Rhopalocera Optimization Algorithm Based Attribute Selection With Improved Fuzzy Artificial Neural Network (ROA - IFANN) Classifier for Coronary Artery Heart Disease Prediction in Diabetes Patients
B. Narasimhan1 , A. Malathi2
Section:Research Paper, Product Type: Journal Paper
Volume-7 ,
Issue-3 , Page no. 926-935, Mar-2019
CrossRef-DOI: https://doi.org/10.26438/ijcse/v7i3.926935
Online published on Mar 31, 2019
Copyright © B. Narasimhan, A. Malathi . This is an open access article distributed under the Creative Commons Attribution License, which permits unrestricted use, distribution, and reproduction in any medium, provided the original work is properly cited.
View this paper at Google Scholar | DPI Digital Library
How to Cite this Paper
- IEEE Citation
- MLA Citation
- APA Citation
- BibTex Citation
- RIS Citation
IEEE Citation
IEEE Style Citation: B. Narasimhan, A. Malathi, “Rhopalocera Optimization Algorithm Based Attribute Selection With Improved Fuzzy Artificial Neural Network (ROA - IFANN) Classifier for Coronary Artery Heart Disease Prediction in Diabetes Patients,” International Journal of Computer Sciences and Engineering, Vol.7, Issue.3, pp.926-935, 2019.
MLA Citation
MLA Style Citation: B. Narasimhan, A. Malathi "Rhopalocera Optimization Algorithm Based Attribute Selection With Improved Fuzzy Artificial Neural Network (ROA - IFANN) Classifier for Coronary Artery Heart Disease Prediction in Diabetes Patients." International Journal of Computer Sciences and Engineering 7.3 (2019): 926-935.
APA Citation
APA Style Citation: B. Narasimhan, A. Malathi, (2019). Rhopalocera Optimization Algorithm Based Attribute Selection With Improved Fuzzy Artificial Neural Network (ROA - IFANN) Classifier for Coronary Artery Heart Disease Prediction in Diabetes Patients. International Journal of Computer Sciences and Engineering, 7(3), 926-935.
BibTex Citation
BibTex Style Citation:
@article{Narasimhan_2019,
author = {B. Narasimhan, A. Malathi},
title = {Rhopalocera Optimization Algorithm Based Attribute Selection With Improved Fuzzy Artificial Neural Network (ROA - IFANN) Classifier for Coronary Artery Heart Disease Prediction in Diabetes Patients},
journal = {International Journal of Computer Sciences and Engineering},
issue_date = {3 2019},
volume = {7},
Issue = {3},
month = {3},
year = {2019},
issn = {2347-2693},
pages = {926-935},
url = {https://www.ijcseonline.org/full_paper_view.php?paper_id=3941},
doi = {https://doi.org/10.26438/ijcse/v7i3.926935}
publisher = {IJCSE, Indore, INDIA},
}
RIS Citation
RIS Style Citation:
TY - JOUR
DO = {https://doi.org/10.26438/ijcse/v7i3.926935}
UR - https://www.ijcseonline.org/full_paper_view.php?paper_id=3941
TI - Rhopalocera Optimization Algorithm Based Attribute Selection With Improved Fuzzy Artificial Neural Network (ROA - IFANN) Classifier for Coronary Artery Heart Disease Prediction in Diabetes Patients
T2 - International Journal of Computer Sciences and Engineering
AU - B. Narasimhan, A. Malathi
PY - 2019
DA - 2019/03/31
PB - IJCSE, Indore, INDIA
SP - 926-935
IS - 3
VL - 7
SN - 2347-2693
ER -
![]() |
![]() |
![]() |
384 | 368 downloads | 216 downloads |
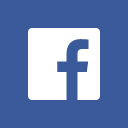
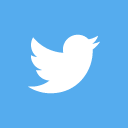
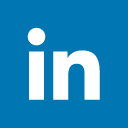
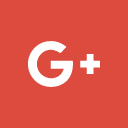
Abstract
Soft computing techniques and its applications extends its wings in almost all areas which includes data mining, pattern discovery, industrial applications, robotics, automation and many more. Soft computing comprises of the core components such as fuzzy logic, genetic algorithm, artificial neural networks and probabilistic reasoning. In spite of these, recently many bio – inspired computing attracted attention for the researchers to work in that area. Machine learning plays an important role in the design and development of decision support systems, applied soft computing and expert systems applications. Attribute selection is conducted by Rhopalocera optimization algorithm which mimic the features of butterfly optimization algorithm. After that an improved fuzzy logic based artificial neural network classifier for predicting coronary artery heart disease among diabetic patients is developed. Real time data are obtained and the built ROA - IFANN classifier is tested for performance in terms of prediction accuracy, sensitivity, specificity and Mathew’s correlation coefficient. The significance of MCC is that to test the ability of the machine learning classifier in spite of other performance metrics. Implementations are done in Scilab and from the obtained results it is inferred that the built ROA - IFANN outperforms that that of other classifiers.
