An Efficient NB-IWD Based Network Traffic Classification over KDD Dataset
Amit Kumar1 , Daya Shankar Pandey2 , Varsha Namdeo3
Section:Research Paper, Product Type: Journal Paper
Volume-7 ,
Issue-4 , Page no. 706-710, Apr-2019
CrossRef-DOI: https://doi.org/10.26438/ijcse/v7i4.706710
Online published on Apr 30, 2019
Copyright © Amit Kumar, Daya Shankar Pandey, Varsha Namdeo . This is an open access article distributed under the Creative Commons Attribution License, which permits unrestricted use, distribution, and reproduction in any medium, provided the original work is properly cited.
View this paper at Google Scholar | DPI Digital Library
How to Cite this Paper
- IEEE Citation
- MLA Citation
- APA Citation
- BibTex Citation
- RIS Citation
IEEE Style Citation: Amit Kumar, Daya Shankar Pandey, Varsha Namdeo, “An Efficient NB-IWD Based Network Traffic Classification over KDD Dataset,” International Journal of Computer Sciences and Engineering, Vol.7, Issue.4, pp.706-710, 2019.
MLA Style Citation: Amit Kumar, Daya Shankar Pandey, Varsha Namdeo "An Efficient NB-IWD Based Network Traffic Classification over KDD Dataset." International Journal of Computer Sciences and Engineering 7.4 (2019): 706-710.
APA Style Citation: Amit Kumar, Daya Shankar Pandey, Varsha Namdeo, (2019). An Efficient NB-IWD Based Network Traffic Classification over KDD Dataset. International Journal of Computer Sciences and Engineering, 7(4), 706-710.
BibTex Style Citation:
@article{Kumar_2019,
author = {Amit Kumar, Daya Shankar Pandey, Varsha Namdeo},
title = {An Efficient NB-IWD Based Network Traffic Classification over KDD Dataset},
journal = {International Journal of Computer Sciences and Engineering},
issue_date = {4 2019},
volume = {7},
Issue = {4},
month = {4},
year = {2019},
issn = {2347-2693},
pages = {706-710},
url = {https://www.ijcseonline.org/full_paper_view.php?paper_id=4103},
doi = {https://doi.org/10.26438/ijcse/v7i4.706710}
publisher = {IJCSE, Indore, INDIA},
}
RIS Style Citation:
TY - JOUR
DO = {https://doi.org/10.26438/ijcse/v7i4.706710}
UR - https://www.ijcseonline.org/full_paper_view.php?paper_id=4103
TI - An Efficient NB-IWD Based Network Traffic Classification over KDD Dataset
T2 - International Journal of Computer Sciences and Engineering
AU - Amit Kumar, Daya Shankar Pandey, Varsha Namdeo
PY - 2019
DA - 2019/04/30
PB - IJCSE, Indore, INDIA
SP - 706-710
IS - 4
VL - 7
SN - 2347-2693
ER -
![]() |
![]() |
![]() |
246 | 208 downloads | 168 downloads |
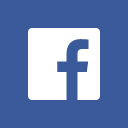
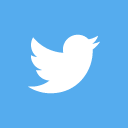
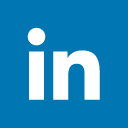
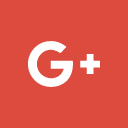
Abstract
The Network traffic arrangement is a procedure by which the large chips get away at different parameters for instance port and convention based which are utilized to identify the classes of the traffic. Thus these types of classification methods are very helpful in providing security at two levels- network as well as system. The main focus of this paper is sorting out the problem which comes while handling network traffic whereas some of the traffic classification methods are unable to find out the special requirements of individual datasets because there are massive measures of network traffic datasets and restricted quantities of resources are accessible to deliver classification examination. The paper uncovers that traffic arrangement should be refreshed normally to keep up the precision and ought to have the capacity to adjust the dynamic conduct of network stream.
Key-Words / Index Term
Network Traffic, Network Traffic Class, Network Features, Statistical features, Classification
References
[1]. R. Kwitt and U. Hofmann, “Unsupervised oddity recognition in system traffic by methods for strong PCA”, in Proc. Int. Conf. Processing in the Global Information Technology (ICCGI), 2007, pp. 3737.
[2]. Anshul Vishwakarma1* , Amit Khare2, “Vehicle Detection and Tracking for Traffic Surveillance Applications: A Review Paper”, Vol.-6, Issue-7, July 2018 E-ISSN: 2347-2693.
[3]. McGregor, M. Lobby, P. Lorier, and J. Brunskill. “Stream grouping utilizing AI methods”. LectureNotes in Computer Science, 2004, pp.205– 214.
[4]. L. Bernaille, R. Teixeira, I. Akodkenou, A. Soule, and K. “Salamatian. Traffic order on the flySIGCOMM Comput. Commun”. 2006.
[5]. Williams N., Zander S., Armitage G., “A Prelimenary Performanc Comparison of Five Machine Learning Algorithms for Practical IP Traffic Flow Comparison”, ACM SIGCOMM Computer Communication Review, Vol. 36, No. 5, 2006, pp. 5-16.
[6]. Andrew W. Moore and Denis Zuev. “Web traffic order utilizing bayesian examination procedures”. In Proceedings of ACM Sigmetrics,2005, pp.50-60.
[7]. ZHAO Jing-jing, HUANG Xiao-hong, SUN Qiong, MA Yan, “Real-time highlight choice in rush hour gridlock grouping”, ELSEVIER, 2008.
[8]. W. Yurcik and Y. Li, “Internet security representation contextual analysis: Instru-menting a system for netflow security perception devices”, in Proc. Yearly Computer Security Applications Conf. (ACSAC 05), Tucson, AZ, Dec. 59, 2005.
[9]. K. Thyagarajan 1* , N. Vaishnavi 2, “Performance Study on Malicious Program Prediction Using Classification Techniques”, Vol.-6, Issue-5, May 2018 E-ISSN: 2347-2693.
[10]. Y. Lim, H. Kim, J. Jeong, C. Kim, T. Kwon, and Y. Choi, “Internet Traffic Classification Demystified: On the Sources of the Discriminative Power”, Proc. ACM CoNEXT, 2010, p. 9.