Comparison of Six Color Models for Variety Identification of Four Paddy Grains
Archana Chaugule1
Section:Research Paper, Product Type: Journal Paper
Volume-7 ,
Issue-4 , Page no. 386-394, Apr-2019
CrossRef-DOI: https://doi.org/10.26438/ijcse/v7i4.386394
Online published on Apr 30, 2019
Copyright © Archana Chaugule . This is an open access article distributed under the Creative Commons Attribution License, which permits unrestricted use, distribution, and reproduction in any medium, provided the original work is properly cited.
View this paper at Google Scholar | DPI Digital Library
How to Cite this Paper
- IEEE Citation
- MLA Citation
- APA Citation
- BibTex Citation
- RIS Citation
IEEE Style Citation: Archana Chaugule, “Comparison of Six Color Models for Variety Identification of Four Paddy Grains,” International Journal of Computer Sciences and Engineering, Vol.7, Issue.4, pp.386-394, 2019.
MLA Style Citation: Archana Chaugule "Comparison of Six Color Models for Variety Identification of Four Paddy Grains." International Journal of Computer Sciences and Engineering 7.4 (2019): 386-394.
APA Style Citation: Archana Chaugule, (2019). Comparison of Six Color Models for Variety Identification of Four Paddy Grains. International Journal of Computer Sciences and Engineering, 7(4), 386-394.
BibTex Style Citation:
@article{Chaugule_2019,
author = {Archana Chaugule},
title = {Comparison of Six Color Models for Variety Identification of Four Paddy Grains},
journal = {International Journal of Computer Sciences and Engineering},
issue_date = {4 2019},
volume = {7},
Issue = {4},
month = {4},
year = {2019},
issn = {2347-2693},
pages = {386-394},
url = {https://www.ijcseonline.org/full_paper_view.php?paper_id=4047},
doi = {https://doi.org/10.26438/ijcse/v7i4.386394}
publisher = {IJCSE, Indore, INDIA},
}
RIS Style Citation:
TY - JOUR
DO = {https://doi.org/10.26438/ijcse/v7i4.386394}
UR - https://www.ijcseonline.org/full_paper_view.php?paper_id=4047
TI - Comparison of Six Color Models for Variety Identification of Four Paddy Grains
T2 - International Journal of Computer Sciences and Engineering
AU - Archana Chaugule
PY - 2019
DA - 2019/04/30
PB - IJCSE, Indore, INDIA
SP - 386-394
IS - 4
VL - 7
SN - 2347-2693
ER -
![]() |
![]() |
![]() |
376 | 279 downloads | 160 downloads |
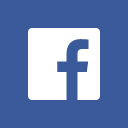
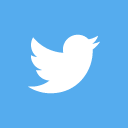
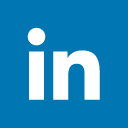
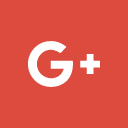
Abstract
The performances of six color models and their features are compared for classification of Karjat-6, Karjat-2, Ratnagiri-4 and Ratnagiri-24 the four paddy varieties. Total of 15 color features-mean, standard deviation, variance, skewness and kurtosis for each channel are extracted from the high-resolution images of kernels and used as input features for classification. Different feature models consisting of the combination of the above features (MSVSK and MSV) are tested for their ability to classify these cereal grains. Effect of using different features on the accuracy of classification is studied. The most suitable feature from the feature set for accurate classification is identified. The accuracy percentage for YCbCr-MSVSK1 is 71.2 % and YCbCr –MSV1 is 65.4%. The MSVSK feature set outperformed the MSV feature set in most of the instances of classification. Similarly YCbCr color model performed well as compared to rest of the color models.
Key-Words / Index Term
Mean, Neural-Network, Standard-deviation, Skewness, Kurtosis and Variance
References
[1] Archana Chaugule and Suresh N. Mali, “Evaluation of Texture and Shape Features for Classification of Four Paddy Varieties,” Hindawi Publishing Corporation, Journal of Engineering, Vol 2014, Article ID 617263, 8 pages, http://dx.doi.org/10.1155/2014/617263.
