Street Traffic Forecasting: Ongoing Advances and New Challenges
Mohd Azeem Ansari1 , T. Arundhathi2
Section:Research Paper, Product Type: Journal Paper
Volume-7 ,
Issue-3 , Page no. 650-656, Mar-2019
CrossRef-DOI: https://doi.org/10.26438/ijcse/v7i3.650656
Online published on Mar 31, 2019
Copyright © Mohd Azeem Ansari, T. Arundhathi . This is an open access article distributed under the Creative Commons Attribution License, which permits unrestricted use, distribution, and reproduction in any medium, provided the original work is properly cited.
View this paper at Google Scholar | DPI Digital Library
How to Cite this Paper
- IEEE Citation
- MLA Citation
- APA Citation
- BibTex Citation
- RIS Citation
IEEE Style Citation: Mohd Azeem Ansari, T. Arundhathi, “Street Traffic Forecasting: Ongoing Advances and New Challenges,” International Journal of Computer Sciences and Engineering, Vol.7, Issue.3, pp.650-656, 2019.
MLA Style Citation: Mohd Azeem Ansari, T. Arundhathi "Street Traffic Forecasting: Ongoing Advances and New Challenges." International Journal of Computer Sciences and Engineering 7.3 (2019): 650-656.
APA Style Citation: Mohd Azeem Ansari, T. Arundhathi, (2019). Street Traffic Forecasting: Ongoing Advances and New Challenges. International Journal of Computer Sciences and Engineering, 7(3), 650-656.
BibTex Style Citation:
@article{Ansari_2019,
author = {Mohd Azeem Ansari, T. Arundhathi},
title = {Street Traffic Forecasting: Ongoing Advances and New Challenges},
journal = {International Journal of Computer Sciences and Engineering},
issue_date = {3 2019},
volume = {7},
Issue = {3},
month = {3},
year = {2019},
issn = {2347-2693},
pages = {650-656},
url = {https://www.ijcseonline.org/full_paper_view.php?paper_id=3897},
doi = {https://doi.org/10.26438/ijcse/v7i3.650656}
publisher = {IJCSE, Indore, INDIA},
}
RIS Style Citation:
TY - JOUR
DO = {https://doi.org/10.26438/ijcse/v7i3.650656}
UR - https://www.ijcseonline.org/full_paper_view.php?paper_id=3897
TI - Street Traffic Forecasting: Ongoing Advances and New Challenges
T2 - International Journal of Computer Sciences and Engineering
AU - Mohd Azeem Ansari, T. Arundhathi
PY - 2019
DA - 2019/03/31
PB - IJCSE, Indore, INDIA
SP - 650-656
IS - 3
VL - 7
SN - 2347-2693
ER -
![]() |
![]() |
![]() |
488 | 338 downloads | 131 downloads |
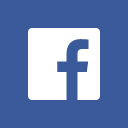
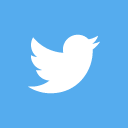
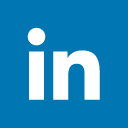
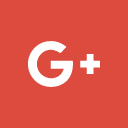
Abstract
In metropolitan cities traffic congestion became a severe issue due to large scale multiple-layer road networks. The multifaceted nature, heterogeneity of traffic framework and the enormous information challenge have turned out to be generous troubles. The current transportation systems deal with these issues with the requirement of qualified overall prediction accuracy. Checking, foreseeing and understanding traffic conditions in any city is a vital issue for city arranging. More recently, the development of new technology for traffic data processing using big data for accurate traffic prediction has shifted the spotlight to data-driven procedures. Different researchers build traffic forecasting systems using big data analytics in order to prevent traffic congestion and accident issues. However, most of the researchers focus on the prediction of individual road segments or intersections instead of the multilayer roads. This paper is an attempt to review the different techniques used by numerous researchers for traffic forecasting using big data analytics. The ultimate goal of this work is to set an updated compilation of prior literature around traffic prediction models so as to motivate and guide future research on this vibrant field.
Key-Words / Index Term
Traffic Forecasting system, Big Data Analytics, Smart Transport System (STS)
References
[1] E. I. Vlahogianni, M. G. Karlaftis, and J. C. Golias, “Short-term traffic forecasting: Where we are and where we’re going,” Transport. Res. Part C Emerging Technol., vol. 43, pp. 3–19, 2014
[2] B. Van Arem, H. R. Kirby, M. J. M. Van Der Vlist, and J. C. Whittaker, “Recent advances and applications in the field of short-term traffic forecasting,” Int. J. Forecasting, vol. 13, no. 1, pp. 1–12, 1997.
[3] B. Van Arem, H. R. Kirby, M. J. M. Van Der Vlist, and J. C. Whittaker, “Recent advances and applications in the field of short-term traffic forecasting,” Int. J. Forecasting, vol. 13, no. 1, pp. 1–12, 1997.
