Analyzing Cognitive Factor to Enhance Student Performance using Deep Learning Technique
J.Suganya 1 , T. Chakravarthy2
Section:Research Paper, Product Type: Journal Paper
Volume-7 ,
Issue-3 , Page no. 156-160, Mar-2019
CrossRef-DOI: https://doi.org/10.26438/ijcse/v7i3.156160
Online published on Mar 31, 2019
Copyright © J.Suganya, T. Chakravarthy . This is an open access article distributed under the Creative Commons Attribution License, which permits unrestricted use, distribution, and reproduction in any medium, provided the original work is properly cited.
View this paper at Google Scholar | DPI Digital Library
How to Cite this Paper
- IEEE Citation
- MLA Citation
- APA Citation
- BibTex Citation
- RIS Citation
IEEE Style Citation: J.Suganya, T. Chakravarthy, “Analyzing Cognitive Factor to Enhance Student Performance using Deep Learning Technique,” International Journal of Computer Sciences and Engineering, Vol.7, Issue.3, pp.156-160, 2019.
MLA Style Citation: J.Suganya, T. Chakravarthy "Analyzing Cognitive Factor to Enhance Student Performance using Deep Learning Technique." International Journal of Computer Sciences and Engineering 7.3 (2019): 156-160.
APA Style Citation: J.Suganya, T. Chakravarthy, (2019). Analyzing Cognitive Factor to Enhance Student Performance using Deep Learning Technique. International Journal of Computer Sciences and Engineering, 7(3), 156-160.
BibTex Style Citation:
@article{Chakravarthy_2019,
author = { J.Suganya, T. Chakravarthy},
title = {Analyzing Cognitive Factor to Enhance Student Performance using Deep Learning Technique},
journal = {International Journal of Computer Sciences and Engineering},
issue_date = {3 2019},
volume = {7},
Issue = {3},
month = {3},
year = {2019},
issn = {2347-2693},
pages = {156-160},
url = {https://www.ijcseonline.org/full_paper_view.php?paper_id=3812},
doi = {https://doi.org/10.26438/ijcse/v7i3.156160}
publisher = {IJCSE, Indore, INDIA},
}
RIS Style Citation:
TY - JOUR
DO = {https://doi.org/10.26438/ijcse/v7i3.156160}
UR - https://www.ijcseonline.org/full_paper_view.php?paper_id=3812
TI - Analyzing Cognitive Factor to Enhance Student Performance using Deep Learning Technique
T2 - International Journal of Computer Sciences and Engineering
AU - J.Suganya, T. Chakravarthy
PY - 2019
DA - 2019/03/31
PB - IJCSE, Indore, INDIA
SP - 156-160
IS - 3
VL - 7
SN - 2347-2693
ER -
![]() |
![]() |
![]() |
472 | 381 downloads | 232 downloads |
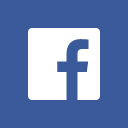
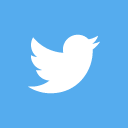
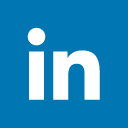
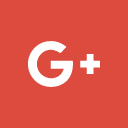
Abstract
Cognitive skills (CS) are the basic processing functions that enable to learn. These include attention, memory, auditory processing, visual processing, logic, and reasoning ability. It play a vital role in performance of any individual. Performance of students can be predicted by knowing the level of cognitive skill. The proposed method consists of three stages quantization, simulation and prediction. Finally, we analyzed the simulated data using deep learning algorithms. The learning algorithm Convolutional Neural Network (CNN) is used for our study. The proposed method is tested on the students` performance data sets in UCI repository. The results shows that CNN achieve higher accuracy than other the traditional approach.
Key-Words / Index Term
Cognitive skills, Study related characteristics, quantization, Deep learning algorithms, Convolutional Neural Network.
References
[1] Y. Wang, Y. Wang, S. Patel, and D. Patel, "A Layered reference model of the brain (LRMB)," IEEE Transactions on Systems, Man, and Cybernetics, Part C: Applications and Reviews, pp. 124-133,2006.
