A Comparative Analysis of Emotion Recognition using DEAP Dataset
Piyush Bhardwaj1 , Pratyaksha Jha2 , Nishtha Chheda3 , Suvarna Gosavi4
Section:Research Paper, Product Type: Journal Paper
Volume-7 ,
Issue-3 , Page no. 876-881, Mar-2019
CrossRef-DOI: https://doi.org/10.26438/ijcse/v7i3.876881
Online published on Mar 31, 2019
Copyright © Piyush Bhardwaj, Pratyaksha Jha, Nishtha Chheda, Suvarna Gosavi . This is an open access article distributed under the Creative Commons Attribution License, which permits unrestricted use, distribution, and reproduction in any medium, provided the original work is properly cited.
View this paper at Google Scholar | DPI Digital Library
How to Cite this Paper
- IEEE Citation
- MLA Citation
- APA Citation
- BibTex Citation
- RIS Citation
IEEE Citation
IEEE Style Citation: Piyush Bhardwaj, Pratyaksha Jha, Nishtha Chheda, Suvarna Gosavi, “A Comparative Analysis of Emotion Recognition using DEAP Dataset,” International Journal of Computer Sciences and Engineering, Vol.7, Issue.3, pp.876-881, 2019.
MLA Citation
MLA Style Citation: Piyush Bhardwaj, Pratyaksha Jha, Nishtha Chheda, Suvarna Gosavi "A Comparative Analysis of Emotion Recognition using DEAP Dataset." International Journal of Computer Sciences and Engineering 7.3 (2019): 876-881.
APA Citation
APA Style Citation: Piyush Bhardwaj, Pratyaksha Jha, Nishtha Chheda, Suvarna Gosavi, (2019). A Comparative Analysis of Emotion Recognition using DEAP Dataset. International Journal of Computer Sciences and Engineering, 7(3), 876-881.
BibTex Citation
BibTex Style Citation:
@article{Bhardwaj_2019,
author = {Piyush Bhardwaj, Pratyaksha Jha, Nishtha Chheda, Suvarna Gosavi},
title = {A Comparative Analysis of Emotion Recognition using DEAP Dataset},
journal = {International Journal of Computer Sciences and Engineering},
issue_date = {3 2019},
volume = {7},
Issue = {3},
month = {3},
year = {2019},
issn = {2347-2693},
pages = {876-881},
url = {https://www.ijcseonline.org/full_paper_view.php?paper_id=3932},
doi = {https://doi.org/10.26438/ijcse/v7i3.876881}
publisher = {IJCSE, Indore, INDIA},
}
RIS Citation
RIS Style Citation:
TY - JOUR
DO = {https://doi.org/10.26438/ijcse/v7i3.876881}
UR - https://www.ijcseonline.org/full_paper_view.php?paper_id=3932
TI - A Comparative Analysis of Emotion Recognition using DEAP Dataset
T2 - International Journal of Computer Sciences and Engineering
AU - Piyush Bhardwaj, Pratyaksha Jha, Nishtha Chheda, Suvarna Gosavi
PY - 2019
DA - 2019/03/31
PB - IJCSE, Indore, INDIA
SP - 876-881
IS - 3
VL - 7
SN - 2347-2693
ER -
![]() |
![]() |
![]() |
721 | 764 downloads | 160 downloads |
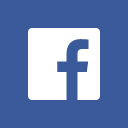
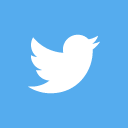
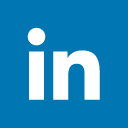
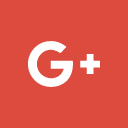
Abstract
Emotions play a crucial role in human communication and the Brain-Computer Interfaces (BCI) aid in emotion-based communication, similar to the way a mobile device aids in text-based communication. Emotions are expressed in myriad ways, including verbal, non-verbal and physiological signals. Most BCI systems accomplish this by using electroencephalography (EEG) signals. Before BCI systems are employed to practical use, efficient algorithms need to be developed in order to maximize efficiency. This paper proposes a method to map several emotional states using EEG signals collected from the publicly available Dataset for Emotion Analysis using Physiological signals (DEAP). It presents a comparative analysis of two different classification algorithms along with two different dimensionality reduction techniques. Linear Discriminant Analysis (LDA) and Principal Component Analysis (PCA) are used in tandem with Support Vector Machine (SVM) and K-Nearest Neighbours (KNN). The objective of this paper is to weigh the results to find the most promising classifier and dimensionality reduction technique. The average accuracy of binary classification using KNN-LDA for each valence, arousal, dominance and liking was 97.98%, 96.21%, 98.24% and 96.19% respectively.
Key-Words / Index Term
Emotion classification, EEG, Physiological signals, Signal processing, Pattern classification, Affective computing, DEAP Dataset, Machine Learning, Brain-Computer Interfaces (BCI), Electroencephalogram (EEG), Linear Discriminant Analysis (LDA), K-Nearest Neighbours (KNN), Support Vector Machine (SVM), Principal Component Analysis (PCA).
References
[1] T. D. Kemper and R. S. Lazarus, “Emotion and Adaptation,” Contemporary Sociology, vol. 21, no. 4. p. 522, 1992.
[2] J. Posner, J. A. Russell, and B. S. Peterson, “The circumplex model of affect: An integrative approach to affective neuroscience, cognitive development, and psychopathology,” Development and Psychopathology, vol. 17, no. 03. 2005.
[3] Y. Liu, O. Sourina, and M. K. Nguyen, “Real-Time EEG-Based Human Emotion Recognition and Visualization,” 2010 International Conference on Cyberworlds. 2010.
[4] R. Plutchik, “The Nature of Emotions: Clinical Implications,” Emotions and Psychopathology. pp. 1–20, 1988.
[5] D. Garrett, D. A. Peterson, C. W. Anderson, and M. H. Thaut, “Comparison of linear, nonlinear, and feature selection methods for EEG signal classification,” IEEE Trans. Neural Syst. Rehabil. Eng., vol. 11, no. 2, pp. 141–144, Jun. 2003.
[6] M. Soleymani, M. Pantic, and T. Pun, “Multimodal emotion recognition in response to videos (Extended abstract),” 2015 International Conference on Affective Computing and Intelligent Interaction (ACII). 2015.
[7] R. Nivedha, M. Brinda, D. Vasanth, M. Anvitha, and K. V. Suma, “EEG based emotion recognition using SVM and PSO,” 2017 International Conference on Intelligent Computing, Instrumentation and Control Technologies (ICICICT). 2017.
[8] A.S. Mali, A.A. Kenjale, P.M. Ghatage, A.G. Deshpande, "Mood based Music System", International Journal of Scientific Research in Computer Science and Engineering, Vol.6, Issue.3, pp.27-30, 2018
[9] Afzal Ahmad, Mohammad Asif, Shaikh Rohan Ali, "Review Paper on Predicting Mood Disorder Risk Using Machine Learning", International Journal of Scientific Research in Computer Science and Engineering, Vol.7, Issue.1, pp.16-22, 2019
[10] S. Koelstra et al., “DEAP: A Database for Emotion Analysis; Using Physiological Signals,” IEEE Transactions on Affective Computing, vol. 3, no. 1. pp. 18–31, 2012.
[11] Russell, J. A. (1980), “A circumplex model of affect,” Journal of Personality and Social Psychology, 39, December, 1161-1178.