[4] E. I. Vlahogianni, J. C. Golias, and M. G. Karlaftis, “Short-term traffic forecasting: Overview of objectives and methods,” Transport Rev., vol. 24, no. 5, pp. 533–557, 2004.
[5] .Ensemble Learning based Urban Traffic State Prediction for Coupling Traffic Network with Large Scale Data*
[6] Urban track modeling and prediction using large scale taxi GPS traces.
[7] DxNAT - Deep Neural Networks for Explaining Non-Recurring Traffic Congestion
[8] .Medium-term Prediction of Urban Traffic States Using Probability Tree
[9] .RECURSIVE PREDICTION OF TRAFFIC CONDITIONS WITH NEURAL NETWORK MODELS
[10] TRAFFIC FLOW PREDICTIONS EMPLOYING NEURAL NETWORKS IN A NOVEL TRAFFIC FLOW REGIME SEPARATION TECHNIQUE
[11] C. van Hinsbergen, J. van Lint, and F. Sanders, “Short term traffic prediction models,” in Proc. 14th World Congress Intelligent Transportation Systems, Beijing, 2007.
[12] H. J. van Lint and C. P. van Hinsbergen, “Short-term traffic and travel time prediction models,” Artif. Intell. Applicat. Crit. Transport. Issues, vol. 22, pp. 22–41, 2012.
[13] E. Bolshinsky and R. Freidman, “Traffic flow forecast survey,” Technion—Israel Institute of Technology, Tech. Rep., 2012.
[14] E. I. Vlahogianni, M. G. Karlaftis, and J. C. Golias, “Short-term traffic forecasting: Where we are and where we’re going,” Transport. Res. Part C Emerging Technol., vol. 43, pp. 3–19, 2014.
[15] Z. Ma, G. Luo, and D. Huang, “Short term traffic flow prediction based on on-line sequential extreme learning machine,” in Proc. 8th Int. Conf. Advanced Computational Intelligence, Chiang Mai, 2016, pp. 143–149.
[16] A. Wibisono, W. Jatmiko, H. A. Wisesa, B. Hardjono, and P. Mursanto, “Traffic big data prediction and visualization using fast incremental model trees-drift detection (FIMT-DD),” Knowledge-Based Syst., vol. 93, pp. 33–46, 2016.
[17] U. Mori, A. Mendiburu, M. Álvarez, and J. A. Lozano, "A review of travel time estimation and forecasting for advanced traveler information systems," Transportmetrica A Transport Sci., vol. 11, no. 2, pp. 119–157, 2015.
[18] N. E. Faouzi and L. A. Klein, “Data fusion for ITS: Techniques and research needs,” Transport. Res. Proc., vol. 15, pp. 495–512, 2016.
[19] Short-term Traffic Flow Prediction Based on Incremental Support Vector Regression
[20] C. Lamboley, J. C. Santucci, and M. Danech-Pajouh, "24 or 48-hour advance traffic forecast in the urban and periurban environment: The example of Paris," in Proc. 4th World Congress Intelligent Transport Systems, Mobility for Everyone, Berlin, 1997.
[21] F. Jin and S. Sun, “Neural network multitask learning for traffic flow forecasting,” in Proc. IEEE Int. Joint Conf. Neural Networks, Hong Kong, 2008, pp. 1897–1901.
[22] P. Næss and A. Strand, “Traffic forecasting at ’strategic’, ’tactical’ and ’operational’ level,” J. Crit. Realism, vol. 51, no. 2, pp. 41–48, 2015.
[23] K. Jha, N. Sinha, S. Arkatkar, and A. K. Sarkar, "A comparative study on the application of time series analysis for traffic forecasting in India: Prospects and limitations," Current Sci., vol. 110, no. 3, pp. 373–385, 2016.
[24] J. A. K. Suykens, J. Vandewalle, Least Square Support Vector Machine Classifiers, Neural Process Letter. 9 (1999): pp. 293-300
[25] J. H. Liu, J. P. Chen, S. Jiang, J. S. Cheng, Online LSSVM for function estimation and classification, Journal of University of Science and Technology Beijing. 10 (2003) 73-77
[26] C.H. Wu, C.C. Wei, M.H. Chang, et al. Travel time prediction with support vector regression. IEEE Transaction on Intelligent Transportation Systems, 2004, 5(12): pp. 276-281
[27] .Z.S. Yang, G.Y. Jiang. Theories and models of urban traffic guidance system based on high-order neural networks. Journal of Highway and Transportation Research and Development, 1998, 6: pp. 16-19
[28] C.Y. Zhang, G.H. Hu, T.Z. Xu. Traffic flow time series prediction based on LS-SVM. Journal of Yunnan University, 2004, 26: pp. 19-22
[29] Traffic Data Collection System Based on Floating Cars. Computer and Communications, 2006, 5(24): pp. 31-34