“Breast Cancer Diagnosis and Classification Using Support vector machines With Diverse Datasets”
Vikas S1 , Thimmaraju S N2
Section:Survey Paper, Product Type: Journal Paper
Volume-7 ,
Issue-4 , Page no. 442-446, Apr-2019
CrossRef-DOI: https://doi.org/10.26438/ijcse/v7i4.442446
Online published on Apr 30, 2019
Copyright © Vikas S, Thimmaraju S N . This is an open access article distributed under the Creative Commons Attribution License, which permits unrestricted use, distribution, and reproduction in any medium, provided the original work is properly cited.
View this paper at Google Scholar | DPI Digital Library
How to Cite this Paper
- IEEE Citation
- MLA Citation
- APA Citation
- BibTex Citation
- RIS Citation
IEEE Style Citation: Vikas S, Thimmaraju S N, ““Breast Cancer Diagnosis and Classification Using Support vector machines With Diverse Datasets”,” International Journal of Computer Sciences and Engineering, Vol.7, Issue.4, pp.442-446, 2019.
MLA Style Citation: Vikas S, Thimmaraju S N "“Breast Cancer Diagnosis and Classification Using Support vector machines With Diverse Datasets”." International Journal of Computer Sciences and Engineering 7.4 (2019): 442-446.
APA Style Citation: Vikas S, Thimmaraju S N, (2019). “Breast Cancer Diagnosis and Classification Using Support vector machines With Diverse Datasets”. International Journal of Computer Sciences and Engineering, 7(4), 442-446.
BibTex Style Citation:
@article{S_2019,
author = {Vikas S, Thimmaraju S N},
title = {“Breast Cancer Diagnosis and Classification Using Support vector machines With Diverse Datasets”},
journal = {International Journal of Computer Sciences and Engineering},
issue_date = {4 2019},
volume = {7},
Issue = {4},
month = {4},
year = {2019},
issn = {2347-2693},
pages = {442-446},
url = {https://www.ijcseonline.org/full_paper_view.php?paper_id=4054},
doi = {https://doi.org/10.26438/ijcse/v7i4.442446}
publisher = {IJCSE, Indore, INDIA},
}
RIS Style Citation:
TY - JOUR
DO = {https://doi.org/10.26438/ijcse/v7i4.442446}
UR - https://www.ijcseonline.org/full_paper_view.php?paper_id=4054
TI - “Breast Cancer Diagnosis and Classification Using Support vector machines With Diverse Datasets”
T2 - International Journal of Computer Sciences and Engineering
AU - Vikas S, Thimmaraju S N
PY - 2019
DA - 2019/04/30
PB - IJCSE, Indore, INDIA
SP - 442-446
IS - 4
VL - 7
SN - 2347-2693
ER -
![]() |
![]() |
![]() |
480 | 297 downloads | 192 downloads |
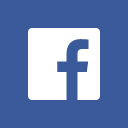
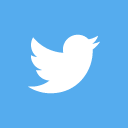
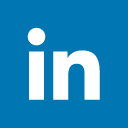
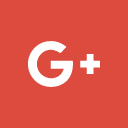
Abstract
Breast cancer is the most prevalent cancer among women around the world. However, increased survival is due to the dramatic advances in the screening methods, early diagnosis, and breakthroughs in treatments. Different strategies of breast cancer classification and staging have evolved over the years. Intrinsic (molecular) sub composing is fundamental in clinical preliminaries and well comprehension of the sickness of the disease. To analyze machine learning systems have been utilized to define a set trained with the “bagging” method. Support vector machines (SVM) have been appeared to outflank numerous related methods. However, there have been very few studies focused on examining the classification performances of different classification. The trial comes about demonstrate that SVM classifier can be the better decision for classification, where accuracy of the algorithm is improved by tuning the parameters of the dataset.
Key-Words / Index Term
Machine Learning, Support Vector Machine(SVM)
References
[1]. U.S. Cancer Statistics Working Group. United
[2]. States Cancer Statistics: 1999–2008 Incidence and Mortality Web-based
[3]. Report. Atlanta (GA): Department of Health and Human Services, Centers for Disease Control and Prevention, and National Cancer Institute; 2012.
[4]. Siegel RL, Miller KD, Jemal A. Cancer Statistics , 2016.
[5]. Noble WS. What is a support vector machine? Nat Biotechnol.
[6]. Rish I. An empirical study of the naive Bayes
[7]. classifier. IJCAI Work Empir methods ArtifIntell. 2001.
[8]. Quinlan JR. C4.5: Programs for Machine Learning.
[9]. Larose DT. Discovering Knowledge in Data.
[10]. Hoboken, NJ, USA: John Wiley & Sons, Inc.; 2004.
[11]. X. Wu, V. Kumar, J. R. Quinlan, J. Ghosh, Q. Yang,H. Motoda, G. J. Mclachlan, A. Ng, B. Liu, P. S. Yu, Z. Z. Michael, S.
