Missing Value Imputation-A Review
Dipalika Das1 , Maya Nayak2 , Subhendu Kumar Pani3
Section:Review Paper, Product Type: Journal Paper
Volume-7 ,
Issue-4 , Page no. 548-558, Apr-2019
CrossRef-DOI: https://doi.org/10.26438/ijcse/v7i4.548558
Online published on Apr 30, 2019
Copyright © Dipalika Das, Maya Nayak, Subhendu Kumar Pani . This is an open access article distributed under the Creative Commons Attribution License, which permits unrestricted use, distribution, and reproduction in any medium, provided the original work is properly cited.
View this paper at Google Scholar | DPI Digital Library
How to Cite this Paper
- IEEE Citation
- MLA Citation
- APA Citation
- BibTex Citation
- RIS Citation
IEEE Style Citation: Dipalika Das, Maya Nayak, Subhendu Kumar Pani, “Missing Value Imputation-A Review,” International Journal of Computer Sciences and Engineering, Vol.7, Issue.4, pp.548-558, 2019.
MLA Style Citation: Dipalika Das, Maya Nayak, Subhendu Kumar Pani "Missing Value Imputation-A Review." International Journal of Computer Sciences and Engineering 7.4 (2019): 548-558.
APA Style Citation: Dipalika Das, Maya Nayak, Subhendu Kumar Pani, (2019). Missing Value Imputation-A Review. International Journal of Computer Sciences and Engineering, 7(4), 548-558.
BibTex Style Citation:
@article{Das_2019,
author = {Dipalika Das, Maya Nayak, Subhendu Kumar Pani},
title = {Missing Value Imputation-A Review},
journal = {International Journal of Computer Sciences and Engineering},
issue_date = {4 2019},
volume = {7},
Issue = {4},
month = {4},
year = {2019},
issn = {2347-2693},
pages = {548-558},
url = {https://www.ijcseonline.org/full_paper_view.php?paper_id=4075},
doi = {https://doi.org/10.26438/ijcse/v7i4.548558}
publisher = {IJCSE, Indore, INDIA},
}
RIS Style Citation:
TY - JOUR
DO = {https://doi.org/10.26438/ijcse/v7i4.548558}
UR - https://www.ijcseonline.org/full_paper_view.php?paper_id=4075
TI - Missing Value Imputation-A Review
T2 - International Journal of Computer Sciences and Engineering
AU - Dipalika Das, Maya Nayak, Subhendu Kumar Pani
PY - 2019
DA - 2019/04/30
PB - IJCSE, Indore, INDIA
SP - 548-558
IS - 4
VL - 7
SN - 2347-2693
ER -
![]() |
![]() |
![]() |
797 | 285 downloads | 142 downloads |
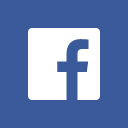
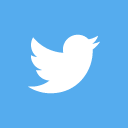
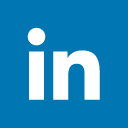
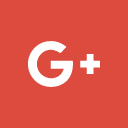
Abstract
The problems of missing values in the field of data mining have become emerging areas of research in recent years. It has been a great challenge in research for quite a long time. The missing values may occur due to several reasons. The missing values in the data set can affect accuracy and performance of result when any algorithm is implemented on it. Presence of missing values leads to less efficiency and difficulty in extracting meaningful information. As we go through the literature we can find there are various imputation techniques basing on type of missing value. Since the amount of data is increasing day by day, there is a need for an appropriate technique to handle the missing values in the data set. In this paper a brief year wise study of existing methods are being done so that it would be a great help while formulating and implementing a new one towards solving the problem of missing values.
Key-Words / Index Term
Data Mining, Data Set, Missing Values, Algorithm, Information, Imputation
References
[1] Qian Ma, Yu Gu, Wang-Chien Lee and Ge Yu, ”Order-Sensitive Imputation for Clustered Missing Values”, IEEE Transactions on Knowledge and Data Engineering, 1041-4347 ©2018.
[2] Teresa Pamuła, “Impact of Data Loss for Prediction of Traffic Flow on an Urban Road Using Neural Networks”, IEEE Transactions On Intelligent Transportation Systems 1524-9050 © 2018.
[3] Siamak Zamani Dadaneh , Edward R. Dougherty and Xiaoning Qian , “Optimal Bayesian Classification With Missing Values”, IEEE Transactions On Signal Processing, Vol. 66, No. 16, August 15, 2018.
[4] Aiguo Wang, Ye Chen, Ning An, Jing Yang, Lian Li, and Lili Jiang, “Microarray Missing Value Imputation: A Regularized Local Learning Method”, IEEE, 1545-5963 ©2018.
