Eyeopen for Predicting of Flood Flow by Statistical and Machine Learning Model
D. Suganya1 , V. Savithri2
Section:Review Paper, Product Type: Journal Paper
Volume-7 ,
Issue-4 , Page no. 564-568, Apr-2019
CrossRef-DOI: https://doi.org/10.26438/ijcse/v7i4.564568
Online published on Apr 30, 2019
Copyright © D. Suganya, V. Savithri . This is an open access article distributed under the Creative Commons Attribution License, which permits unrestricted use, distribution, and reproduction in any medium, provided the original work is properly cited.
View this paper at Google Scholar | DPI Digital Library
How to Cite this Paper
- IEEE Citation
- MLA Citation
- APA Citation
- BibTex Citation
- RIS Citation
IEEE Style Citation: D. Suganya, V. Savithri, “Eyeopen for Predicting of Flood Flow by Statistical and Machine Learning Model,” International Journal of Computer Sciences and Engineering, Vol.7, Issue.4, pp.564-568, 2019.
MLA Style Citation: D. Suganya, V. Savithri "Eyeopen for Predicting of Flood Flow by Statistical and Machine Learning Model." International Journal of Computer Sciences and Engineering 7.4 (2019): 564-568.
APA Style Citation: D. Suganya, V. Savithri, (2019). Eyeopen for Predicting of Flood Flow by Statistical and Machine Learning Model. International Journal of Computer Sciences and Engineering, 7(4), 564-568.
BibTex Style Citation:
@article{Suganya_2019,
author = {D. Suganya, V. Savithri},
title = {Eyeopen for Predicting of Flood Flow by Statistical and Machine Learning Model},
journal = {International Journal of Computer Sciences and Engineering},
issue_date = {4 2019},
volume = {7},
Issue = {4},
month = {4},
year = {2019},
issn = {2347-2693},
pages = {564-568},
url = {https://www.ijcseonline.org/full_paper_view.php?paper_id=4077},
doi = {https://doi.org/10.26438/ijcse/v7i4.564568}
publisher = {IJCSE, Indore, INDIA},
}
RIS Style Citation:
TY - JOUR
DO = {https://doi.org/10.26438/ijcse/v7i4.564568}
UR - https://www.ijcseonline.org/full_paper_view.php?paper_id=4077
TI - Eyeopen for Predicting of Flood Flow by Statistical and Machine Learning Model
T2 - International Journal of Computer Sciences and Engineering
AU - D. Suganya, V. Savithri
PY - 2019
DA - 2019/04/30
PB - IJCSE, Indore, INDIA
SP - 564-568
IS - 4
VL - 7
SN - 2347-2693
ER -
![]() |
![]() |
![]() |
493 | 269 downloads | 161 downloads |
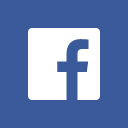
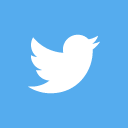
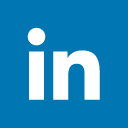
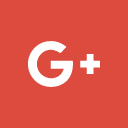
Abstract
Floods are rare and dangerous disaster in minimum duration, which have the most destructive impact within urban and rural areas. The research on the advancement of flood prediction models contributed to risk reduction, to prevent the loss of human life, and reduction the property damage in floods. This paper structure the machine learning models, two separate models based on including and excluding the river flow were developed for each variable to quantify the importance of the river flow on the accuracy of the flood forecasting models. In this paper, aims to discovering more accurate and efficient prediction models. In recent research, two main approaches are developed in hydrological forecasting. The first approach is based on mathematical modeling. It models the physical dynamics between the principal components of the hydrological system. The second approach is based on modeling the statistical relationship between the hydrologic input and output, without explicitly considering the relationships that exist among the involved physical processes. The water level flow is deducted in this research, river flow proves the most and least improvement on the efficiency of the models applied for flood forecasting. As a result, this paper describes the most promising prediction methods for both long-term and short-term floods. This paper can be used as a predicting the flood by choosing the proper Machine Learning (ML) algorithm such as Support Vector Machine(SVM) and Artificial Neural Network(ANN) algorithm for showing higher accuracy.
Key-Words / Index Term
flood prediction, hydrological model, machine learning, flood prediction, artificial intelligence, time series prediction
References
[1] H. Shahraiyni, M. Ghafouri, S. Shouraki, B. Saghafian, and M. Nasseri, “Comparison between active learning method and support vector machine for runoff modeling,” J. Hydrol. Hydromechanics, vol. 60, no. 1, pp. 16–32, 2012.
[2] S. H. Elsafi, “Artificial Neural Networks (ANNs) for flood forecasting at Dongola Station in the River Nile, Sudan,” Alexandria Eng. J., vol. 53, no. 3, pp. 655–662, 2014.
[3] J. Noymanee, N. O. Nikitin, and A. V. Kalyuzhnaya, “Urban Pluvial Flood Forecasting using Open Data with Machine Learning Techniques in Pattani Basin,” Procedia Comput. Sci., vol. 119, no. 2017, pp. 288–297, 2017.
[4] O. I. Abiodun, A. Jantan, A. E. Omolara, K. V. Dada, N. A. E. Mohamed, and H. Arshad, “State-of-the-art in artificial neural network applications: A survey,” Heliyon, vol. 4, no. 11, p. e00938, 2018.
[5] R. UshaRani, T. K. R. Krishna Rao, and R. Kiran Kumar Reddy, “An Efficient Machine Learning Regression Model for Rainfall Prediction,” Int. J. Comput. Appl., vol. 115, no. 23, pp. 24–30, 2015.
[6] J. Abbot and J. Marohasy, “Forecasting of Medium-term Rainfall Using Artificial Neural Networks: Case Studies from Eastern Australia,” Eng. Math. Top. Rainfall, 2018.
[7] F. Liu, F. Xu, and S. Yang, “A Flood Forecasting Model Based on Deep Learning Algorithm via Integrating Stacked Autoencoders with BP Neural Network,” Proc. - 2017 IEEE 3rd Int. Conf. Multimed. Big Data, BigMM 2017, pp. 58–61, 2017.
[8] M. Hitokoto and M. Sakuraba, “Applicability of the Deep Learning Flood Forecast Model Against the Inexperienced Magnitude of Flood,” vol. 3, pp. 901–893, 2018.
[9] P. Misra and S. Shukla, “Implementation of Neuro-Fuzzy and Statistical Technique for Flood Forecasting in Cauvery Basin , India,” no. 6, 2018.
[10] S. Vandana, D. D. Raj, B. Rushika, and V. M. Venkatesh, “Open Access,” no. 11, pp. 444–447, 2018.