Classification and Prediction of Student Academic Performance using Machine Learning: A Review
Zeba Parveen1 , Mohatesham Pasha Quadri2
Section:Review Paper, Product Type: Journal Paper
Volume-7 ,
Issue-3 , Page no. 607-614, Mar-2019
CrossRef-DOI: https://doi.org/10.26438/ijcse/v7i3.607614
Online published on Mar 31, 2019
Copyright © Zeba Parveen, Mohatesham Pasha Quadri . This is an open access article distributed under the Creative Commons Attribution License, which permits unrestricted use, distribution, and reproduction in any medium, provided the original work is properly cited.
View this paper at Google Scholar | DPI Digital Library
How to Cite this Paper
- IEEE Citation
- MLA Citation
- APA Citation
- BibTex Citation
- RIS Citation
IEEE Citation
IEEE Style Citation: Zeba Parveen, Mohatesham Pasha Quadri, “Classification and Prediction of Student Academic Performance using Machine Learning: A Review,” International Journal of Computer Sciences and Engineering, Vol.7, Issue.3, pp.607-614, 2019.
MLA Citation
MLA Style Citation: Zeba Parveen, Mohatesham Pasha Quadri "Classification and Prediction of Student Academic Performance using Machine Learning: A Review." International Journal of Computer Sciences and Engineering 7.3 (2019): 607-614.
APA Citation
APA Style Citation: Zeba Parveen, Mohatesham Pasha Quadri, (2019). Classification and Prediction of Student Academic Performance using Machine Learning: A Review. International Journal of Computer Sciences and Engineering, 7(3), 607-614.
BibTex Citation
BibTex Style Citation:
@article{Parveen_2019,
author = {Zeba Parveen, Mohatesham Pasha Quadri},
title = {Classification and Prediction of Student Academic Performance using Machine Learning: A Review},
journal = {International Journal of Computer Sciences and Engineering},
issue_date = {3 2019},
volume = {7},
Issue = {3},
month = {3},
year = {2019},
issn = {2347-2693},
pages = {607-614},
url = {https://www.ijcseonline.org/full_paper_view.php?paper_id=3888},
doi = {https://doi.org/10.26438/ijcse/v7i3.607614}
publisher = {IJCSE, Indore, INDIA},
}
RIS Citation
RIS Style Citation:
TY - JOUR
DO = {https://doi.org/10.26438/ijcse/v7i3.607614}
UR - https://www.ijcseonline.org/full_paper_view.php?paper_id=3888
TI - Classification and Prediction of Student Academic Performance using Machine Learning: A Review
T2 - International Journal of Computer Sciences and Engineering
AU - Zeba Parveen, Mohatesham Pasha Quadri
PY - 2019
DA - 2019/03/31
PB - IJCSE, Indore, INDIA
SP - 607-614
IS - 3
VL - 7
SN - 2347-2693
ER -
![]() |
![]() |
![]() |
378 | 257 downloads | 123 downloads |
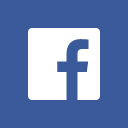
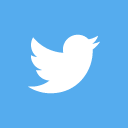
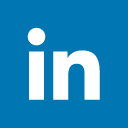
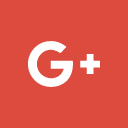
Abstract
Today, as education is very important for all human being, so it is necessary to analyze and improve the education system as technologies growing day by day, so use of latest technologies is very crucial to enhance the education system and academic performance of the student. Many researchers have been worked on predicting student performance and built predictive models to measure and predict students’ performance and found interesting results. This classification presents a review of works previously done by different authors on student performance by using different techniques. The aim of this work is to review the available study, to compare different models developed by different authors accordingly and to find out the best model from it. This study shows how different techniques used and produces result and which is best suitable technique. The various factors identified with the representation of machine learning algorithms based on methods and tools followed by their attributes and results respectively. This can help students, faculties, and institutions to increase the performance.
Key-Words / Index Term
Student Performance, Machine learning algorithms, Tools
References
[1] Amjad Abu Saa, “Educational Data Mining & Students’ Performance Prediction”, International Journal of Advanced Computer Science and Applications(IJACSA), Vol. 7, No. 5, 2016.
[2] Brijesh Kumar Bhardwaj and Saurabh Pal, “Data Mining: A prediction for performance improvement using classification”, International Journal of Computer Science and Information Security(IJCSIS), Vol. 9, No. 4, 2011.
[3] Augusto Sandoval, Carlos Gonzalez, Rosa Alarcon, Karim Pichara, Maximiliano Montenegro, “Centralized student performance prediction in large courses based on low-cost variables in an institutional context”, The Internet and Higher Education, Vol. 37, pp.76-89, 2018, DOI:10.1016/j.iheduc.2018.02.002.
[4] Ioannis E. Livieris, Konstantina Drakopoulou, Vassilis T. Tampakas, Tassos A. Mikropoulos, Panagiotis Pintelas, “Predicting Secondary School Students’ Performance Utilizing a Semi-supervised Learning Approach”, Journal of Educational Computing Research, 2018, DOI: 10.1177/0735633117752614.
[5] Evandro B. Costa, Baldoino Fonseca, Marcelo Almeida Santana, Fabrísia Ferreira de Araújo, Joilson Rego, “Evaluating the effectiveness of educational data mining techniques for early prediction of students` academic failure in introductory programming courses”, Computers in Human Behavior, Vol.73, pp. 247-256, 2017, doi:10.1016/j.chb.2017.01.047.