Key-Words / Index Term
soft computing, fuzzy logic, machine learning, CAHD, diabetes, artificial neural network, applications of soft computing
References
[1] D. Gupta, S. Sundaram, A. Khanna, A. E. Hassanien, V. H. Albuquerque, Improved diagnosis of Parkinson`s disease using optimized crow search algorithm, Computers & Electrical Engineering, Vol 68, pp 412-424, 2018.
[2] P. Samanta, A. Pathak, K. Mandana, G. Saha, Classification of coronary artery diseased and normal subjects using multi-channel phonocardiogram signal, Biocybernetics and Biomedical Engineering, 2019.
[3] Z. Zhang, J. Dong, X. Luo, K. Choi, X. Wu, Heartbeat classification using disease-specific feature selection, Computers in Biology and Medicine, Vol 46, pp 79-89, 2014.
[4] M. Esmaelian, H. Shahmoradi, M. Vali, A novel classification method: A hybrid approach based on extension of the UTADIS with polynomial and PSO-GA algorithm, Applied Soft Computing, Vol 49, pp 56-70, 2016.
[5] V. M. Guerra, J. Novo, J. Rouco, M. G. Penedo, M. Ortega, Heartbeat classification fusing temporal and morphological information of ECGs via ensemble of classifiers, Biomedical Signal Processing and Control, Vol 47, pp 41-48, 2019.
[6] L. Hu, C. Yin, S. Ma, Z. Liu, Rapid detection of three quality parameters and classification of wine based on Vis-NIR spectroscopy with wavelength selection by ACO and CARS algorithms, Spectrochimica Acta Part A: Molecular and Biomolecular Spectroscopy, Vol 205, pp 574-581, 2018.
[7] G. Sannino, G. D. Pietro, A deep learning approach for ECG-based heartbeat classification for arrhythmia detection, Future Generation Computer Systems, Vol 86, pp 446-455, 2018.
[8] M. H. Vafaie, M. Ataei, H. R. Koofigar, Heart diseases prediction based on ECG signals’ classification using a genetic-fuzzy system and dynamical model of ECG signals, Biomedical Signal Processing and Control, Vol 14, pp 291-296, 2014.
[9] H. H. Carvalho, R. L. Moreno, T. C. Pimenta, P. C. Crepaldi, E. Cintra, A heart disease recognition embedded system with fuzzy cluster algorithm, Computer Methods and Programs in Biomedicine, Vol 110, pp 447-454, 2013.
[10] Z. Arabasadi, R. Alizadehsani, M. Roshanzamir, H. Moosaei, A. A. Yarifard, Computer aided decision making for heart disease detection using hybrid neural network-Genetic algorithm, Computer Methods and Programs in Biomedicine, Vol 141, pp 19-26, 2017.
[11] S. Arora, S. Singh, "Butterfly optimization algorithm: a novel approach for global optimization", Soft Computing - A Fusion of Foundations, Methodologies and Applications, vol. 23, no. 3, pp - 715 - 734, 2019.
[12] B. Narasimhan, A. Malathi, "Artificial Lampyridae Classifier (ALC) for coronary artery heart disease prediction in diabetes patients", International Journal of Advance Research, Ideas and Innovations in Technology, vol. 5, no. 2, pp - 683 - 689, 2019.
[13] B. Narasimhan, A. Malathi, " Improved Fuzzy Artificial Neural Network (IFANN) Classifier for coronary artery heart disease prediction in diabetes patients", Indian Journal of Advanced Research, vol. 9, no.4, 2019.