Linear Norm Tree Based Least Square TSVM Scheme for Wilt Diseased Trees Classification
Mayank Arya Chandra1 , S S Bedi2
Section:Research Paper, Product Type: Journal Paper
Volume-7 ,
Issue-4 , Page no. 13-17, Apr-2019
CrossRef-DOI: https://doi.org/10.26438/ijcse/v7i4.1317
Online published on Apr 30, 2019
Copyright © Mayank Arya Chandra, S S Bedi . This is an open access article distributed under the Creative Commons Attribution License, which permits unrestricted use, distribution, and reproduction in any medium, provided the original work is properly cited.
View this paper at Google Scholar | DPI Digital Library
How to Cite this Paper
- IEEE Citation
- MLA Citation
- APA Citation
- BibTex Citation
- RIS Citation
IEEE Style Citation: Mayank Arya Chandra, S S Bedi, “Linear Norm Tree Based Least Square TSVM Scheme for Wilt Diseased Trees Classification,” International Journal of Computer Sciences and Engineering, Vol.7, Issue.4, pp.13-17, 2019.
MLA Style Citation: Mayank Arya Chandra, S S Bedi "Linear Norm Tree Based Least Square TSVM Scheme for Wilt Diseased Trees Classification." International Journal of Computer Sciences and Engineering 7.4 (2019): 13-17.
APA Style Citation: Mayank Arya Chandra, S S Bedi, (2019). Linear Norm Tree Based Least Square TSVM Scheme for Wilt Diseased Trees Classification. International Journal of Computer Sciences and Engineering, 7(4), 13-17.
BibTex Style Citation:
@article{Chandra_2019,
author = {Mayank Arya Chandra, S S Bedi},
title = {Linear Norm Tree Based Least Square TSVM Scheme for Wilt Diseased Trees Classification},
journal = {International Journal of Computer Sciences and Engineering},
issue_date = {4 2019},
volume = {7},
Issue = {4},
month = {4},
year = {2019},
issn = {2347-2693},
pages = {13-17},
url = {https://www.ijcseonline.org/full_paper_view.php?paper_id=3986},
doi = {https://doi.org/10.26438/ijcse/v7i4.1317}
publisher = {IJCSE, Indore, INDIA},
}
RIS Style Citation:
TY - JOUR
DO = {https://doi.org/10.26438/ijcse/v7i4.1317}
UR - https://www.ijcseonline.org/full_paper_view.php?paper_id=3986
TI - Linear Norm Tree Based Least Square TSVM Scheme for Wilt Diseased Trees Classification
T2 - International Journal of Computer Sciences and Engineering
AU - Mayank Arya Chandra, S S Bedi
PY - 2019
DA - 2019/04/30
PB - IJCSE, Indore, INDIA
SP - 13-17
IS - 4
VL - 7
SN - 2347-2693
ER -
![]() |
![]() |
![]() |
767 | 625 downloads | 207 downloads |
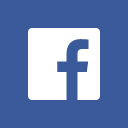
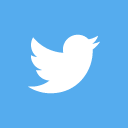
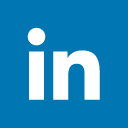
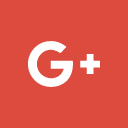
Abstract
The latest generation of Least Square Twin Support Vector Machine has achieved amazing outcomes in the field of image classification. This paper presents a new method Linear Norms Tree based Least Square Twin Support Vector Machine for developing a plant disease recognition system, which is based on high-resolution multispectral satellite imagery. This proposed model can identify and classify different kinds of plant diseases. Dataset is a composition of diseased trees and other land cover. That are used to identify whether the tree is diseased or not. Experiments with wilt disease data set carried out indicate that new classifier, Linear Norms Tree based Least Square Twin Support Vector Machine, yields a progressively balanced classification accuracy between classes compared to different classification schemes in resolving the imbalanced classification problem.
Key-Words / Index Term
Twin SVM, SVM, Norm, wilt, diseases, least square, imbalance data
References
[1] K. A. Garrett, S. P. Dendy, E. E. Frank, M. N. Rouse, and S. E. Travers, “Climate change effects on plant disease: genomes to ecosystems,” Annual Review of Phytopathology, Vol. 44, pp. 489–509, 2006.
[2] J. G. Arnal Barbedo, “Digital image processing techniques for detecting, quantifying and classifying plant diseases,” SpringerPlus, Vol. 2, article 660, pp. 1–12, 2013.
[3] Srdjan Sladojevic, Marko Arsenovic, Andras Anderla, Dubravko Culibrk, and Darko Stefanovic, “Deep Neural Networks Based Recognition of Plant Diseases by Leaf Image Classification,” Computational Intelligence and Neuroscience, Vol. 2016, pp 1-11, 2016..
[4] L. Kurvonen, M.T. Hallikainen, “Textural Information of Multitemporal ERS-1 and JERS-1 SAR Images with Applications to Land and Forest Type Classification in Boreal Zone,” IEEE Transactions On Geoscience And Remote Sensing, Vol. 37(2) , pp. 680-689,1999.