[2] L.-F. Rodriguez, F. Ramos, and Y. Wang, "Cognitive computational models of emotions," in Proc. 2011 10th IEEE InternationalConference on the Cognitive Informatics & Cognitive Computing, 2011.
[3] O. AlZoubi, I. Koprinska, and R. A. Calvo, "Classification of braincomputer interface data," presented at the 7th Australasian Data Mining Conference, Glenelg, Australia, 2008.
[4] E. A. Patall, R. R. Steingut, A. C. Vasquez, S. S. Trimble, K. A. Pituch, and J. L. Freeman, ``Daily autonomy supporting or thwarting and students` motivation and engagement in the high school science classroom,`` J. Educ.Psychol., vol. 110, no. 2, pp. 269_288, 2017.
[5] J. Härkönen, F. Bernardi, and D. Boertien, ``Family dynamics and childoutcomes: An overview of research and open questions,`` Eur. J. Population, vol. 33, no. 2, pp. 163_184, 2017.
[6] S. Ahmad, K. Li, H. A. I. Eddine, and M. I. Khan, ``A biologically inspired cognitive skills measurement approach,`` Biol. Inspired Cogn. Archit.,vol. 24, pp. 35_46, Apr. 2018.
Nkambou, R., J. Bourdeau, et al. (2010). [7] Koedinger, K. and E. A. Mclaughlin (2010). Advances in Intelligent Tutoring Systems.
[8]Seeing language learning inside the math: Cognitive analysis yields transfer. the 32nd Annual Conference of the Cognitive Science Society. Austin, TX: 471- 476.
[9] Anderson, J. R. and B. J. Reiser (1985). "The LISP Tutor." Byte 10: 159-175.
[10] J. Xu, K. H. Moon, and M. van der Schaar, ``A machine learning approach for tracking and predicting student performance in degree programs,``
IEEE J. Sel. Topics Signal Process. vol. 11, no. 5, pp. 742_753, Aug. 2017.
[11] M. Pojon, ``Using machine learning to predict student performance,`` M.S. thesis, Fac. Natural Sci. Softw. Develop. Univ. Tampere, Tampere, Finland, 2017.
[12] Z. Iqbal, J. Qadir, A. N. Mian, and F. Kamiran. (2017). ``Machine learning based student grade prediction: A case study.`` [Online]. Available: https://arxiv.org/abs/1708.08744
[13] A. S. Lillard, M. D. Lerner, E. J. Hopkins, R. A. Dore, E. D. Smith, and C. M. Palmquist, ``The impact of pretend play on children`s development:
A review of the evidence,`` Psychol. Bull., vol. 139, no. 1, pp. 1_34, 2013.
[14] B. Y. Ohye et al., ``Three-generation model: A family systems framework for the assessment and treatment of veterans with posttraumatic stress disorder and related conditions,`` Prof. Psychol., Res. Pract., vol. 46, no. 2,
pp. 97_106, 2015.
[15] G. M. Fosco, M. J. Van Ryzin, A. M. Connell, and E. A. Stormshak, ``Preventing adolescent depression with the family check-up: Examining family con_ict as a mechanism of change,`` J. Family Psychol., vol. 30, no. 1, pp. 82_92, 2016.
[16] I. E. Livieris, K. Drakopoulou, and P. Pintelas, ``Predicting students` performance using arti_cial neural networks,`` in Proc. 8th PanHellenicConf. Int. Participation Inf. Commun. Technol. Edu., 2012, pp. 321_328.
[17] D. Dorner and J. Gerdes, "Motivation, emotion, intelligence," in Proc. the International Conference on Systems and Informatics, Yantai, 2012.
[18]Weka 3:Data Mining Software in Java, Machine Learning Group at the University of Waikato, Official Web: http://www.cs.waikato.ac.nz/ml/weka/index.html, accessed on 26th March 2016.