citation:
@article{Anastraj_2019,
author = { K. Anastraj, T. Chakravarthy},
title = {Analysis the Breast Cancer using Back Propagation with Deep Neural Network},
journal = {International Journal of Computer Sciences and Engineering},
issue_date = {4 2019},
volume = {7},
Issue = {4},
month = {4},
year = {2019},
issn = {2347-2693},
pages = {844-847},
url = {https://www.ijcseonline.org/full_paper_view.php?paper_id=4128},
doi = {https://doi.org/10.26438/ijcse/v7i4.844847}
publisher = {IJCSE, Indore, INDIA},
}
RIS Citation
RIS Style Citation:
TY - JOUR
DO = {https://doi.org/10.26438/ijcse/v7i4.844847}
UR - https://www.ijcseonline.org/full_paper_view.php?paper_id=4128
TI - Analysis the Breast Cancer using Back Propagation with Deep Neural Network
T2 - International Journal of Computer Sciences and Engineering
AU - K. Anastraj, T. Chakravarthy
PY - 2019
DA - 2019/04/30
PB - IJCSE, Indore, INDIA
SP - 844-847
IS - 4
VL - 7
SN - 2347-2693
ER -
![]() |
![]() |
![]() |
684 | 366 downloads | 136 downloads |
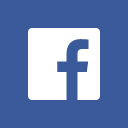
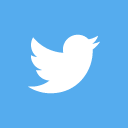
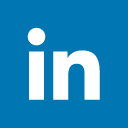
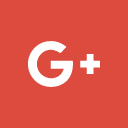
Abstract
Breast cancer is one of the leading diseases among the worldwide disease; the breast cancer is occur will both gender but it is very rare for man. The breast cancer is an unwanted tissue is growth on the breast. The survival rate has increased above 500,000 around the world. When detected early, the five-year continued existence rate for breast cancer exceeds 80% of cases. Early analysis of breast cancer is serious for the continued existence of the patient. It is formed the multiple cells which may it occur on benign and malignant. The malignant is a cluster of cells and it is irregular shape. The benign tumor is oval shaped and smooth surface. In our approach, the medical microwave imaging technique is an innovative technology for detecting cancer it is avoiding for the patient uncomfortable feelings and screening is very easy. It is analysis the tissue by using the radio-frequencies and differentiates either benign or malignant. The deep learning is an important role for bio-medical images, classification and gains the human approaches. The grey level co-occurrence matrix is a feature extraction to reduce the noise detection and apply the grey color for differentiate the cancerous tissue and non-cancerous tissue . The back propagation algorithm is trained the network randomly and minimized the error rate. For each classifier, the presentation factor such as sensitivity, specificity and accuracy are computed. It is observed that the proposed scheme with classifier outperforms specificity to classify microwave images as normal or abnormal.
Key-Words / Index Term
breast cancer, medical microwave images, grey level co-occurrence matrix(GLCM), Back propagation.
References
[1].Qi, H., & Diakides, N. A. (n.d.). Thermal infrared imaging in early breast cancer detection-a survey of recent research. Proceedings of the 25th Annual International Conference of the IEEE Engineering in Medicine and Biology Society (IEEE Cat. No.03CH37439). doi:10.1109/iembs.2003.1279442
[2]. Lehman CD, Schnall MD. Imaging in breast cancer: Magnetic resonance imaging. Breast Cancer Res. 2005
[3] Dan Ciresan, Alessandro Giusti, Luca M Gambardella, and J¨urgenSchmidhuber, “Deep neural networks segment neuronal membranes in electron microscopy images,” In Advances in neural information processing systems, vol 2012, pp. 2843–2851
[4].Avril N, Mather SJ, Roylance R. FDG-PET and PET/CT in breast cancer staging. Breast Care, vol 2007; page:372–377.
[5].Fahssi KE, Elmoufidi A, Abenaou A, Jai-Andaloussi S, Sekkaki A (2016) Novel approach to classification of Abnormalities in the mammogram image. International Journal of Biology and Biomedical Engineering.
[6]. Bozek J, Mustra M, Delac K, Grgic M (2009). A survey of image processing algorithms in digital mammography. Recent Advances in Multimedia Signal Processing and Communications, SCI, 231, 631–657.
[7]Yifan Chen, Ian James Craddock, and Panagiotis Kosmas, “Feasibility study of lesion classification via contrast-agent-aided uwb breast imaging,”IEEE Transactions on Biomedical Engineering, vol. 57, no. 5, pp.1003–1007, 2010.
[8].Rangaraj M Rangayyan, Nema M El-Faramawy, JE Leo Desautels, and Onsy Abdel Alim, “Measures of acutance and shape for classification of breast tumors,” IEEE Transactions on medical imaging, vol. 16, no.6, pp. 799–810, 1997.
[9]. C. Tobias Charistian Cahoon, Melanie A.Sutton, “Three-class mammogram classification based on descriptive cnn features,” 2000.
[10].Steven P. Poplack,MDTor D. Tosteson et. al, ScDElectromagnetic Breast Imaging:Results of a Pilot Study in Women with Abnormal Mammograms. Volume 243: Number 2—May 2007
[11].Rangaraj M Rangayyan, Nema M El-Faramawy, JE Leo Desautels, and Onsy Abdel Alim, “Measures of acutance and shape for classification of breast tumors,” IEEE Transactions on medical imaging, vol. 16, no.6, pp. 799–810, 1997.
[12] Sreedevi S, Sherly E (2015) A novel approach for removal of pectoral muscles in digital mammogram. Procedia Computer Science, 46: 724-1731.
[13] Pereira DC, Ramos R.P, Nascimento MZ (2014) “Segmentation and detection of breast cancer in mammograms combining wavelet analysis and genetic algorithm Computer Methods and Programs Biomedicine” vol 114 (1): 88-101.
[14] Anuradha.PV, Jose BR, Mathew J (2015) “Improved Segmentation of Suspicious Regions of Masses in Mammograms by Watershed Transform”. Procedia Computer Science 46:1483-1490.
[15] Kaur J, Kaur M (2016) “Automatic cancer detection in mammographic images.” International Journal of advanced Research in Computer Communications in Engineering (5) 7:473-476.
[16] Pam Stephan (2017) The basics on benign and cancerous breast lumps.
[17] Salazar-Licea LA, Pedraza-Ortega JC, Pastrana-Palma A, Marco A, Aceves-Fernandez (2017) Location of mammograms ROI’s and reduction of false-positive. Computer methods and Programs in Biomedicine 143:97-111.
[18].Li Y, Chen H, Yang Y, Yang N (2013) Pectoral muscle segmentation in mammograms based on homogenous texture and intensity deviation. Pattern Recognition. (46): 681 – 691
[19]. D. S. Shumakov, D. Tajik, A. S. Beaverstone, and N. K. Nikolova, “Study of practical limitations of real-time microwave imaging of tissue,”in Proc. IEEE Int. Symp. Antennas Propag., San Diego, CA, USA, Jul. 2017.
[20]. Timo Ojala, KimmoValkealahti, ErkkiOja, MattiPietikaKinen, “Texture discrimination with multidimensional distributions of signed gray-level differences”,Pattern Recognition, vol. 34, pp.727-739, 2001.
[21]. Goutam Barman, Babulal Seal “Survival Analysis of Breast Cancer Patients Using Additive Hazards Regression Models” ISROSET Volume-3 , Issue-6 Research Paper Page No : 7-10