[5] Wujun Si, Qingyu Yang , Leslie Monplaisir and Yong Chen, “Reliability Analysis of Repairable Systems With Incomplete Failure Time Data”, IEEE , 0018-9529 © 2018.
[6] Nur Afiqah Zakaria, Norazian Mohamed Noor,” Imputation Methods For Filling Missing Data In Urban Air Pollution Data For Malaysia”, Urbanism. Arhitectură. Construcţii, Vol. 9 , No. 2 , 2018.
[7] Xiaolong Xu, Weizhi Chong, Shancang Li, Abdullahi Arabo, “Missing Data Imputation Based On The Evidence Chain”, IEEE Access, Vol. 6, 2169-3536, 2018.
[8] Zeng Yu, Tianrui Li, Shi-Jinn Horng, Yi Pan, Hongjun Wang and Yunge Jing, “An Iterative Locally Auto-Weighted Least Squares Method for Microarray Missing Value Estimation”, IEEE Transactions On Nanobioscience, Vol. 16, No. 1, January 2017.
[9] Ivan Markovsky,” A Missing Data Approach to Data-Driven Filtering and Control”, IEEE Transactions On Automatic Control, Vol. 62, No. 4, April 2017.
[10] Weiwei Shi, Yongxin Zhu, Philip S. Yu, Jiawei Zhang, Tian Huang, Chang Wang, and Yufeng Chen, “Effective Prediction of Missing Data on Apache Spark over Multivariable Time Series”, IEEE Transactions on Big Data ,DOI 10.1109/TBDATA.2017.2719703.
[11] R. Misir and R.K. Samanta,”A Study on performance of UCI Hungarian dataset using missing value management techniques”, IJCSE, Volume-5, Issue-3, 2017.
[12] Malay Mitra and R. K. Samanta,”
A Study on Missing Data Management”, IJCSE, Volume-5, Issue-2, E-ISSN: 2347-2693, 2017.
[13] Yelipe UshaRani, Dr.P.Sammulal, “An Innovative Imputation and Classification Approach for Accurate Disease Prediction”, International Journal of Computer Science and Information Security (IJCSIS), Vol. 14 S1, February 2016.
[14] Darryl ND, Rahman MM, “Missing Value Imputation Using Stratified Supervised Learning for Cardiovascular Data”, Global J Technol Optim 7:6 DOI: 10.4172/2229-8711. S1:113,2016.
[15] Tejal Patil, “Systematic Mapping Study of Missing ValuesTechniques using Naive Bayes”, IRJET, e-ISSN: 2395 -0056, Volume: 03, Issue: 03 , Mar-2016.
[16] Y.Usha Rani1, P. Sammulal, “A Novel Approach for Imputation of Missing Attribute Values for Efficient Mining of Medical Datasets – Class Based Cluster Approach”, Rev. Téc. Ing. Univ. Zulia. Vol. 39, No 2, 184 - 195, 2016.
[17] R. Naveen Kumar, M. Anand Kumar, “Enhanced Fuzzy K-NN Approach for Handling Missing Values in Medical Data Mining”, Indian Journal of Science and Technology, Vol 9(S1), DOI: 10.17485/ijst/2016/v9iS1/94094 , December 2016.
[18] Jocelyn T. Chi, Eric C. Chi, and Richard G. Baraniuk, “k-POD A Method for k-Means Clustering of Missing Data”, arXiv:1411.7013v3 [stat.CO] 27 Jan 2016.
[19] Swati Jain & Mrs. Kalpana Jain, “Estimation of Missing Attribute Value in Time Series Database in Data Mining”, Global Journals Inc. (USA), Volume 16, Issue 5, Version 1.0, Year 2016.
[20] P.Saravanan,P.Sailakshmi, “Missing Value Imputation Using Fuzzy Possibilistic C Means Optimized With Support Vector Regression And Genetic Algorithm”, JATIT & LLS, Vol.72, No.1, 2015.
[21] Elsiddig Elsadig Mohamed Koko, Amin Ibrahim Adam Mohamed, “Missing Data Treatment Method On Cluster Analysis”, International Journal of Advanced Statistics and Probability, Vol.3,No.2 ,191-209, 2015.
[22] Huseyin Ozkan, Ozgun Soner Pelvan, and Suleyman S. Kozat, “Data Imputation Through the Identification of Local Anomalies”, IEEE Transactions On Neural Networks And Learning Systems, Vol. 26, NO. 10, October 2015.
[23] Edgar Acuna ,Caroline Rodriguez, “The treatment of missing values and its effect in the classifier accuracy”, Research Gate, DOI: 10.1007/978-3-642-17103-1_60, 2015 .
