A Survey on Machine Learning and Statistical Methods for Bankruptcy Prediction
C. Punitha Devi1 , T. Vigneswari2 , C. Nancy3 , E. Priyanka4 , R. Yamuna5
Section:Survey Paper, Product Type: Journal Paper
Volume-7 ,
Issue-3 , Page no. 104-111, Mar-2019
CrossRef-DOI: https://doi.org/10.26438/ijcse/v7i3.104111
Online published on Mar 31, 2019
Copyright © C. Punitha Devi, T. Vigneswari, C. Nancy, E. Priyanka, R. Yamuna . This is an open access article distributed under the Creative Commons Attribution License, which permits unrestricted use, distribution, and reproduction in any medium, provided the original work is properly cited.
View this paper at Google Scholar | DPI Digital Library
How to Cite this Paper
- IEEE Citation
- MLA Citation
- APA Citation
- BibTex Citation
- RIS Citation
IEEE Citation
IEEE Style Citation: C. Punitha Devi, T. Vigneswari, C. Nancy, E. Priyanka, R. Yamuna, “A Survey on Machine Learning and Statistical Methods for Bankruptcy Prediction,” International Journal of Computer Sciences and Engineering, Vol.7, Issue.3, pp.104-111, 2019.
MLA Citation
MLA Style Citation: C. Punitha Devi, T. Vigneswari, C. Nancy, E. Priyanka, R. Yamuna "A Survey on Machine Learning and Statistical Methods for Bankruptcy Prediction." International Journal of Computer Sciences and Engineering 7.3 (2019): 104-111.
APA Citation
APA Style Citation: C. Punitha Devi, T. Vigneswari, C. Nancy, E. Priyanka, R. Yamuna, (2019). A Survey on Machine Learning and Statistical Methods for Bankruptcy Prediction. International Journal of Computer Sciences and Engineering, 7(3), 104-111.
BibTex Citation
BibTex Style Citation:
@article{Devi_2019,
author = {C. Punitha Devi, T. Vigneswari, C. Nancy, E. Priyanka, R. Yamuna},
title = {A Survey on Machine Learning and Statistical Methods for Bankruptcy Prediction},
journal = {International Journal of Computer Sciences and Engineering},
issue_date = {3 2019},
volume = {7},
Issue = {3},
month = {3},
year = {2019},
issn = {2347-2693},
pages = {104-111},
url = {https://www.ijcseonline.org/full_paper_view.php?paper_id=3805},
doi = {https://doi.org/10.26438/ijcse/v7i3.104111}
publisher = {IJCSE, Indore, INDIA},
}
RIS Citation
RIS Style Citation:
TY - JOUR
DO = {https://doi.org/10.26438/ijcse/v7i3.104111}
UR - https://www.ijcseonline.org/full_paper_view.php?paper_id=3805
TI - A Survey on Machine Learning and Statistical Methods for Bankruptcy Prediction
T2 - International Journal of Computer Sciences and Engineering
AU - C. Punitha Devi, T. Vigneswari, C. Nancy, E. Priyanka, R. Yamuna
PY - 2019
DA - 2019/03/31
PB - IJCSE, Indore, INDIA
SP - 104-111
IS - 3
VL - 7
SN - 2347-2693
ER -
![]() |
![]() |
![]() |
480 | 437 downloads | 225 downloads |
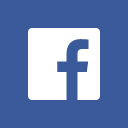
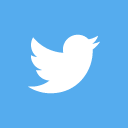
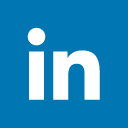
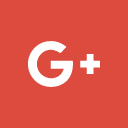
Abstract
To validate the management or organization`s creditworthiness, bankruptcy prediction model (BPM) is significantly important for financial firms. The socio-economic effects can be devastated if there exist a lack in predicting bankruptcy precisely. To anticipate this, it is important to provide financial decision makers with bankruptcy prediction in a efficient manner. To deal with this bankruptcy prediction problems, this paper projects a inclusive review depending on different machine learning and statistical methods. The methods of machine learning includes decision trees, artificial neural networks (ANN) and support vector machines (SVM) whereas the statistical methods such as logistic regression (LR), multivariate discriminant analysis (MDA) and linear discriminant analysis (LDA) are used. To manage huge data sets without degrading performance by means of prediction, conventional statistical methods were employed. For small data sets, the machine learning methods offers better accuracy in terms of predictions when compared with the conventional statistical methods. Depending on the respective methods advantages and drawbacks, this paper examines a comparative study of different methods. To enhance the accuracy for massive data sets, particle swarm optimization (PSO) and genetic algorithm (GA) are the optimization methods that were combined for bankruptcy prediction
Key-Words / Index Term
Bankruptcy, ANN; SVM; Machine learning
References
[1] Z. S. Hua et al., "Predicting corporate financial distress based on integration of support vector machine and logistic regression," Expert Systems with Applications, vol. 33, no. 2, pp. 434-440, 2007.
[2] G. Kumar and S. Roy, “Development of hybrid boosting technique for bankruptcy prediction,” in Proc. International Conference on Information Technology, 2016, pp. 248-253.
[3] L. Becchetti, and J. Sierra, “Bankruptcy risk and productive efficiency in manufacturing firms,” Journal of Banking & Finance, vol. 27, no. 11, pp. 2099-2120, November 2003.
[4] W.-S. Chen and Y.-K. Du, “Using neural networks and data mining techniques for the financial distress prediction model," Expert Systems with Applications, vol. 36, no. 2, pp. 4075-4086, 2009.
[5] M. A. Aziz and H. A. Dar, “Predicting corporate bankruptcy: Where we stand?” Corporate Governance: The International Journal of Business in Society, vol. 6, no.1, pp. 18-33, 2006.
[6] W. Y. Lin, Y. H. Hu, and C. F. Tsai, “Machine learning in financial crisis prediction: A survey,” IEEE Transactions on Systems, Man, and Cybernetics, Part C (Applications and Reviews), vol. 42, no. 4, pp. 421-436, November 2011.
[7] P. R. Kumar and V. Ravi, “Bankruptcy prediction in banks and firms via statistical and intelligent techniques — A review,” European Journal of Operational Research, vol. 180, no. 1, pp. 1-28, July 2007.
[8] M. Y. Chen, “Bankruptcy prediction in firms with statistical and intelligent techniques and a comparison of evolutionary computation approaches,” Computers & Mathematics with Applications, vol. 62, no. 12, pp. 4514-4524, December 2011.
[9] E. Kirkos, C. Spathis, and Y. Manolopoulos, “Data mining techniques for the detection of fraudulent financial statements,” Expert Systems with Applications, vol. 32, no. 4, pp. 995-1003, May 2007.
[10] S. Lee and W. S. Choi, “A multi-industry bankruptcy prediction model using back-propagation neural network and multivariate discriminant analysis,” Expert Systems with Applications, vol. 40, no. 8, pp. 2941-2946, June 2013.