[12]. David, and J. H. Dan, Top 10 algorithms in data mining. 2008.
[13]. Datafloq - Top 10 Data Mining Algorithms,
[14]. Demystified.
[15]. 44. Accessed December 29, 2015.V. Chaurasia and S. Pal, “Data Mining Techniques : To Predict and Resolve Breast Cancer Survivability,” vol. 3,2014.
[16]. Djebbari, A., Liu, Z., Phan, S., AND Famili, F. International journal of computational biology anddrug design (ijcbdd). 21st Annual Conference on Neural Information Processing Systems (2008).
[17]. S. Aruna and L. V Nandakishore, “KNOWLEDGE BASED ANALYSIS OF VARIOUS STATISTICAL TOOLS IN DETECTING BREAST,”
[18]. A. C. Y, “An Empirical Comparison of Data Mining Classification Methods,” vol. 3, no. 2, 2011.
[19]. A. Pradesh, “Analysis of Feature Selection with Classification : Breast Cancer Datasets,” Indian J. Comput. Sci. Eng., vol. 2, no.5, 2011.
[20]. Thorsten J. Transductive Inference for Text
[21]. Classification Using Support Vector Machines.
[22]. Icml. 1999.
[23]. L. Ya-qin, W. Cheng, and Z. Lu, “Decision tree based predictive models for breast cancer
[24]. survivability on imbalanced data,” 2009.
[25]. D. Delen, G. Walker, and A. Kadam, “Predicting breast cancer survivability: a comparison of three data mining methods,” Artif. Intell. Med., vol. 34, pp. 113–127, 2005. W. Version, “Machine Learningwith WEKA,” 2004.
[26]. “UCI Machine Learning Repository: Breast Cancer Wisconsin (Original) Data Set.” [Online].
[27]. “SUGI 31 Statistics and Data Analysis Receiver Operating Characteristic ( ROC ) Curves MithatGönen , Memorial Sloan-Kettering Cancer Center SUGI 31 Statistics and Data Analysis FN + FP,”, 2001.
[28]. Olutobi Owoputi, Brendan O’Connor, Chris Dyer, Kevin Gimpel, Nathan Schneider, and Noah A. Smith. 2013. Improved part-of-speech tagging for online conversational text with word clusters. In Proceedings of NAACL-2013, pages 380–390.
[29]. Fabian Pedregosa, Gael Varoquaux, Alexandre Gramfort, Vincent Michel, Bertrand Thirion, Olivier Grisel, Mathieu Blondel, Peter Prettenhofer, Ron Weiss, Vincent Dubourg, Jake Vanderplas, Alexandre Passos, David Cournapeau, Matthieu Brucher, Matthieu Perrot, and Edouard Duchesnay. 2011. Scikit-learn: Machine learning in Python. Journal of Machine Learning Research, 12:2825–2830.
[30]. M. Zaharia, M. Chowdhury, T. Das, A. Dave, J. Ma,M. McCauley, M. Franklin, S. Shenker, and I. Stoica.Resilient Distributed Datasets: A fault-tolerantabstraction for in-memory cluster computing. In NSDI, 2012.
[31]. M. Bostock, V. Ogievetsky, and J. Heer. D3: Data-Driven Documents. In InfoVis, 2011.
[32]. Pandey, Neha, B. K. Singh, and Ankur Singh Bist. "A novel feature learning for image classification using wrapper approach in GA." Signal Processing and Integrated Networks (SPIN), 2015 2nd International Conference on. IEEE, 2015.
[33]. M. Li, T. Zhang, Y. Chen, and A. J. Smola. Efficient mini-batch training for stochastic optimization. In Proceedings of the 20th ACM SIGKDD International Conference on Knowledge Discovery and Data Mining, KDD ’14, pages 661–670, New York, NY, USA, 2014.ACM. ww.cs.cmu.edu/˜muli/file/minibatch sgd.pdf.
[34]. C. J. Maddison, A. Huang, I. Sutskever, and D. Silver. Move evaluation in Go using deep convolutional neural networks. arXiv preprint arXiv:1412.6564, 2014. arxiv.org/abs/1412.6564.
[35]. F. McSherry, M. Isard, and D. G. Murray. Scalability! But at what COST? In Proceedings of the 15th USENIX Conference on Hot Topics in Operating Systems, HOTOS’15, Berkeley, CA, USA, 2015. USENIX Association. www.usenix.org/system/files/conference/hotos15/hotos15-paper-mcsherry.pdf.
[36]. P. Moritz, R. Nishihara, I. Stoica, and M. I. Jordan. SparkNet: Training deep networks in Spark. In International Conference on Learning Represntations, 2016. arxiv.org/abs/1511.06051.
[37]. Kriti Jain1, Megha Saxena2andShweta Sharma3 “Breast Cancer Diagnosis Using Machine Learning Techniques” IJISET - International Journal of Innovative Science, Engineering & Technology, Vol. 5 Issue 5, May 2018 ISSN (Online) 2348 – 7968