[5] N. Kosaka, T. Akiyama, B. Tsai, T. Kojima, “Forest type classification using data fusion of multispectral and panchromatic high-resolution satellite imageries,” In International Geoscience and Remote Sensing Symposium, Vol. 4, pp. 2980- 2983, 2005.
[6] X.Yang, N. Rochdi, J Zhang, J. Banting, D. Rolfson, C King, B. Purdy, “Mapping tree species in a boreal forest area using RapidEye and LiDAR data,” In Geoscience and Remote Sensing Symposium (IGARSS), IEEE International, pp. 69-71, 2014.
[7] M.S.M. Pozi, M. N. Sulaiman, N. Mustapha and T. Perumal, “A new classification model for a class imbalanced data set using genetic programming and support vector machines: case study for wilt disease classification,” Remote Sensing Letters, 6:7, 568-577, 2015.
[8] M. A. Chandra & S. S. Bedi, “Survey on SVM and their application in image classification,” International Journal of Information Technology, pp 1–11, 2018.
[9] C. Cortes and V. Vapnik, “Support-vector network,” Machine Learning 20(3):273–297, 1995.
[10] S. Tao, D Chen, W. Zhao, “Fast pruning algorithm for multi-output LS-SVM and its application in chemical pattern classification,” Chemometrics and Intelligent Laboratory Systems - hemometrics and Intelligent Laboratory Systems Vol.96 (1), pp. 63-69, 2009.
[11] O. L. Mangasarian and David R. Musicant, “Lagrangian Support Vector Machines” Journal of Machine Learning Research Vol. 1, pp. 161-177, 2001.
[12] J. A. K Suykens and J. Vandewalle, “Least squares support vector machine classifiers,” Neural Process, Letter. 9, pp. 293-300, 1999.
[13] Jayadeva, R. Khemchandani, S. Chandra, “Twin support vector machines for pattern classification”, IEEE Transactions on Pattern Analysis and Machine Intelligence, Vol. 29, Issue 5, pp. 905–910, 2007.
[14] B. Johnson, R. Tateishi, N. Hoan, A hybrid pansharpening approach and multiscale object-based image analysis for mapping diseased pine and oak trees, International Journal of Remote Sensing, Vol. 34(20), pp. 6969-6982, 2013.
[15] J. G. Arnal Barbedo, Digital image processing techniques for detecting, quantifying and classifying plant diseases, Springer-Plus, Vol. 2, article 660, pp. 1–12, 2013.
[16] A. K. Mahlein, T. Rumpf, P. Welke et al., Development of spectral indices for detecting and identifying plant diseases, Remote Sensing of Environment, Vol. 128, pp. 21–30, 2013.
[17] W. Xiuqing,W. Haiyan, and Y. Shifeng, Plant disease detection based on near-field acoustic holography, Transactions of the Chinese Society for Agricultural Machinery, Vol. 2, article 43,2014.
[18] J. K. Patil and R. Kumar, “Feature extraction of diseased leaf images,” Journal of Signal & Image Processing, Vol. 3, no. 1, pp. 60, 2012.
[19] T. Rumpf, A.-K.Mahlein, U. Steiner, E.-C. Oerke, H.-W. Dehne, and L. Pl¨umer, “Early detection and classification of plant diseases with Support VectorMachines based on hyperspectral reflectance,” Computers and Electronics in Agriculture, Vol. 74, no. 1, pp. 91–99, 2010.
[20] A. Anagaw, and YL Chang, “A new complement naïve Bayesian approach for biomedical data classification,” Journal of Ambient Intelligence and Humanized Computing pp 1–9 (2018).
[21] M F Unlersen and K. Sabanci, “The Classification of Diseased Trees by Using kNN and MLP Classification Models According to the Satellite Imagery,” International Journal of Intelligent Systems and Applications in Engineering, Vol 4, No 2 (2016).
[22] D. Sheela Jeyarani, A. Pethalakshmi, “An Efficient Fuzzy Entropy Based Feature Selection Algorithm for High Dimensional Data,” International Journal of Advanced Research in Computer Science, Vol. 6, No. 6, June - July 2015.
[23] U Mahdiyah, M. I. Irawan, E. Imah, “Integrating Data Selection and Extreme Learning Machine for Imbalanced Data,” International Conference on Computer Science and Computational Intelligence (ICCSCI 2015), Procedia Computer Science 59, 221 – 229, 2015.
[24] C. K. Reddy, D. Hiranmayi, K. Eswaran, G. G. Likhita, B.Saritha, “Classification of Diseased Plants using Separation of Points by Planes,” International Journal of Engineering Technology Science and Research, Vol. 4, Issue 9 September 2017.
[25] D. Tomar, S. Agarwal, “Twin Support Vector Machine: A review from 2007 to 2014,” Egyptian Informatics Journal Volume 16, Issue 1, March 2015, Pages 55-69.
[26] M A. Kumar, M, Gopal, “Least squares twin support vector machines for pattern classification,” Expert System Appl 36(3):7535– 7543, 2009
[27] M.A. Chandra and S.S. Bedi, “Benchmarking tree-based least squares twin support vector machine classifiers,” International Journal of Business Intelligence and Data Mining.10.1504/IJBIDM.2018.10009883.