Survey on Intrusion Detection System Based on Feature Classification and Selection
Madhavi Dhingra1
Section:Survey Paper, Product Type: Journal Paper
Volume-7 ,
Issue-3 , Page no. 399-403, Mar-2019
CrossRef-DOI: https://doi.org/10.26438/ijcse/v7i3.399403
Online published on Mar 31, 2019
Copyright © Madhavi Dhingra . This is an open access article distributed under the Creative Commons Attribution License, which permits unrestricted use, distribution, and reproduction in any medium, provided the original work is properly cited.
View this paper at Google Scholar | DPI Digital Library
How to Cite this Paper
- IEEE Citation
- MLA Citation
- APA Citation
- BibTex Citation
- RIS Citation
IEEE Style Citation: Madhavi Dhingra, “Survey on Intrusion Detection System Based on Feature Classification and Selection,” International Journal of Computer Sciences and Engineering, Vol.7, Issue.3, pp.399-403, 2019.
MLA Style Citation: Madhavi Dhingra "Survey on Intrusion Detection System Based on Feature Classification and Selection." International Journal of Computer Sciences and Engineering 7.3 (2019): 399-403.
APA Style Citation: Madhavi Dhingra, (2019). Survey on Intrusion Detection System Based on Feature Classification and Selection. International Journal of Computer Sciences and Engineering, 7(3), 399-403.
BibTex Style Citation:
@article{Dhingra_2019,
author = {Madhavi Dhingra},
title = {Survey on Intrusion Detection System Based on Feature Classification and Selection},
journal = {International Journal of Computer Sciences and Engineering},
issue_date = {3 2019},
volume = {7},
Issue = {3},
month = {3},
year = {2019},
issn = {2347-2693},
pages = {399-403},
url = {https://www.ijcseonline.org/full_paper_view.php?paper_id=3852},
doi = {https://doi.org/10.26438/ijcse/v7i3.399403}
publisher = {IJCSE, Indore, INDIA},
}
RIS Style Citation:
TY - JOUR
DO = {https://doi.org/10.26438/ijcse/v7i3.399403}
UR - https://www.ijcseonline.org/full_paper_view.php?paper_id=3852
TI - Survey on Intrusion Detection System Based on Feature Classification and Selection
T2 - International Journal of Computer Sciences and Engineering
AU - Madhavi Dhingra
PY - 2019
DA - 2019/03/31
PB - IJCSE, Indore, INDIA
SP - 399-403
IS - 3
VL - 7
SN - 2347-2693
ER -
![]() |
![]() |
![]() |
486 | 243 downloads | 142 downloads |
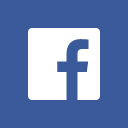
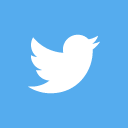
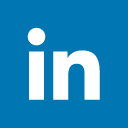
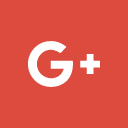
Abstract
Wireless networks are facing variety of attacks nowadays. To prevent from such attacks, a few Intrusion Detection frameworks are being created to distinguish and evacuate the attacks. Intrusion detection frameworks need to manage huge information having duplicate and excess features that require moderate training and testing processes leading to higher resource utilization and poor discovery rate. The performance of the Intrusion detection frameworks depend on the accuracy of the predicted attacks. Various performance parameters are to be considered for determining accuracy of a framework. The whole process is highly dependent on the network features and thus, Feature Classification is a vital issue in intrusion detection process. This paper covers the importance of feature selection, the common feature selection methods and various feature classification approaches that have been used in the field of Intrusion Detection System. The paper has also revised about the different researches that had taken place in the relevant field.
Key-Words / Index Term
Intrusion detection System, Feature Classification, Feature selection, Wireless Attacks
References
1. Snort Intrusion Detection System. 2006. Available online: http://www.snort.org, 10 October 2017.
2. Li, Y.; Wang, J.-L.; Tian, Z.-H.; Lu, T.-B.; Chen, Y. Building lightweight intrusion detection system using wrapper-based feature selection mechanisms. Comput. Secur., 28, pp. 466–475, 2009.
3. M. Dash, H. Liu, “Feature Selection for Classification,” Intelligent Data Analysis, Elsevier, pp. 131-156, 1997.
4. An Introduction to Feature Selection by Jason Brownlee on October 6, Machine Learning Process, 2014.
5. Hodo, E.; Bellekens, X.; Hamilton, A.; Dubouilh, P.; Iorkyase, E.; Tachtatzis, C.; Atkinson, R. Threat analysis of iot networks using artificial neural network intrusion detection system. In Proceedings of the IEEE International Symposium on Networks, Computers and Communications (ISNCC), Yasmine Hammamet, Tunisia, pp. 11–13 May 2016.
6. Hodo, E.; Bellekens, X.; Hamilton, A.; Tachtatzis, C.; Atkinson, R. Shallow and Deep Networks Intrusion Detection System: A Taxonomy and Survey; Cornell University Library: Ithaca, NY, USA, 2017.
7. Brifcani, A.; Issa, A. Intrusion detection and attack classifier based on three techniques: A comparative study. Eng. Technol. J., 29, pp. 368–412, 2011.
8. Roopadevi, E.; Bhuvaneswari, B.; Sahaana, B. Intrusion Detection using Support Vector Machine with Feature Reduction Techniques. Indian J. Sci. 23, pp. 148–156, 2016,.
9. Zhang, J.; Zulkernine, M. A hybrid network intrusion detection technique using random forests.In Proceedings of the IEEE First International Conference on Availability Reliability and Security (ARES’06), Vienna, Austria, 20–22 April 2006.
