Network Intrusion Detection System using Threat Intelligence and Deep Learning Approach
Kushal Jani1 , Punit Lalwani2 , Deepak Upadhyay3 , M. B. Potdar4
Section:Research Paper, Product Type: Journal Paper
Volume-7 ,
Issue-4 , Page no. 526-531, Apr-2019
CrossRef-DOI: https://doi.org/10.26438/ijcse/v7i4.526531
Online published on Apr 30, 2019
Copyright © Kushal Jani, Punit Lalwani, Deepak Upadhyay, M. B. Potdar . This is an open access article distributed under the Creative Commons Attribution License, which permits unrestricted use, distribution, and reproduction in any medium, provided the original work is properly cited.
View this paper at Google Scholar | DPI Digital Library
How to Cite this Paper
- IEEE Citation
- MLA Citation
- APA Citation
- BibTex Citation
- RIS Citation
IEEE Style Citation: Kushal Jani, Punit Lalwani, Deepak Upadhyay, M. B. Potdar, “Network Intrusion Detection System using Threat Intelligence and Deep Learning Approach,” International Journal of Computer Sciences and Engineering, Vol.7, Issue.4, pp.526-531, 2019.
MLA Style Citation: Kushal Jani, Punit Lalwani, Deepak Upadhyay, M. B. Potdar "Network Intrusion Detection System using Threat Intelligence and Deep Learning Approach." International Journal of Computer Sciences and Engineering 7.4 (2019): 526-531.
APA Style Citation: Kushal Jani, Punit Lalwani, Deepak Upadhyay, M. B. Potdar, (2019). Network Intrusion Detection System using Threat Intelligence and Deep Learning Approach. International Journal of Computer Sciences and Engineering, 7(4), 526-531.
BibTex Style Citation:
@article{Jani_2019,
author = {Kushal Jani, Punit Lalwani, Deepak Upadhyay, M. B. Potdar},
title = {Network Intrusion Detection System using Threat Intelligence and Deep Learning Approach},
journal = {International Journal of Computer Sciences and Engineering},
issue_date = {4 2019},
volume = {7},
Issue = {4},
month = {4},
year = {2019},
issn = {2347-2693},
pages = {526-531},
url = {https://www.ijcseonline.org/full_paper_view.php?paper_id=4070},
doi = {https://doi.org/10.26438/ijcse/v7i4.526531}
publisher = {IJCSE, Indore, INDIA},
}
RIS Style Citation:
TY - JOUR
DO = {https://doi.org/10.26438/ijcse/v7i4.526531}
UR - https://www.ijcseonline.org/full_paper_view.php?paper_id=4070
TI - Network Intrusion Detection System using Threat Intelligence and Deep Learning Approach
T2 - International Journal of Computer Sciences and Engineering
AU - Kushal Jani, Punit Lalwani, Deepak Upadhyay, M. B. Potdar
PY - 2019
DA - 2019/04/30
PB - IJCSE, Indore, INDIA
SP - 526-531
IS - 4
VL - 7
SN - 2347-2693
ER -
![]() |
![]() |
![]() |
672 | 361 downloads | 211 downloads |
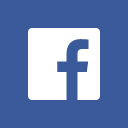
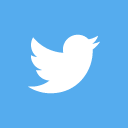
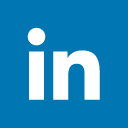
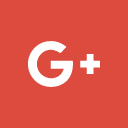
Abstract
Network Intrusion Detection System (NIDS) is one of the best solutions against network attacks. Attackers also dynamically change tools and technologies. Powerful network security analytics is not a function of making use of simply one approach. To detect emerging threats, a network intrusion detection system should be able to use a mixture of techniques. In this research we start off evolved via collecting the proper information for complete visibility and the usage of analytical strategies along with behavioral modeling and deep learning. All that is supplemented by means of global threat intelligence that is aware about the malicious campaigns and maps the suspicious conduct to a recognized threat for extended fidelity of detection. In this research we prepare multichannel deep learning approach and incremental learning approach to enhance detection rate.
Key-Words / Index Term
Network Intrusion Detection System, Network Security, Deep Learning, Threat Intelligence, Performance Evolution.
References
[1] Almseidin, Mohammad, et al. "Evaluation of machine learning algorithms for intrusion detection system." Intelligent Systems and Informatics (SISY), 2017 IEEE 15th International Symposium on. IEEE, 2017.