[24] Artur Matyja, “Comparison of Algorithms for Clustering Incomplete Data, Foundations Of Computing And Decision Sciences”, Vol.39, No.2, DOI: 10.2478/fcds-2014-0007, ISSN 0867-6356, 2014.
[25] Minakshi, Dr. Rajan Vohra, Gimpy, “Missing Value Imputation in Multi Attribute Data Set”, IJCSIT, Vol. 5 (4) , 5315-5321, 2014,.
[26] Xiaoping Zhu, “Comparison of Four Methods for Handling Missing Data in Longitudinal Data Analysis Through a Simulation Study”, Open Journal of Statistics, 4, 933-944, 2014.
[27] Jiri Kaiser, “Dealing with Missing Values in Data, Journal Of Systems Integration”, 2014/1.
[28] Tapas Ranjan Baitharu and Subhendu Kumar Pani, “Effect of Missing Values on Data Classification, JETEAS”, 4(2): 311-316, (ISSN: 2141-7016), 2013.
[29] Luciano C. Blomberg, Duncan Dubugras A. Ruiz, “Evaluating the Influence of Missing Data on Classification Algorithms in Data Mining Applications”,SBSI,2013.
[30] Jing Tian, Bing Yu, Dan Yu, and Shilong Ma, “Clustering-Based Multiple Imputation via Gray Relational Analysis for Missing Data and Its Application to Aerospace Field”, The ScientificWorld Journal, Article ID 720392, 10 pages, 2013.
[31] Sujatha.R, “Enhancing Iterative Non-Parametric Algorithm for Calculating Missing Values of Heterogeneous Datasets by Clustering”, IJSR Publications, Volume 3, Issue 3, March 2013.
[32] Aasha.M, “Imputation in Mixed Attribute Datasets using Higher Order Kernel Functions”, IJIET, Vol. 2 Issue 3, ISSN: 2319-1058, June 2013.
[33] Santosh Dane, Dr. R. C. Thool, “Imputation Method for Missing Value Estimation of Mixed-Attribute Data Sets”, IJARCSSE, Volume 3, Issue 5, ISSN: 2277 128X, May 2013.
[34] Ji Liu, Przemyslaw Musialski, Peter Wonka, and Jieping Ye, “Tensor Completion for Estimating Missing Values in Visual Data”, IEEE Transactions On Pattern Analysis And Machine Intelligence, Vol. 35, No. 1, January 2013.
[35] Bhavisha Suthar, Hemant Patel, Ankur Goswami,”A Survey: Classification of Imputation Methods in Data Mining”,IJETAE, ISSN 2250-2459, Volume 2, Issue 1, January 2012.
[36] R.Devi Priya, S.Kuppuswami, “A Genetic Algorithm Based Approach for Imputing Missing Discrete Attribute Values in Databases”, WSEAS Transactions On Information Science And Applications, E-ISSN: 2224-3402, Volume 9, Issue 6, June 2012.
[37] Noel Lopes, Bernardete Ribeiro, “Handling Missing Values Via A Neural Selective Input Model” Neural Network World 4/12, 357-370, ICS AS CR 2012.
[38] Julian Luengo, Jose A. Saez, Francisco Herrera,”Missing data imputation for fuzzy rule-based classification systems”, 16:863–881 DOI 10.1007/s00500-011-0774-4, 2012.
[39] Satish Gajawada, Durga Toshniwal, “Missing Value Imputation Method Based on Clustering and Nearest Neighbours”, International Journal of Future Computer and Communication, Vol. 1, No. 2, August 2012.
[40] K. Raja , G. Tholkappia Arasu ,Chitra. S. Nair, “Imputation Framework for Missing Values, International Journal of Computer Trends and Technology”, volume3,Issue2,2012.
[41] Ganga.A.R, B.Lakshmipathi, “Higher Order Kernel Function Algorithm for Imputing Missing Values”,IJARCS, Volume 3, No. 3, ISSN No. 0976-5697, May-June 2012,.
[42] Ibrahim Berkan Aydilek and Ahmet Arslan, “A Novel Hybrid Approach To Estimating Missing Values In Databases Using K-Nearest Neighbors And Neural Networks”, International Journal of Innovative Computing, Information and Control, ISSN 1349-4198,Volume 8, Number 7(A), pp. 4705-4717, July 2012,.
[43] R.S. Somasundaram, R. Nedunchezhian, “Missing Value Imputation using Refined Mean Substitution”, IJCSI, Vol. 9, Issue 4, No 3, July 2012.