A Survey on Student Performance using Data Mining Techniques
Zainab Fatema1 , Geeta Pattun2
Section:Survey Paper, Product Type: Journal Paper
Volume-7 ,
Issue-3 , Page no. 707-710, Mar-2019
CrossRef-DOI: https://doi.org/10.26438/ijcse/v7i3.707710
Online published on Mar 31, 2019
Copyright © Zainab Fatema, Geeta Pattun . This is an open access article distributed under the Creative Commons Attribution License, which permits unrestricted use, distribution, and reproduction in any medium, provided the original work is properly cited.
View this paper at Google Scholar | DPI Digital Library
How to Cite this Paper
- IEEE Citation
- MLA Citation
- APA Citation
- BibTex Citation
- RIS Citation
IEEE Style Citation: Zainab Fatema, Geeta Pattun, “A Survey on Student Performance using Data Mining Techniques,” International Journal of Computer Sciences and Engineering, Vol.7, Issue.3, pp.707-710, 2019.
MLA Style Citation: Zainab Fatema, Geeta Pattun "A Survey on Student Performance using Data Mining Techniques." International Journal of Computer Sciences and Engineering 7.3 (2019): 707-710.
APA Style Citation: Zainab Fatema, Geeta Pattun, (2019). A Survey on Student Performance using Data Mining Techniques. International Journal of Computer Sciences and Engineering, 7(3), 707-710.
BibTex Style Citation:
@article{Fatema_2019,
author = {Zainab Fatema, Geeta Pattun},
title = {A Survey on Student Performance using Data Mining Techniques},
journal = {International Journal of Computer Sciences and Engineering},
issue_date = {3 2019},
volume = {7},
Issue = {3},
month = {3},
year = {2019},
issn = {2347-2693},
pages = {707-710},
url = {https://www.ijcseonline.org/full_paper_view.php?paper_id=3904},
doi = {https://doi.org/10.26438/ijcse/v7i3.707710}
publisher = {IJCSE, Indore, INDIA},
}
RIS Style Citation:
TY - JOUR
DO = {https://doi.org/10.26438/ijcse/v7i3.707710}
UR - https://www.ijcseonline.org/full_paper_view.php?paper_id=3904
TI - A Survey on Student Performance using Data Mining Techniques
T2 - International Journal of Computer Sciences and Engineering
AU - Zainab Fatema, Geeta Pattun
PY - 2019
DA - 2019/03/31
PB - IJCSE, Indore, INDIA
SP - 707-710
IS - 3
VL - 7
SN - 2347-2693
ER -
![]() |
![]() |
![]() |
777 | 271 downloads | 129 downloads |
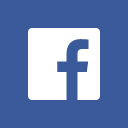
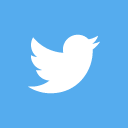
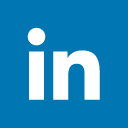
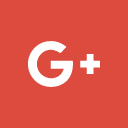
Abstract
Students are the main stakeholders of institutions and their performance plays a significant role in country development. The aim of institutions is to give excellence educations to their students. It has been observed in the previous works, students of slow performance and dropouts are the most vital issues. Due to early detection of slow performers and dropouts of students, help the teachers, administrator, and management to take appropriate actions at the right time for improving the overall performance of the students. The purpose of this study is to analyze different data mining and machine learning techniques on student data and find which technique gives better accuracy. And, we also find different factors like socio-demographic, psychological factors, attendance of students, understanding level of students, previous grades, study time, parent’s status, internet usage, travel time, extracurricular activities, and also health factors affect the performance of students. Data mining is a process of analyzing data and turns it into useful information.
Key-Words / Index Term
Students Academic Performance, Data Mining, Machine Learning
References
[1] A. Sethi, “Data Mining for Prediction and Classification of Engineering Students achievements using Improved Naïve Bayes”, International Journal of Advanced Research in Computer Engineering & Technology (IJARCET) Vol. 6, Issue 7, pp. 966-971, 2017.
[2] E. Osmanbegović, “Data Mining Approach for Predicting Student Performance”, Economic Review – Journal of Economics and Business, Vol. X, Issue 1, pp.3-12, May 2012.
[3] B. K. Baradwaj, “Mining Educational Data to Analyze Students‟ Performance”, (IJACSA) International Journal of Advanced Computer Science and Applications, Vol. 2, No. 6, pp. 63-69, 2011.
[4] F. Marbouti, “Models for early prediction of at-risk students in a course using standards-based grading”, ELSEVIER, Vol. 103, pp.1-15, 2016.
[5] P. Kaur, “Classification and prediction based data mining algorithms to predict slow learners in education sector”, 3rd International Conference on Recent Trends in Computing 2015(ICRTC-2015), Ghaziabad, India, pp.500-508, 2015.
[6] M. Ramaswami, “A CHAID Based Performance Prediction Model in Educational Data Mining”, International Journal of Computer Science Issues, Vol.7, Issue.1, pp.10-18, 2010.
[7] V. P. Desai, “Classification Technique for Predicting Learning Behavior of Student in Higher Education”, International Conference on Digital Economy and its Impact on Business and Industry, Sangli, India, pp.163-166, 2018.
[8] R. Sumitha, “Prediction of Students Outcome using Data Mining Techniques”, International Journal of Scientific Engineering and Applied Science ,Vol.2, Issue.6, pp.132-139, 2016.
[9] A. Mueen, “Modeling and Predicting Students’ Academic Performance Using Data Mining Techniques”, I.J. Modern Education and Computer Science, Vol.8, No.11, pp.36-42, 2016.
[10] A. Daud, “Predicting Student Performance using Advanced Learning Analytics”, International World Wide Web Conference Committee, Perth, Australia, pp.415-421, 2017.
[11] H. Shaziya, “Prediction of Students Performance in Semester Exams using a Naïve Bayes Classifier”, International Journal of Innovative Research in Science, Engineering and Technology, Vol.4, Issue.10, pp.9823-9829, 2015.
[12] M. Zahedifard, “Prediction of Students Performance in High School by Data Mining Classification Techniques”, International Academic Journal of Science and Engineering Vol. 2, No. 7, pp.25-33, 2015.
[13] K. P. Rao, “Predicting Learning Behavior of Students using Classification Techniques”, International Journal of Computer Applications, Vol.139, No7, pp.15-19, 2016.
[14] Swati, “Using Factor Classification for the Slow Learner Prediction over Various Class of Student Dataset”, Indian Journal of Science and Technology, Vol.9, Issue.48, pp.1-5, 2016.
[15] M. Kumar, “Recognition of Slow Learners using Classification Data Mining Techniques”, Imperial Journal of Interdisciplinary Research, Vol.2, Issue.12, pp.741-747, 2016.
[16] F. Marbouti, “Models for early prediction of at-risk students in a course using standards-based grading”, ELSEVIER, Vol.103, pp.1-15, 2016.
[17] Q. A. Al-Radaideh, “Mining Student Data using Decision Trees”, International Arab Conference on Information Technology, Jordan, Arab, 2006.
[18] V. Ramesh, “Predicting Student Performance: A statistical and Data Mining Approach”, International Journal of Computer Applications, Vol.63, No.8, 2013.