[2] Choudhury, Sumouli, and AnirbanBhowal. "Comparative analysis of machine learning algorithms along with classifiers for network intrusion detection." Smart technologies and management for computing, communication, controls, energy and materials (ICSTM), 2015 International conference on. IEEE, 2015.
[3] Hamid, Yasir, M. Sugumaran, and LudovicJournaux. "Machine learning techniques for intrusion detection: a comparative analysis." Proceedings of the International Conference on Informatics and Analytics. ACM, 2016.
[4] Haq, Nutan Farah, et al. "Application of machine learning approaches in intrusion detection system: a survey." IJARAI-International Journal of Advanced Research in Artificial Intelligence 4.3 (2015): 9-18.
[5] Dhanabal, L., and S. P. Shantharajah. "A study on NSL-KDD dataset for intrusion detection system based on classification algorithms." International Journal of Advanced Research in Computer and Communication Engineering 4.6 (2015): 446-452.
[6] Buczak, Anna L., and ErhanGuven. "A survey of data mining and machine learning methods for cyber security intrusion detection." IEEE Communications Surveys & Tutorials 18.2 (2016): 1153-1176.
[7] Belavagi, Manjula C., and BalachandraMuniyal. "Performance evaluation of supervised machine learning algorithms for intrusion detection." Procedia Computer Science 89 (2016): 117-123.
[8] Shanmugavadivu, R., and N. Nagarajan. "Network intrusion detection system using fuzzy logic." Indian Journal of Computer Science and Engineering (IJCSE) 2.1 (2011): 101-111.
[9] Shone, Nathan, et al. "A deep learning approach to network intrusion detection." IEEE Transactions on Emerging Topics in Computational Intelligence 2.1 (2018): 41-50.
[10] Park, Kinam, Youngrok Song, and Yun-Gyung Cheong. "Classification of Attack Types for Intrusion Detection Systems Using a Machine Learning Algorithm." 2018 IEEE Fourth International Conference on Big Data Computing Service and Applications (BigDataService). IEEE, 2018.
[11] Papernot, Nicolas, et al. "Towards the science of security and privacy in machine learning." arXiv preprint arXiv:1611.03814(2016).
[12] Lee, Chie-Hong, et al. "Machine learning based network intrusion detection." Computational Intelligence and Applications (ICCIA), 2017 2nd IEEE International Conference on. IEEE, 2017.
[13] Jiang, Feng, et al. "Deep Learning based Multi-channel intelligent attack detection for Data Security." IEEE Transactions on Sustainable Computing (2018).
[14] Haque, MdEnamul, and Talal M. Alkharobi. "Adaptive hybrid model for network intrusion detection and comparison among machine learning algorithms." International Journal of Machine Learning and Computing 5.1 (2015): 17.
[15] Ahmed, Mohiuddin, AbdunNaser Mahmood, and Jiankun Hu. "A survey of network anomaly detection techniques." Journal of Network and Computer Applications 60 (2016): 19-31.
[16] Liu, Qiang, et al. "A survey on security threats and defensive techniques of machine learning: a data driven view." IEEE access 6 (2018): 12103-12117.
[17] https://en.wikipedia.org/wiki/Machine_learning
[18] https://en.wikipedia.org/wiki/Random_forest
[19] https://machinelearningmastery.com/a-tour-of-machine-learning-algorithms/
[20] Bromiley, Matt. "Threat intelligence: What it is, and how to use it effectively." SANS Institute InfoSec Reading Room (2016).
[21] https://en.wikipedia.org/wiki/Intrusion_detection_sy-stem
[22] Gaddam, Ravi Teja, and M. Nandhini. "Analysis of Various Intrusion Detection Systems with a Model for Improving Snort Performance." Indian Journal of Science and Technology10.20 (2017).
[23] Kumar, Sailesh. "Survey of current network intrusion detection techniques." Washington Univ. in St. Louis (2007).
[24] Kwon, Donghwoon, et al. "A survey of deep learning-based network anomaly detection." Cluster Computing (2017): 1-13.
[25] Jasper, Scott E. "US cyber threat intelligence sharing frameworks." International Journal of Intelligence and Counter Intelligence 30.1 (2017): 53-65.
[26] Suman Sharma, Yogesh Verma, Amit Nadda, "Information Security: Cyber Security Challenges", International Journal of Scientific Research in Computer Science and Engineering, Vol.7, Issue.1, pp.10-15, 2019
[27] Shailja Sharma, Sheeba Khan, "Analysis of Cloud Security, Performance, Scalability and Availability (SPSA)", International Journal of Scientific Research in Network Security and Communication, Vol.7, Issue.1, pp.13-15, 2019