10. Farid, D.M.; Zhang, L.; Hossain, M.A.; Strachan, R. Hybrid decision tree and naïve Bayes classifiers for multi-class classification tasks. Expert Syst. Appl., 41, pp.1937–1946, 2014.
11. Koc, L.; Mazzuchi, T.A.; Sarkani, S. A network intrusion detection system based on a Hidden Naïve Bayes multiclass classifier. Expert Syst. Appl., 39, pp.13492–13500, 2012.
12. Farid, D.M.; Harbi, N.; Rahman, M.Z. Combining Naive Bayes and Decision Tree for Adaptive Intrusion Detection; Cornell University Library: Ithaca, NY, USA, arXiv preprint, 2010.
13. Fahad, A.; Zahir, T.; Ibrahim, K.; Ibrahim, H.; Hussein, A. Toward an efficient and scalable feature selection approach for internet traffic classification. Comput. Netw., 57, pp. 2040–2057, 2013.
14. Al-mamory, S.O.; Jassim, F.S. On the designing of two grains levels network intrusion detection system. Karbala Int. J. Mod. Sci., 1, pp. 15–25, 2015.
15. Yang, J.; Olafsson, S. Optimization-based feature selection with adaptive instance sampling. Comput. Oper. Res., 33, pp. 3088–3106, 2006.
16. Sánchez-Maroño, N.; Alonso-Betanzos, A.; Calvo-Estévez, R.M. A wrapper method for feature selection in multiple classes datasets. In International Work-Conference on Artificial Neural Networks; Springer: Berlin/Heidelberg, Germany, 2009.
17. Sani, R.A.; Ghasemi, A. Learning a new distance metric to improve an svm-clustering based intrusion detection system. In Proceedings of the IEEE International Symposium on Artificial Intelligence and Signal Processing (AISP), Mashhad, Iran, 3–5 March 2015.
18. Sarikaya, R.; Hinton, G.E.; Deoras, A. Application of deep belief networks for natural languageunderstanding. IEEE/ACM Trans. Audio Speech Lang. Process., 22, pp. 778–784, 2014.
19. Gisung Kim and Seungmin Lee, A Novel Hybrid Intrusion Detection Method Integrating Anomaly Detection With Misuse Detection, ELSEVIER, Expert Systems with Applications vol. 41 pp. 1690 – 1700, 2014.
20. Shi-Jinn Horng and Ming-Yang Su, “Novel Intrusion Detection System Based On Hierarchical Clustering and Support Vector Machines”, ELSEVIER, Expert Systems with Applications. pp. 38 306-313, 2011.
21. Mrutyunjaya Panda and Manas Ranjan Patra, “A Comparative Study Of Data Mining Algorithms For Network Intrusion Detection”, First International Conference on Emerging Trends in Engineering and Technology, pp 504-507, IEEE, 2008.
22. Juan Wang, Qiren Yang, Dasen Ren, “An intrusion detection algorithm based on decision tree technology”, In the Proc. of IEEE Asia-Pacific Conference on Information Processing, 2009.
23. Hong Kuan Sok et.al, “Using the ADTree for Feature Reduction through Knowledge Discovery” Instrumentation and Measurement Technology Conference (I2MTC), IEEE International ,pp 1040 – 1044, 2013.
24. Avallaee M, Bagheri E, Lu W, Ghorbani A. “A detailed analysis of the KDD CUP 99 data set”, 2009 IEEE Symposium on Computational intelligence for security and defense applications,,pp 1-6, 2009.
25. Bakar AA, Othman ZA, Hamdan AR, Yusof R, Ismail R: An Agent Based Rough Classifier for Data Mining. Eighth International Conference on Intelligent Systems Design and Applications , vol 1. IEEE Computer Society, Washington, pp.145-151, 2008.
26. Zhu X, Huang Z, Zhoul H: Design of a Multi-agent Based Intelligent Intrusion Detection System. IEEE International Symposium on Pervasive Computing and Applications. IEEE, Amsterdam, pp.290-295, 2006.
27. Fonk C-h, Parr GP, Morrow PJ: Security schemes for Mobile Agent based Network and System Management Framework. J. Networks Syst. Manag. Springer, 19: 232-256, 2011.
28. Mell P, Marks D, McLarnon M: A Denial of service resistant intrusion detection architecture. Comput Networks J Elsevier, Amsterdam, 2000.
29. Mar J, Yeh Y-C, Hsiao I-F: An ANFIS-IDS against Deauthentication DOS Attacks for a WLAN Taichung, 17–20 October 2010. IEEE, Amsterdam, pp. 548-553, 2010.
30. Linda O, Vollmer T, Manic M: Neural network based intrusion detection system for critical infrastructures. In Proceedings of IEEE International Joint Conference on Neural Networks, Georgia. IEEE, Amsterdam, pp.102-109, 2009.
31. Li Y, Wang R, Xu J, Yang G, Zhao B: Intrusion detection method based on fuzzy hidden Markov model. Sixth IEEE International Conference on Fuzzy Systems and Knowledge Discovery, vol 3. IEEE, Piscataway; pp. 470-474, 2009.
32. Tian J, Gu H: Anomaly detection combining one-class SVMs and Particle swarm optimization algorithms, Nonlinear Dynamics. Volume 61. Springer, Berlin; 2010:303-310.
33. Karmore, Preetee K.; Bodkhe, Sonali T, “A Survey on Intrusion in Ad Hoc Networks and its Detection Measures,” Proc. International Journal on Computer Science & Engineering, vol. 3, Issue 5, pp. 1896-1903, 2011.
34. Aakanksha Kori, Harsh Mathur,”A Performance Evaluation of Intrusion Detection system to get better detection rate using ANN Technique”, International Journal of Scientific Research in Computer Science, Engineering and Information Technology, Vol.2, Issue 5, pp.217-223, 2017.