[6] Parneet Kaur, Manpreet Singh, Gurpreet Singh Josan, “Classification and prediction based data mining algorithms to predict slow learners in education sector”, 3rd International Conference on Recent Trends in Computing(ICRTC-2015), Vol.57, pp. 500-508, 2015.
[7] Shiwani Rana, Roopali Garg, “Slow Learner Prediction using Multi-Variate Naïve Bayes Classification Algorithm”, International Journal of Engineering and Technology Innovation, Vol . 7, No. 1, pp. 11-23, 2017.
[8] A. T. M. Shakil Ahamed, Navid Tanzeem Mahmood, Rashedur M Rahman,“An intelligent system to predict academic performance based on different factors during adolescence”, Journal of Information and Telecommunication, Vol.1, No.2, pp.155-175, 2017.
[9] Ahmed Mueen, Bassam Zafar , Umar Manzoor, “Modeling and Predicting Students’ Academic Performance Using Data Mining Techniques”, I.J. Modern Education and Computer Science, Vol.11, pp. 36-42, 2016.
[10] Fadhilah Ahmad, Nur Hafieza Ismail , Azwa Abdul Aziz, “The Prediction of Students’ Academic Performance Using Classification Data Mining Techniques”, Applied Mathematical Sciences, Vol. 9, No. 129, pp. 6415 - 6426, 2015.
[11] Jie Xu, Kyeong Ho Moon, Mihaela van der Schaar, “A Machine Learning Approach for Tracking and Predicting Student Performance in Degree Programs”, IEEE Journal of Selected Topics in Signal Processing, Vol.11, No.5, pp. 742-753, 2017.
[12] M. Mayilvaganan, D. Kalpanadevi, “Comparison of Classification Techniques for predicting the performance of Students Academic Environment”, International Conference on Communication and Network Technologies (ICCNT). IEEE, pp.113-118, 2014, doi:10.1109/CNT.2014.7062736.
[13] M. Durairaj, C. Vijitha, “Educational Data mining for Prediction of Student Performance Using Clustering Algorithms”, (IJCSIT) International Journal of Computer Science and Information Technologies, Vol. 5, pp.5987-5991, 2014.
[14] S.Taruna, Mrinal Pandey, “An Empirical Analysis of Classification Techniques for Predicting Academic Performance”, 2014 IEEE International Advance Computing Conference (IACC), pp. 523-528, 2014, doi: 10.1109/IAdCC.2014.6779379.
[15] Olugbenga Adejo, Thomas Connolly, “An Integrated System Framework For Predicting Students’ Academic Performance In Higher Educational Institutions”, International Journal of Computer Science & Information Technology (IJCSIT), Vol 9, No. 3, 2017.
[16] Maria Koutina, Katia Lida Kermanidis, “Predicting Postgraduate Students’ Performance Using Machine Learning Techniques”, Springer, Berlin, Heidelberg, Vol. 364, pp.159-168, 2011.
[17] Ashkan Sharabiani, Fazle Karim, Anooshiravan Sharabiani, Mariya Atanasov, Houshang Darabi, ”An Enhanced Bayesian Network Model for Prediction of Students’ Academic Performance in Engineering Programs”, IEEE Global Engineering Education Conference (EDUCON), pp.832-837, 2014, doi: 10.1109/EDUCON.2014.6826192.
[18] Paulo Cortez, Alice Silva, “Using Data Mining To Predict Secondary School Student Performance” , EUROSIS, 2008.
[19] Qasem A. et al., “Mining Student Data Using Decision Trees”, The International Arab Conference on Information Technology (ACIT’2006), Nov. 2006, Jordan.
[20] M. Ramaswami, R. Bhaskaran , “A CHAID Based Performance Prediction Model in Educational Data Mining”, International Journal of Computer Science Issues(IJCSI), Vol. 7, Issue 1, No. 1, January 2010.
[21] Ali Daud, Naif Radi Aljohani, Rabeeh Ayaz Abbasi, Miltiadis D. Lytras, Farhat Abbas, Jalal S. Alowibdi, “Predicting Student Performance using Advanced Learning Analytics”, 2017 International World Wide Web Conference Committee (IW3C2), pp. 415-421, 2017.
[22] Shaobo Huang, Ning Fang, “Predicting student academic performance in an engineering dynamics course: A comparison of four types of predictive mathematical models”, Computers & Education Vol.61, pp.133–145, 2013.
[23] S. Kotsiantis et al., “Predicting Students’ Performance In Distance Learning Using Machine Learning Techniques”, Applied Artificial Intelligence, Vol.18, No.5, pp.411-426, 2004.
[24] Reynold A. Rustia et al., “Predicting Student’s Board Examination Performance using Classification Algorithms”, in the proceedings of the 2018 7th International Conference on Software and Computer Applications (ICSCA), pp.233-237, 2018, doi: 10.1145/3185089.3185101
[25] J.F. Superby et al., “Determination of factors influencing the achievement of the first-year university students using data mining methods” 8th international conference on intelligent tutoring systems, Educational Data Mining Workshop, Jhongli, Taiwan, 2006.
[26] Havan Agrawal, Harshil Mavani , “Student Performance Prediction using Machine Learning”, International Journal of Engineering Research & Technology (IJERT), Vol. 4, Issue 03, March-2015.
[27] Amirah Mohamed Shahiri et al., “A Review on Predicting Student’s Performance using Data Mining Techniques”, The Third Information Systems International Conference, Procedia Computer Science, Vol.72, pp. 414-422, 2015.