Handwritten Signature Verification Using Convolutional Neural Networks
D.V.S. Abhigna1 , D. Srujana2 , D. Hema Varshini3 , G. Niharika4 , A. Vishnu Vardhan5
Section:Research Paper, Product Type: Journal Paper
Volume-7 ,
Issue-3 , Page no. 55-59, Mar-2019
CrossRef-DOI: https://doi.org/10.26438/ijcse/v7i3.5559
Online published on Mar 31, 2019
Copyright © D.V.S. Abhigna, D. Srujana, D. Hema Varshini, G. Niharika, A. Vishnu Vardhan . This is an open access article distributed under the Creative Commons Attribution License, which permits unrestricted use, distribution, and reproduction in any medium, provided the original work is properly cited.
View this paper at Google Scholar | DPI Digital Library
How to Cite this Paper
- IEEE Citation
- MLA Citation
- APA Citation
- BibTex Citation
- RIS Citation
IEEE Style Citation: D.V.S. Abhigna, D. Srujana, D. Hema Varshini, G. Niharika, A. Vishnu Vardhan, “Handwritten Signature Verification Using Convolutional Neural Networks,” International Journal of Computer Sciences and Engineering, Vol.7, Issue.3, pp.55-59, 2019.
MLA Style Citation: D.V.S. Abhigna, D. Srujana, D. Hema Varshini, G. Niharika, A. Vishnu Vardhan "Handwritten Signature Verification Using Convolutional Neural Networks." International Journal of Computer Sciences and Engineering 7.3 (2019): 55-59.
APA Style Citation: D.V.S. Abhigna, D. Srujana, D. Hema Varshini, G. Niharika, A. Vishnu Vardhan, (2019). Handwritten Signature Verification Using Convolutional Neural Networks. International Journal of Computer Sciences and Engineering, 7(3), 55-59.
BibTex Style Citation:
@article{Abhigna_2019,
author = {D.V.S. Abhigna, D. Srujana, D. Hema Varshini, G. Niharika, A. Vishnu Vardhan},
title = {Handwritten Signature Verification Using Convolutional Neural Networks},
journal = {International Journal of Computer Sciences and Engineering},
issue_date = {3 2019},
volume = {7},
Issue = {3},
month = {3},
year = {2019},
issn = {2347-2693},
pages = {55-59},
url = {https://www.ijcseonline.org/full_paper_view.php?paper_id=3796},
doi = {https://doi.org/10.26438/ijcse/v7i3.5559}
publisher = {IJCSE, Indore, INDIA},
}
RIS Style Citation:
TY - JOUR
DO = {https://doi.org/10.26438/ijcse/v7i3.5559}
UR - https://www.ijcseonline.org/full_paper_view.php?paper_id=3796
TI - Handwritten Signature Verification Using Convolutional Neural Networks
T2 - International Journal of Computer Sciences and Engineering
AU - D.V.S. Abhigna, D. Srujana, D. Hema Varshini, G. Niharika, A. Vishnu Vardhan
PY - 2019
DA - 2019/03/31
PB - IJCSE, Indore, INDIA
SP - 55-59
IS - 3
VL - 7
SN - 2347-2693
ER -
![]() |
![]() |
![]() |
668 | 630 downloads | 264 downloads |
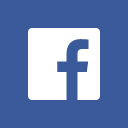
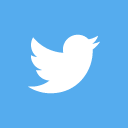
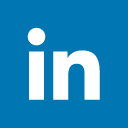
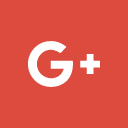
Abstract
The area of Handwritten Signature Verification has been extensively investigated in the most recent decades yet remains an open research issue in image recognition. The target of signature verification is to segregate if the given signature is genuine (delivered by the guaranteed individual), or a fraud (created by an impostor). These curves describe the general shape of the signature and ignore the slight details that vary from a genuine signature to another. The verification is based on the comparison of characteristic curves by dynamic programming, which is a very powerful method for curves comparison. Much progression has been proposed in the writing in the last 5-10 years, most remarkably the use of Convolutional Neural Networks (CNN) strategies to take in highlight portrayals from signature pictures. Convolutional Neural Networks are utilized to order pictures and perform object recognition in the images. In this paper, we present how the issue has been taken care of in the previous couple of decades, discuss the ongoing progressions in the field, and the potential headings for future research.
Key-Words / Index Term
Forgery, Handwritten Recognition, Image Processing, Neural Networks
References
[1] S. Ghandali and M. Ebrahimi Moghaddam, Off-Line Persian Signature Identification and Verification based on Image Registration and Fusion, In: Journal of Multimedia, volume 4, 2009, pages: 137-144
[2] J. R. Justino , F Bortolozzi and R. sabourin , Off-line signature verification Using HMM for random, simple and skilled forgeries,ICDAR 2001, International Conference on document Analysis and Recognition, vol. 1 pp. 105-110. 2001.
[3] Larkins, R. Mayo, M., “Adaptive Feature Thresholding for Off-Line Signature Verification”, In: Image and vision computing NewZealand, 2008, pages: 1-6.
[4] Kovari, B. Kertesz, Z. and Major, a., Off-Line Signature Verification Based on Feature Matching: In: Intelligent Engineering Systems,2007, pages 93-97.
[5] B. Fang, C.H Leung, Y.Y. Tang, K.W. Tse, P.C.K. Kwok, and Y.K. Wong, Off-line signature verification by the tracking of feature and stroke positions, Pattern Recognition, vol. 36, pp. 91-101, 2003.