[2] Archana Chaugule and Dr. Suresh Mali, “Seed Technological Development – A Survey,” ACEEE, Proc. of International Conference on Information Technology in Signal and Image Processing, doi:03.LSCS.2013.6.528 , pp. 71-78, 2013.
[3] Cao Weishi, Zhang Chunqing, Wang Jinxing, Liu shuangxi, and Xu Kingzhen, “Purity Identification of Maize Seed Based on Discrete Wavelet Transform and BP Neural Network,” Transactions of the chinese society of Agricultural Engineering, vol. 28, pp. 253-258, 2012.
[4] Chandra B. Singh, Digvir S. Jayas, Jitendra Paliwal, Noel D.G. White, “Identification of insect-damaged wheat kernels using short-wave near-infrared hyperspectral and digital color imaging,” Elsevier, Computers and Electronics in Agriculture, vol. 73, pp. 118–125, 2010.
[5] H.K. Mebatsion, J. Paliwal , D.S. Jayas, “Automatic classification of non-touching cereal grains in digital images using limited morphological and color features,” Elsevier, Computers and Electronics in Agriculture, vol. 90, pp. 99–105, 2013.
[6] J. Paliwal; N.S. Visen; D.S. Jayas; N.D.G. White, “Cereal Grain and Dockage Identification using Machine Vision,” PH - Postharvest Technology, Biosystems Engineering, vol. 85, no. 1, pp. 51–57, 2003a.
[7] J. Paliwal; N.S. Visen; D.S. Jayas; N.D.G. White, “Comparison of a Neural Network and a Non-parametric Classifier for Grain Kernel Identification,” AE- Automation and Emerging Technologies, Biosystems Engineering, vol. 85, no. 4, pp. 405–413, 2003b.
[8] K. Kiratiratanapruk and W. Sinthupinyo, “Color and texture for corn seed classification by machine vision,” in Proceedings of the 19th IEEE International Symposium on Intelligent Signal Processing and Communication Systems (ISPACS ’11), Chiang Mai, Thailand, December 2011.
[9] Li Jingbin, Chen Bingqi, ,Shao Luhao,Tian Xushun, Kan Za, “Variety Identification of Delinted Cottonseeds Based on BP Neural Network,” Transactions of the Chinese society of Agricultural Engineering, vol. 28, pp. 265-269, 2012.
[10] Marian Wiwart, Elzbieta Suchowilska, Waldemar Lajszner, ukasz Graban, “Identification of Hybrids of Spelt and Wheat and their Parental Forms Using Shape and Color
[11] Descriptors,” Elsevier, Computers and Electronics in Agriculture, vol. 83, pp. 68-76, 2012.
[12] M. Zhao,W.Wu, Y. Q. Zhang, and X. Li, “Combining genetic algorithm and SVM for corn variety identification,” in Proceedings of the International Conference onMechatronic Science, Electric Engineering and Computer (MEC ’11), pp. 990–993, Jilin, China, August 2011.
[13] N. S. Visen, J. Paliwal, D. S. Jayas, and N.D.G.White, “Specialist neural networks for cereal grain classification,” Biosystems Engineering, vol. 82, no. 2, pp. 151–159, 2001.
[14] Pablo M. Granitto, Hugo D. Navone, Pablo F. Verdes, H.A. Ceccatto, “Weed Seeds Identification by Machine Vision,” Elsevier, Computers and Electronics in Agriculture, vol. 33, pp. 91-103, 2002.
[15] Pablo M. Granitto, Pablo F. Verdes, H. Alejandro Ceccatto, “Scale Investigation of Weed Seed Identification by Machine Vision,” Elsevier, Computers and Electronics in Agriculture, vol. 47, pp. 15-24, 2005.
[16] Xiao Chena, Yi Xunb, Wei Li, Junxiong Zhang, “Combining Discriminant Analysis and Neural Networks for Corn Variety Identification,” Elsevier, Computers and Electronics in Agriculture, vol. 71S, pp. S48-S53, 2010.
[17] B.P. Dubey, S.G. Bhagwat, S.P. Shouche, and J.K. Sainis, “Potential of Artificial Neural Networks in Varietal Identification using Morphometry of Wheat Grains,” Elsevier, Biosystems Engineering, PH—Postharvest Technology, vol. 95, no.1, pp. 61–67, 2006.